Sugarcane Classification Using Spectral Signature and Object-Based Image Analysis (OBIA) in LiDAR Data Sets
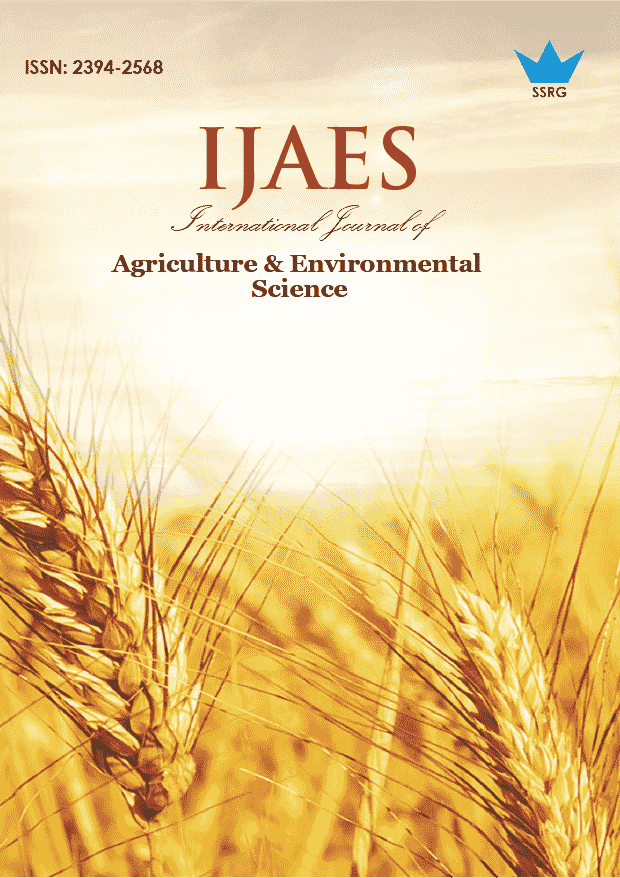
International Journal of Agriculture & Environmental Science |
© 2019 by SSRG - IJAES Journal |
Volume 6 Issue 4 |
Year of Publication : 2019 |
Authors : Marife Kung Villareal, Alejandro Fernandez Tongco |
How to Cite?
Marife Kung Villareal, Alejandro Fernandez Tongco, "Sugarcane Classification Using Spectral Signature and Object-Based Image Analysis (OBIA) in LiDAR Data Sets," SSRG International Journal of Agriculture & Environmental Science, vol. 6, no. 4, pp. 9-16, 2019. Crossref, https://doi.org/10.14445/23942568/IJAES-V6I4P103
Abstract:
The aim of this study is to classify and identify the different stages of sugarcane by combining the spectral signature and Object-based Image Analysis (OBIA) with Light Detection and Ranging (LiDAR) data sets. Preliminary field spectral measurement is carried out to determine the growth stages of sugarcane. Spectral measurement is done using the Ocean Optics USB4000 VIS NIR a miniature spectrometer pre-configured for general visible and near-IR measurements. It covers a wide wavelength range, from 350 to 1000 nm field spectrometer device, and a 20-meter fiber optic cable and white reference panel. The spectral signatures of sugarcane are evaluated within the spectrum range of 400 to 700 nanometer to fit the spectral range of the RGB bands of the orthoimages. A Normalized Digital Surface Model (nDSM) was created using the LiDAR data. The nDSM was paired with Orthoimages and segmented for feature extraction in OBIA. A rule-set was developed in eCognition software to classify the sugarcane growth stages. A machine learning algorithm called Support Vector Machine (SVM) was used to classify sugarcane growth stages in the image. SVM model was constructed using the nDSM. High overall accuracies are obtained for the growth stages of sugarcane. Establishment stage is 80%, Tillering stage is 92% and Yield Formation/Ripening stage is 83%. With the remotely sensed data like LiDAR and the specific band ratios derived from the spectral signature of sugarcane, proves to be reliable features in classifying the growth stages of sugarcane.
Keywords:
spectral signature, sugarcane growth stages
References:
[1] R.N. Sahoo, S. S. Ray, K.R. Manjunath. Hyperspectral remote sensing of agriculture. Current Science. Vol. 108. Issue 3, pp. 848-859, 2015
[2] C. Ndegwa, M. Boitt, P. Pellika, Using Hyperspectral Data to Identify Crops in a Cultivated Agricultural Landscape - A Case Study of Taita Hills, Kenya, Journal of Earth Science & Climatic Change. Vol. 5, Issue 9. 2014. doi:10.4172/2157-7617.1000232
[3] L. J. Hui, Z. Chen, S. Pei, X. Hu Zhang, Monitoring Sugarcane Growth Using ENVISAT ASAR Data, IEEE Transactions on Geoscience and Remote Sensing, VOL. 47, NO. 8. 2009.
[4] S. Padma, S. Sanjeevi, Jeffries Matusita, Based mixed-measure for improved spectral matching in hyperspectral image analysis. Int. J. Appl. Earth Obs. Geoinf. 2014, 32, 138–151.
[5] Yuanyuan Chen, Quanfang Wang, Yanlong Wang , Si-Bo Duan, Miaozhong Xu, and Zhao-Liang Li. A Spectral Signature Shape-Based Algorithm for Landsat Image Classification. International Journal o f Geo-Information.Vol.5, Issue 9. 2016. doi:10.3390/ijgi5090154
[6] C.J. Gers, Applications of remote sensing in sugarcane agriculture at Umflozi, SouthAfrica. MSc thesis, School of Environmental Sciences, University of KwaZulu-Natal,Pietermaritzburg, South Africa. 2004
[7] C. Fortesand J.A.M. Dematte, Discrimination of sugarcane varieties using Landsat7 ETM+ spectral data. International Journal of Remote Sensing, 27, pp. 395–1412., 2006
[8] Galva˜ O, L.S., Formaggio, A.R. and Tisot, D.A., Discrimination of sugarcanevarieties in Southeastern Brazil with EO-1 Hyperion data. Remote Sensing of Environment, 94, pp. 523–534., 2005
[9] L.S.Galva˜ O, A.R. Formaggio and D.A. Tisot, The influence of spectral resolutionon discriminating Brazilian sugarcane varieties. International Journal of RemoteSensing, 24, pp. 769–777., 2006
[10] A. Apan, A. Held, S. Phinn, and J. Markley, 2004a, Detection of sugarcane „orange rust‟disease using EO-1 Hyperion hyperspectal imagery. International Journal of RemoteSensing, 25, pp. 489–498.
[11] R.M. Johnson, R.P. Viator, C.J. Veremis, E.P. Richard, and P. Zimba, 2005,Discrimination of sugarcane varieties with hyperspectral reflectance measurements and plant pigment analysis. Journal of the American Society of Sugar CaneTechnologists, 25, pp. 111–112
[12] C.-I Chang, Hyperspectral Imaging: Techniques for Spectral Detection and Classification, Kluwer/Plenum Acad. Publ., 2003.
[13] M.T., Blaschke, Object based image analysis for remote sensing, ISPRS J. Photogram. RemoteSens., Vol. 65, pp. 2 – 16, 2010.
[14] B. Dixon, N. Candade, Multispectral landuse classification using neural networks and support vector machines: one or the other, or both? International Journal ofRemote Sensing, 29, pp. 1185-1206, 2007.
[15] M. Hansen, R. Dubayah, R.Defries, Classification trees: an alternative to traditional land cover classifiers. International Journal of Remote Sensing, 17, pp. 1075-1081, 1996.
[16] G.M. Foody, Supervised image classification by MLP and RBF neural networks with and without an exhaustively defined set of classes, Int. J. Remote Sens., 25, 3091–3104., 2004
[17] G.I.J. Mountrakis and C. Ogole, Support vector machines in remote sensing: A review. ISPRS Journal of Photogrammetry and Remote Sensing, 66, pp. 247-259, 2011.
[18] eCognition reference book
http://haralick.org/journals/TexturalFeatures.pdf
http://murphylab.web.cmu.edu/publications/boland/boland_node26.html