A Pilot Study to Explore the Possibility of Combining Field and Remotely Sensed Data for Agricultural Resources Management
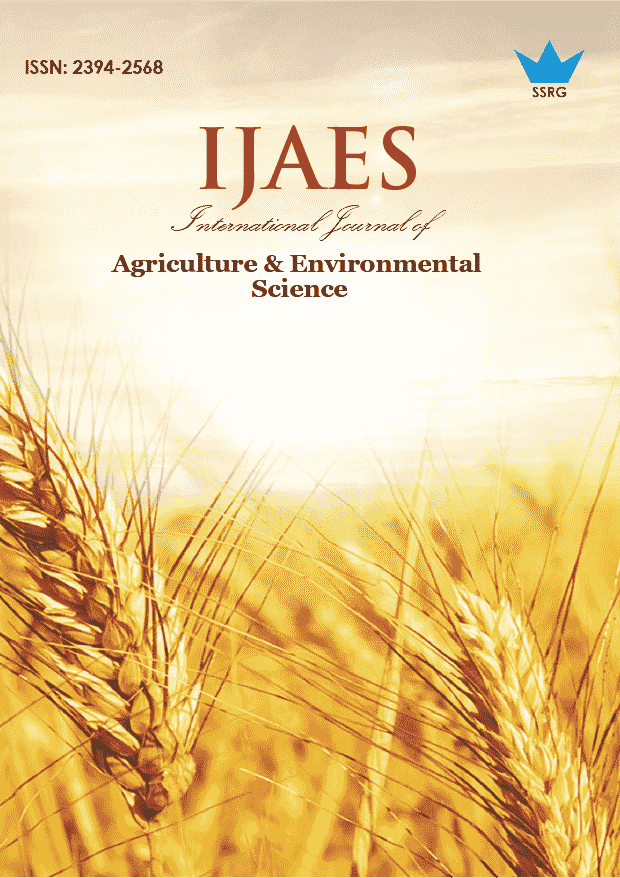
International Journal of Agriculture & Environmental Science |
© 2017 by SSRG - IJAES Journal |
Volume 4 Issue 4 |
Year of Publication : 2017 |
Authors : Prabisha Shrestha, Rita Sharma, Buddhi Raj Gyawali, Essence Carlisle, Dejah Hopkins |
How to Cite?
Prabisha Shrestha, Rita Sharma, Buddhi Raj Gyawali, Essence Carlisle, Dejah Hopkins, "A Pilot Study to Explore the Possibility of Combining Field and Remotely Sensed Data for Agricultural Resources Management," SSRG International Journal of Agriculture & Environmental Science, vol. 4, no. 4, pp. 100-103, 2017. Crossref, https://doi.org/10.14445/23942568/IJAES-V4I4P117
Abstract:
Soil moisture and temperature are important measures of soil productivity, but monitoring agricultural stresses such as water shortage and extreme temperature at large scale farming can be difficult. This research is a pilot study conducted on a research plot to explore the possibility of utilizing both field data and remotely sensed data for agricultural management. The DJI Inspire 1 drone was used to collect aerial images of the plot while Davis soil moisture and temperature station was used to collect field measurements from the plot. Results from the study show field data on soil moisture and temperature can provide insights on to movement of soil water and remotely sensed data such as aerial images and digital surface models can be used to quantify crop conditions with normalized difference vegetation index (NDVI) Such combination of on-ground and off-ground data could provide a holistic approach to observe field conditions and crop health that allows monitoring crops throughout growth and development cycle and planning for optimal harvest.
Keywords:
Soil Moisture, Soil Temperature, Remotely sensed data, aerial images.
References:
[1] Doering, C. (2014, March 23). Growing use of drones poised to transform agriculture. USA Today. Retrieved 11 June, 2017 from https://www.usatoday.com/story/money/business/2014/03/23/drones-agriculture-growth/6665561/.
[2] ESRI. 2016. ArcGIS Desktop. v. 10.5. Redlands, CA: Environmental Systems Research Institute.
[3] ESRI. 2016. Curvature function. Redlands, CA: Environmental Systems Research Institute.
Retrieved 21 June, 2017 from http://desktop.arcgis.com/en/arcmap/10.3/manage-data/raster-and-images/curvature-function.htm [4] Hassan-Esfahani, L., Torres-Rua, A., Jensen, A., & McKee, M. (2015). Assessment of surface soil moisture using high-resolution multi-spectral imagery and artificial neural networks. Remote Sensing, 7(3), 2627-2646.
[5] Lobell, D. B., Ortiz-Monasterio, J. I., Asner, G. P., Naylor, R. L., & Falcon, W. P. (2005). Combining field surveys, remote sensing, and regression trees to understand yield variations in an irrigated wheat landscape. Agronomy Journal, 97(1), 241-249.
[6] Pix4D SA. 2017. Pix4D. v. 3.42. Lausanne, Switzerland.
[7] Wihbey, J. (September 22, 2015). Agricultural drones may change the way we farm. The Boston Globe. Retrieved 1 July, 2017 from https://www.bostonglobe.com/ideas/2015/08/22/agricultural-drones-change-way-farm/WTpOWMV9j4C7kchvbmPr4J/story.html.
[8] Yang, Y., Guan, H., Long, Di., Liu, B., Qin, G. and Batelaan, O. (2015). Estimation of surface soil moisture from thermal infrared remote sensing using an improved trapezoid method. Remote Sensing 7, 8250-8270.
[9] Zhuo, L., Han, D. and Dai, Q. (2016). Soil moisture deficit estimation using satellite multi-angle brightness temperature. Journal of Hydrology 539, 392-405.