Change Detection, Modeling, and Simulation of LCLU Using Multispectral Satellite Images for the Sustainability Development in Najaf City, Iraq
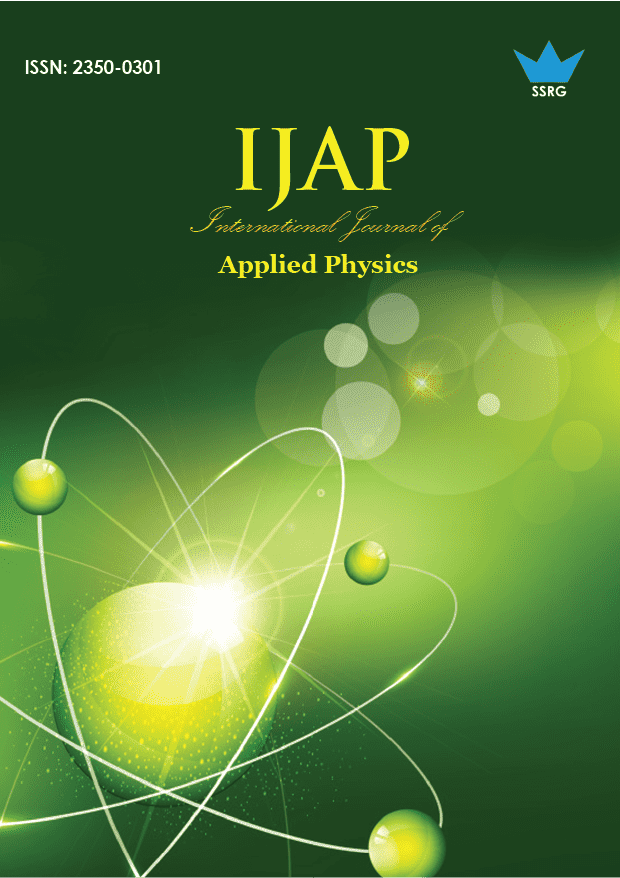
International Journal of Applied Physics |
© 2021 by SSRG - IJAP Journal |
Volume 8 Issue 2 |
Year of Publication : 2021 |
Authors : Kawther Hussein Mohammed, Ahmed Asal Kzar |
How to Cite?
Kawther Hussein Mohammed, Ahmed Asal Kzar, "Change Detection, Modeling, and Simulation of LCLU Using Multispectral Satellite Images for the Sustainability Development in Najaf City, Iraq," SSRG International Journal of Applied Physics, vol. 8, no. 2, pp. 64-71, 2021. Crossref, https://doi.org/10.14445/23500301/IJAP-V8I2P109
Abstract:
Sustainability development is the most important and dangerous issue globally, in the present and future. In this study, remote sensing technology represented by multispectral Landsat images is used to find change detection in classes of land cover and land use that are considered part of the sustainable development goals in this city. The adopted duration time are 2000, 2005, 2009, 2015, and 2020 with five multispectral Landsat images. ERDAS Imagine 2015 is the main program used in this study, where the maximum likelihood method is used for the supervised classification. The results are classified images with accuracies 92.13%, 90.91%, 89.74%, 88.39%, and 85.22%, whereas Kappa coefficient 0.8668, 0.8486, 0.8413, 0.8296, and 0.7805 respectively. The change detection of the area for each class during these years has been realized. The results are an increase in the water bodies area by 6.546%, agricultural lands by 2.8%, And urban land area by 7.719%. In contrast, there is a decrease in the bare lands by -17.068%. The results were followed by modeling from the adopted period, then a simulation for all classes of LCLU for period years (2025- 2050). The outcomes of this study gave useful information for sustainability development through providing a benefit to government institutions related to urban planning, water resources, environment, and agriculture in Al-Najaf city, Iraq. The outcomes of this study are considered as part of achievements that related to the 17 goals of 2015 Paris agreement that should be verified in 2030.
Keywords:
Sustainability development; Remote Sensing; Multispectral; change detection; image Classification.
References:
[1] FAN, Fenglei; WENG, Qihao; WANG, Yunpeng. Land use and land cover change in Guangzhou, China, from 1998 to 2003, based on Landsat TM/ETM+ imagery. Sensors, 7(7) (2007) 1323-1342.
[2] C. Brodhag, F. Breuil, N. Gondran, and F. Ossama, Dictionnaire du développement durable, 284 (2004 ) Afnor Paris.
[3] A. Kavvada et al., Towards delivering on the sustainable development goals using earth observations., Elsevier, (2020).
[4] R. J. Baumgartner., Sustainable development goals and the forest sector—A complex relationship, Forests, 10(2) (2019) 152.
[5] H. Q. Hamdy and O. H. Mutlag., Land Cover Change Detection in Al-Karkh/Baghdad,” Int. J. Sci. Res., 9(1) (2018).
[6] M. Butenuth, G. v Gösseln, M. Tiedge, C. Heipke, U. Lipeck, and M. Sester., Integration of heterogeneous geospatial data in a federated database, ISPRS J. Photogramm. Remote Sens., 62(5) (2007) 328–346.
[7] S. D. Prince., Challenges for remote sensing of the Sustainable Development Goal SDG 15.3. 1 productivity indicator, Remote Sens. Environ., 234(2019) 111428.
[8] O. Ololade, H. J. Annegarn, D. Limpitlaw, and M. A. Kneen, Land-use/cover mapping and change detection in the Rustenburg Mining Region using Landsat images, in IGARSS 2008-2008 IEEE International Geoscience and Remote Sensing Symposium, 4(2008) 4–818.
[9] O. Şatır and S. Berberoğlu., Land use/cover classification techniques using optical remotely sensed data in landscape planning, Landsc. Planning, InTech, Shanghai, China, (2012) 21–55.
[10] A. A. Belal and F. S. Moghanm., Detecting urban growth using remote sensing and GIS techniques in Al Gharbiya governorate, Egypt, Egypt. J. Remote Sens. Sp. Sci., 14(2) (2011) 73–79.
[11] S. Abburu and S. B. Golla., Satellite image classification methods and techniques: A review, Int. J. Comput. Appl., 119(8) (2015).
[12] S. G. Tesfamichael and I. T. Rampedi., Remote sensing of water quality indicators associated with mining activities: the case study of Mooi River in Carletonville, Gauteng Province, South Africa, (2020).
[13] S. H. Ali., Utilities of GIS and Remote Sensing Data in the Spatial Analysis of the Radioactive Contamination Site in Nineveh Province, J. Earth Sci. Geotech. Eng., 3(3) (2013) 223–239.
[14] P. Gibas and A. Majorek., Use of Land Use Change Analysis between 2012–2018 to Assessment of Sustainable Development in Europe, (2019).
[15] A. Y. Kwarteng and P. S. Chavez Jr., Change detection study of Kuwait City and environs using multi-temporal Landsat Thematic Mapper data, Int. J. Remote Sens., 19(9) (1998) 1651–1662.
[16] Z. E. Hussein, R. H. Hasan, and N. A. Aziz., Detecting the changes of AL-Hawizeh Marshland and surrounding areas using GIS and remote sensing techniques, Assoc. Arab Univ. J. Eng. Sci., 25(1) (2018) 53–63.
[17] K. Alkaradaghi, S. S. Ali, N. Al-Ansari, and J. Laue., Land use classification and change detection using multi-temporal Landsat imagery in Sulaimaniyah Governorate, Iraq, in Conference of the Arabian Journal of Geosciences, (2018) 117–120.
[18] A. M. Sabea and A. H. N. Mashhadani., Monitoring the changes between the land cover and land use in the area of Amiriya Fallujah, J. Tikrit Univ. Humanit. 25(10) (2019) 185–203.
[19] Y. T. Mustafa and D. R. Ismail., Land Use Land Cover Change in Zakho District, Kurdistan Region, Iraq: Past, Current and Future, in 2019 International Conference on Advanced Science and Engineering (ICOASE) (2019) 141–146.
[20] K. H. Fahad, S. Hussein, and H. Dibs., Spatial-Temporal Analysis of
Land Use and Land Cover Change Detection Using Remote Sensing and GIS Techniques, in IOP Conference Series: Materials Science and Engineering, 671(1) (2020) 12046.
[21] S. W. Wang, L. Munkhnasan, and W.-K. Lee., Land use and land cover change detection and prediction in Bhutan’s high altitude city of Thimphu, using cellular automata and Markov chain, Environ. Challenges, 2(2021) 100017.
[22] R. G. Congalton and K. Green, Assessing the accuracy of remotely sensed data: principles and practices. CRC Press, (2019).
[23] Rei Sonobe, Yuki Yamaya, Hiroshi Tani, et al. Mapping crop cover using multi-temporal Landsat 8 OLI imagery. International Journal of Remote Sensing 38(15) (2017) 4348-4361.
[24] Alex O. Onojeghuo, George A. BlackburnI, Qunming Wang, Peter M. Atkinson, Daniel Kindred, and Yuxin Miao. Mapping paddy rice fields by applying machine learning algorithms to multi-temporal Sentinel-1A and Landsat data. International Journal of Remote Sensing 39(4) (2017) (2018) 1042-1067.
[25] Ya’nan Zaho, Jiancheng Luo, Li Feng, Yingpin Yang, Yuehong Chen and Wei Wu. Long-short-term-memory-based crop Classification using high-resolution optical images and multi-temporal SAR data. GIScience & Remote Sensing., 56(8) (2019) 1170-1191.
[26] Natural Resource Canada 2010. http://www.ccrs.nrcan.gc.ca/resource/tutor/ fundam/chapter4/07_e.php. Accessed 20 February (2010).
[27] P.J., Curran and E. M. M. Novo., The relationship between suspended sediment concentration and remotely sensed spectral radiance: a review, J. Coast. Res., (1988) 351–368.
[28] Francis D. Chizea and Benjamin G. Ayantunji, Accuracy assessment of nigeriasat-x and landsat images for landuse/landcover analyses in enugu state,Nigeria, SSRG International Journal of Applied Physics 6(3) (2019) 9-15.