Children Tracking and Tele Health Care Monitoring System in Signal Processing
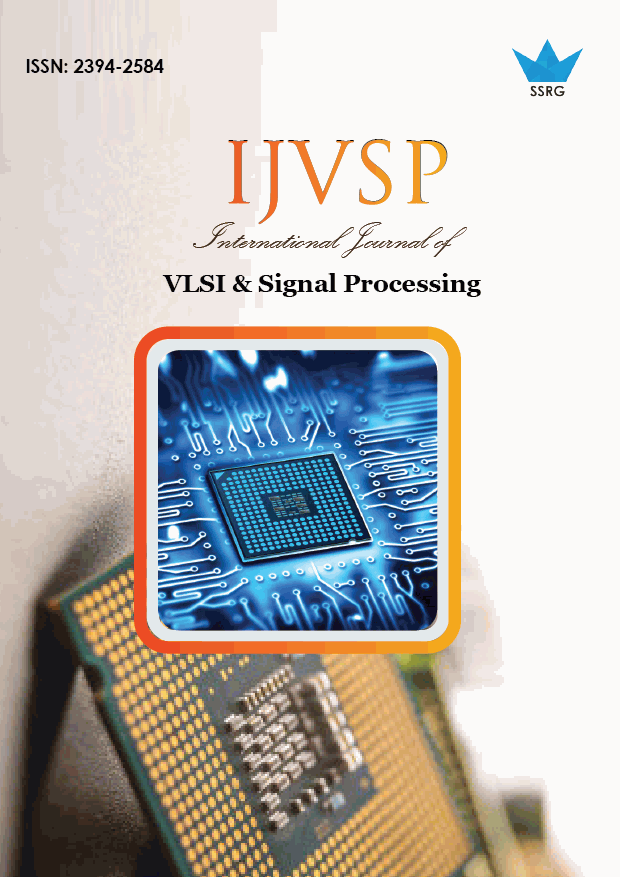
International Journal of VLSI & Signal Processing |
© 2014 by SSRG - IJVSP Journal |
Volume 1 Issue 1 |
Year of Publication : 2014 |
Authors : S.Kalaiyarasan and Dr.N.Sivakumar |
How to Cite?
S.Kalaiyarasan and Dr.N.Sivakumar, "Children Tracking and Tele Health Care Monitoring System in Signal Processing," SSRG International Journal of VLSI & Signal Processing, vol. 1, no. 1, pp. 4-6, 2014. Crossref, https://doi.org/10.14445/23942584/IJVSP-V1I1P102
Abstract:
This paper discuss about the latest technology evolved in the signal processing. This paper propose a new technique to monitor the school going childrens and it is used to prevent them from kidnapping and from other sort of injuries. It will also extented the facility for the hospital management system by implementing the telemonitoring system in healthcare. The new sensor technology were entered into the field by rapid developement in mobile technology and telecommunication.
Keywords:
wearable sensor, SMS, GSM, pulse rate, heartbeat rate.
References:
[1] Chandola V., Banerjee A., Kumar V. Anomaly detection for discrete sequences: A survey. IEEE Trans. Knowl. Data Eng. 2012; 24:823–839.
[2] Chatterjee S., Dutta K., Xie H.Q., Byun J., Pottathil A., Moore M. Persuasive and Pervasive Sensing: A New Frontier to Monitor, Track and Assist Older Adults Suffering from Type-2 Diabetes. Proceedings of the 46th Hawaii International Conference on System Sciences; Grand Wailea, Maui, HI, USA. 7–10 January 2013; pp. 2636–2645.
[3] Zhu Y. Automatic detection of anomalies in blood glucose using a machine learning approach. J. Commun. Netw. 2011;13:125–131.
[4] Mukherjee A., Pal A., Misra P. Data Analytics in Ubiquitous Sensor-Based Health Information Systems. Proceedings of the 2012 6th International Conference on Next Generation Mobile Applications, Services and Technologies; Paris, France. 12–14 September 2012; pp. 193–198.
[5] Gaura E., Kemp J., Brusey J. Leveraging knowledge from physiological data: On-body heat stress risk prediction with sensor networks. IEEE Trans. Biomed. Circuits Syst. 2013 in press.
[6] Lee K.H., Kung S.Y., Verma N. Low-energy formulations of support vector machine kernel functions for biomedical sensor applications. J. Signal Process. Syst. 2012;69:339–349.
[7] Chandola V., Banerjee A., Kumar V. Anomaly detection: A survey. ACM Comput. Surv. 2009;41:15:1–15:58.
[8] Gialelis J., Chondros P., Karadimas D., Dima S., Serpanos D. Identifying Chronic Disease Complications Utilizing State of the Art Data Fusion Methodologies and Signal Processing Algorithms. In: Nikita K.S., Lin J.C., Fotiadis D.I., Arredondo Waldmeyer M.T., editors. Wireless Mobile Communication and Healthcare. Volume 83. Springer; Berlin, Germany: 2012. pp. 256–263.
[9] Thakker B., Vyas A.L. Support vector machine for abnormal pulse classification. Int. J. Comput. Appl. 2011; 22:13–19.
[10] Huang G., Zhang Y., Cao J., Steyn M., Taraporewalla K. Online mining abnormal period patterns from multiple medical sensor data streams. World Wide Web. 2013 doi: 10.1007/s11280-013-0203-y.
[11] Clifton L., Clifton D.A., Pimentel M.A.F., Watkinson P.J., Tarassenko L. Gaussian processes for personalized e-health monitoring with wearable sensors. IEEE Trans. Biomed. Eng. 2013;60:193–197.
[12] Banaee H., Ahmed M.U., Loutfi A. A Framework for Automatic Text Generation of Trends in Physiological Time Series Data. Proceedings of the IEEE International Conference on System, Man, and Cybernetics; Manchester, UK. 13–16 October 2013; pp. 3876–3881.