Estimating the Effect of Biennial Olive Bearing to Forecast Syrian Olive- Oil Production by Using Box-Jenkins (ARIMA) Methodology
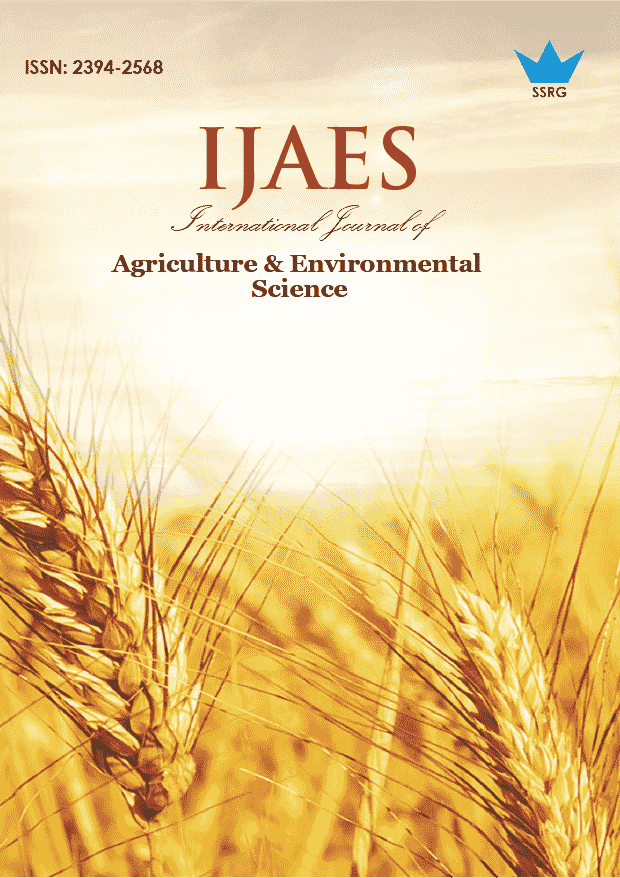
International Journal of Agriculture & Environmental Science |
© 2020 by SSRG - IJAES Journal |
Volume 7 Issue 5 |
Year of Publication : 2020 |
Authors : Wael Habib, Mohammad Ghoush |
How to Cite?
Wael Habib, Mohammad Ghoush, "Estimating the Effect of Biennial Olive Bearing to Forecast Syrian Olive- Oil Production by Using Box-Jenkins (ARIMA) Methodology," SSRG International Journal of Agriculture & Environmental Science, vol. 7, no. 5, pp. 21-30, 2020. Crossref, https://doi.org/10.14445/23942568/IJAES-V7I5P104
Abstract:
This study aimed to analyze the time series of olive-oil production in Syria and thus estimate the effects of biennial/alternate fruit-bearing phenomena
and determine the appropriate forecasting model based on The Box-Jenkins method. It used annual data of olive-oil production over 58 years from 1961 to 2018. The results showed that the time series of olive-oil in Syria is non-stationary, and it is characterized by three trends: the first is a general
trend that tends to rise; the second is a cyclical trend, i.e., biennial bearing (BB)that leads to the exchange of production every other year; and the third is a random trend resulting from abnormal climate changes or security disorders and their economic effects. The biennial bearing phenomenon is characterized by instability and irregularity, which made forecasting more difficult.
It has been found that BB was responsible for 21.7% of the rise in production over the years of positive/bearing/production (increase above the general production of the series). In contrast, this factor was responsible for 18.9% of the decline in production over the years of negative/non-bearing production.
The best model for forecasting live-oil production was ARIMA (3.1.1), but the lag parameter Yt-2 was non-significant. The self-regression parameters (AR) reveal that the behavior of this time series has often been determined by its values in the first and third recent years, while the moving average parameters (MA) indicate that the behavior of the time series (Yt)is often determined in terms of current random noise and previous random noise.
Keywords:
Olive production in Syria, Box-Jenkins models, time series, biennial/alternate bearing, cyclical trends, ARIMA
References:
[1] Esmander, E. (2014). “Difficulties of exporting and marketing olives”. Working Paper, Export Developing Authority, Ministry of Economy, Damascus, Syria.
[2] Al-Yusuf, Husam (2006): “A study on olive-oil sector in Syria”, Foreign Trade Centre, Ministry of Economy and Trade, Syria, P. 8.
[3] Naser S. (2004). “Structural construction of olive tree, contribution to solve the biennial bearing phenonmennon”. Jordanian Royal University Journal, vol. 37. No 3
[4] Mouhammad M. (2009). “Syrian and world trade of olive oil and relevant agricultural policies”, National Agricultural Policy Center (NAPC), Syria.
[5] Mansour F., JaradS. (2009). “Value chain analysis for olive sector in Syria”. National Agricultural Policy Center (NAPC), Syria
[6] Food and Agricultural Organization of United Nations (FAO) (2013), 2016. “Statistical Data baseolive oil”. Networks, Rome, Italy. Available from: htt://faostat.fao.org.
[7] Agricultural Statistical Abstracts for the year 2006 and 2017. Ministry of Agriculture and Agrarian Reform MAAR, Damascus, Syria.
[8] Pirece,A.D.(1971),"Least Squares Estimation in the Regression Model withAutoregresseion-Moving Average Errors",Biomatrika,Vol.58,P(299-321).
[9] Nuno Crato (1996), “Some Results on the Spectral Analysis of Stationary TimeSeries” Portugal Mathematic Vol. 53.PP 87.
[10] Dickey D. and Fuller W. (1979), “Distribution of the estimators for Autoregressive Time SeriesWith a unit Root ", Journal of the American Statistical
Association, n74: pp .427-431.
[11] Dickey D. and Fuller W. (1981) '”The likelihood Ratio Statistics for Autoregressive Time Series with a Unit Root", Econometric, n49: pp .1057-1072.
[12] Box, G. E. P., and Jenkins, G. M. (1976). Time Series Analysis Forecasting and Control, second ed., Holden-Day, San Francisco.
[13] Shaarawi, S. (2005). “Introduction to modern analysis of time series”. Center for Scientific Publishing, King AbdulAziz University, Saudi
Arabia.
[14] Brockwell, P. J. and Davis, R. A. (1996). “Introduction to Time Series and Forecasting”, Springer-Verlag, New York.
[15] Armstrong S. J., Collopy F. (1992). “Error measures for generalizing about forecasting Methods: Empirical comparisons”. International Journal of
Forecasting, no.8, Pp 69-80.
[16] Al-Taei F. G. A. (2003) "A Comparative Study between Box and Jinken Methods and the Modified Technology Method." PhD Thesis, Faculty of
Computer Science and Mathematics, University of Mosul, Iraq.
[17] Ljung, G.M. and Box G.E.P. (1978) "on a measure of the lack of fit in time Series models".Biometrika, n65:PP.297-303.
[18] Brockwell, P. J. and Davis, R. A. (1991). “Time Series: Theory and Methods”, second Edition, Springer-Verlag, New York.
[19] Jubouri, W. D. S., (2010). Predicting the inflation level of monthly consumer prices in Iraq using binomial variables-time series. Master of Statistics,
Faculty of Management and Economics, Mustansiriya University.
[20] Shaza Mahmoud Sabbouh, Smaan Alattuan, Ibrahim Mohammad Abdullah, "The Technical and Economic Efficiency of Olive Oil Production in the Syrian Coast" SSRG International Journal of Agriculture & Environmental Science 6.1 (2019): 62-66.