Building a Predictive Model for Tobacco Production in Syria Using the Multi-Layer Neural Network (Perceptron MLP)
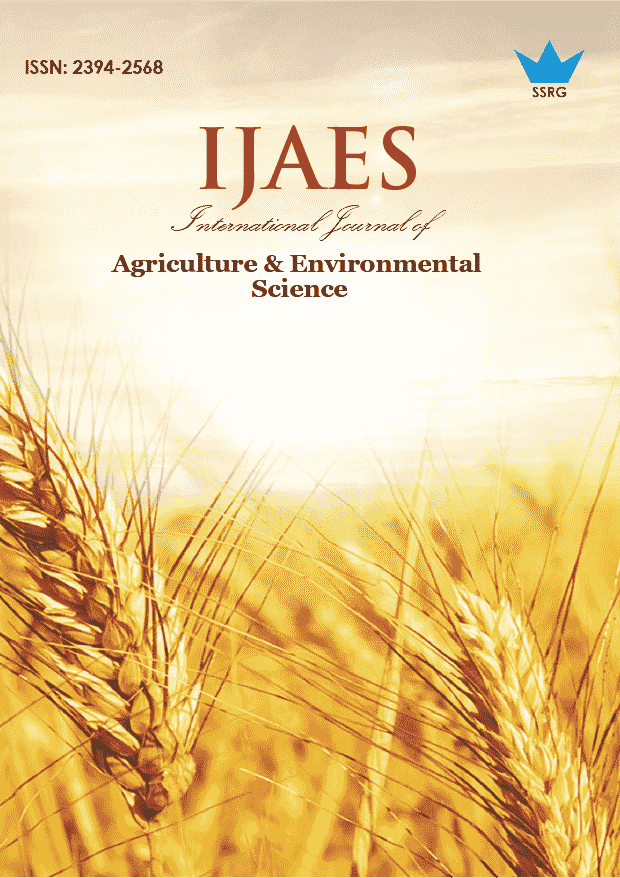
International Journal of Agriculture & Environmental Science |
© 2020 by SSRG - IJAES Journal |
Volume 7 Issue 4 |
Year of Publication : 2020 |
Authors : Majd Namaa, Ghassan Yacoub, Ayman Achouch |
How to Cite?
Majd Namaa, Ghassan Yacoub, Ayman Achouch, "Building a Predictive Model for Tobacco Production in Syria Using the Multi-Layer Neural Network (Perceptron MLP)," SSRG International Journal of Agriculture & Environmental Science, vol. 7, no. 4, pp. 69-77, 2020. Crossref, https://doi.org/10.14445/23942568/IJAES-V7I4P110
Abstract:
The research aimed to build a predictive model for tobacco production at the level of the Syrian Arab Republic using neural networks technology, based on the statistics of the World Food and Agriculture Organization (FAO) for the period between (1975- 2017), where the relationship between independent variables (year of production, area planted with tobacco was studied). Yield per hectare of tobacco crop) and its effect on the amount of production of the crop as a dependent variable based on the descriptive approach and the analytical statistical approach and building a multilayer neural network model (Perceptron MLP) and training it with a back propagation algorithm using statistical software (SPSS20).
The neural network consisted of three layers (the input layer, the processing layer or the hidden layer, in addition to the output layer), where the input layer consisted of three units (the number of independent variables), while the output and hidden layers consisted of one unit. The input layer received the information from the time series data, the hidden layer processed the information and produced the output layer. The values from the input layer entering the hidden node are multiplied by weights, which is the set of predetermined numbers and products are then added to produce a single number. She passed this number as a parameter to a nonlinear mathematical function (activation function) using the Hyperbolic function as
an activation function in the hidden layer, and the Identity function was used in the output layer.
34-year data was used in the training of the chosen neural network, and 9 years in the process of network testing and comparison of network outputs with actual outputs.
The model showed high accuracy in prediction, as the total of error squares (0.18) in the training phase with a accuracy of (99.82)% and in the testing phase (0.17) with an accuracy of (99.83)%.
The neural network showed the relative importance of the independent variables in the impact on agricultural production of the tobacco crop, where
yield per hectare occupied the first rank in the influence according to the predictive model by (100)%, while the cultivated area affected production by (77.2%), while the productive year was its effect Little by (4.7)%.
Keywords:
Production, Tobacco, Syria, Multi-layer neural network
References:
[1] Tobacco balance sheet, 2017. The Ministry of Industry. Official Statistics Office. Syria
[2] John Wiley, Sons (2008). “Introduction to Time Series Analysis”
[3] Ali Abdul-Azim Abdul-Karim (2013), "Using Artificial Neural Networks to Predict a Multidimensional Macroeconomic Model in Iraq during the Period (1996-2007)" (Gulf Economic Journal, Issue (24).
[4] Ali AwadallahTayfour (2010), “Introduction to Artificial Neural Networks” (College of Engineering, Sudan University of Science and Technology).
[5] Yochanan Shachnurove , “ Utilizing Artificial Network to predict Stock Markets “, the city collage of the city University of New York & The University of pennsylvania , September 2000 p.1
[6] Leonldas Anastasakis , &Neil Mort, “Network-based of the USD / GBP exchange rate the Utilization of data compressionTechniques For input imension Reduction “ , University of Sheffield , SI 3JD , U.K , p.1
[7] www.FAO STATISCS.com
[8] Al-Kaabi Haider Hamid, Ali Darb Al-Hayali (2015), "Predicting the Food Gap for Wheat and Rice Crops in Iraq Using the Artificial Neural Networks Method ANN for the period 2014-2022", (Al-Muthanna Journal of Agricultural Sciences, Iraq, Volume 4, page 1-21
[9] Coryn AL-Baler-Jones Daviad J.C Mackag “ A Recurrent Neural Network for Modeling Dynamical “, Department of Materials Science &Metallurg , University of Cambridge, Pembroke S.T,Cambridge ,CB23 QZ,England , 1998,p.Forecasting “, Technical University of Denmark.
[10] K.WAR Wick ; QMZHU & ZMA ,” A hyperstable neural network for the modeling and control of nonlinear system “ . Sadhand ,vol.25 , part 2 , April 2000 , p169 – 180 , printed in India
[11] Khaled Abdel-Wahid Mohamed Ahmed (2001), "Application of artificial neuronal networks to predict the rate of self-sufficiency of red meat" (Journal of Agricultural Sciences, Faculty of Agriculture, Mansoura University, Egypt)
[12] Gurpreet Kaur, Gurmeet Kaur” Various Types of Functional Link Artificial Neural Network Based Nonlinear Equalizers used in CO-OFDM System for Nonlinearities Mitigation” SSRG International Journal of Electronics and Communication Engineering(SSRG - IJECE)–Volume5 Issue2 Feb 2018.