Spatial Planning Under Data Paucity: Dasymetric Interpolation of Population, Validated by Google Earth, to Support Health Facility Location Modelling
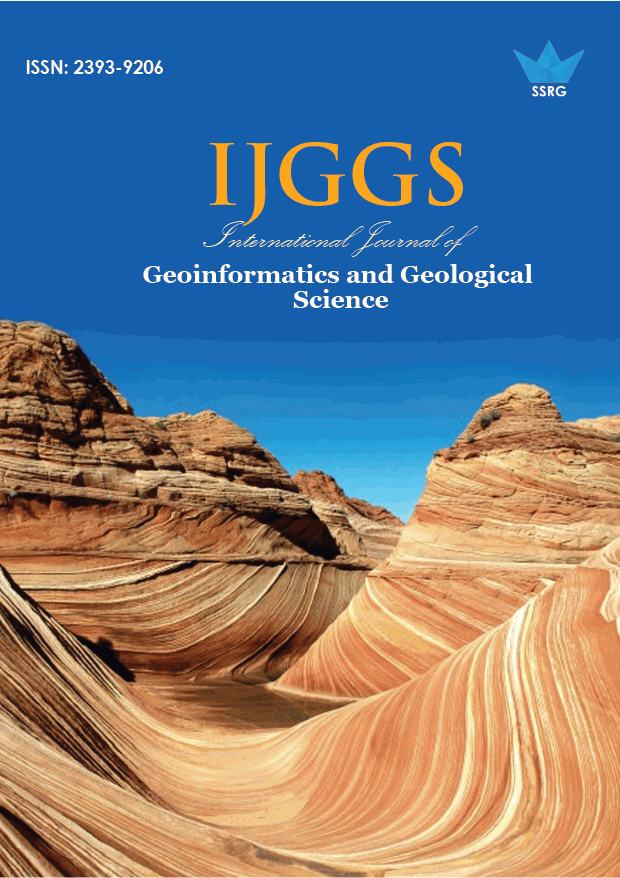
International Journal of Geoinformatics and Geological Science |
© 2017 by SSRG - IJGGS Journal |
Volume 4 Issue 2 |
Year of Publication : 2017 |
Authors : Idris Mohammed Jega, Alexis J. Comber, Nicholas J. Tate |
How to Cite?
Idris Mohammed Jega, Alexis J. Comber, Nicholas J. Tate, "Spatial Planning Under Data Paucity: Dasymetric Interpolation of Population, Validated by Google Earth, to Support Health Facility Location Modelling," SSRG International Journal of Geoinformatics and Geological Science, vol. 4, no. 2, pp. 1-11, 2017. Crossref, https://doi.org/10.14445/23939206/IJGGS-V4I3P101
Abstract:
Small area population estimates are important for facility location-allocation analyses as they provide a spatial distribution of „demand‟ against which potential „supply‟ locations are evaluated. However, in many parts of the world small area estimates of census data are not available which makes it difficult to validate and constrain interpolated population or demand surfaces. For such cases a number of interpolation methods have been proposed to redistribute summary population census totals over small areas. Binary dasymetric interpolation has been shown to perform well across a range of spatial scales and resolutions supported by ancillary data. To date no published research describes the use of dasymetric approaches as methods for disaggregating population data over small areas in any part of West Africa. This study applies a binary dasymetric approach to generate population surface for Port-Harcourt, Nigeria, an area for which small area population estimates are unavailable. This was validated by visual inspection using Google Earth and found to be 87% correct. The demand surface was then used as input to a location-allocation analysis of health facility provision. The locations of current primary healthcare centres (PHCCs) were evaluated and then alternative, improved spatial arrangement for the facilities that optimised the spatial distribution of supply with that of demand, were suggested. The results show 13 alternative sites for PHCCs to be located would provide almost the same demand coverage as the 17 current locations of PHCCs. The analysis indicated that fewer PHCCs in a different spatial arrangement could satisfy the spatial distribution of demand. The results suggest that such methods can be used to support and inform decision making and spatial planning in countries where only limited socioeconomic data exist.
Keywords:
Small area population estimates, binary dasymetric mapping, health facility locationallocation, spatial planning.
References:
[1]. Cromley, R.G., J. Lin, and D.A. Merwin, Evaluating representation and scale error in the maximal covering location problem using GIS and intelligent areal interpolation. International Journal of Geographical Information Science, 2012. 26(3): p. 495-517.
[2]. Tomintz, M.N., et al., Optimising the location of antenatal classes. Midwifery, 2013. 29(1): p. 33-43.
[3]. Eicher, C.L. and C.A. Brewer, Dasymetric mapping and areal interpolation: Implementation and evaluation. Cartography and Geographic Information Science, 2001. 28(2): p. 125-138.
[4]. Mennis, J., Generating surface models of population using dasymetric mapping∗. The Professional Geographer, 2003. 55(1): p. 31-42.
[5]. Langford, M., Rapid facilitation of dasymetric-based population interpolation by means of raster pixel maps. Computers, Environment and Urban Systems, 2007. 31(1): p. 19-32.
[6]. Langford, M., et al., Urban population distribution models and service accessibility estimation. Computers, Environment and Urban Systems, 2008. 32(1): p. 66-80.
[7]. Cai, Q., et al., Estimating Small‐ Area Populations by Age and Sex Using Spatial Interpolation and Statistical Inference Methods. Transactions in GIS, 2006. 10(4): p. 577-598.
[8]. Higgs, G. and M. Langford, GIScience, environmental justice, & estimating populations at risk: The case of landfills in Wales. Applied Geography, 2009. 29(1): p. 63-76.
[9]. Maantay, J.A., A.R. Maroko, and H. Porter-Morgan, Research Note—A New Method for Mapping Population and Understanding the Spatial Dynamics of Disease in Urban Areas: Asthma in the Bronx, New York. Urban Geography, 2013. 29(7): p. 724-738.
[10]. Comber, A., C. Proctor, and S. Anthony, The creation of a national agricultural land use dataset: combining pycnophylactic interpolation with dasymetric mapping techniques. Transactions in GIS, 2008. 12(6): p. 775-791.
[11]. Poulsen, E. and L.W. Kennedy, Using dasymetric mapping for spatially aggregated crime data. Journal of Quantitative Criminology, 2004. 20(3): p. 243-262.
[12]. Herrmann, C. and A. Maroko, Crime pattern analysis: exploring Bronx auto thefts using GIS. GIS for the Urban Environment. Redlands, CA: Environmental Systems Research Institute (ESRI), 2006: p. 407-413.
[13]. Langford, M., An Evaluation of Small Area Population Estimation Techniques Using Open Access Ancillary Data. Geographical Analysis, 2013. 45(3): p. 324-344.
[14]. Mennis, J. and T. Hultgren, Intelligent dasymetric mapping and its application to areal interpolation. Cartography and Geographic Information Science, 2006. 33(3): p. 179-194.
[15]. Martin, D., N.J. Tate, and M. Langford, Refining population surface models: experiments with Northern Ireland census data. Transactions in GIS, 2000. 4(4): p. 343-360.
[16]. Wright, J.K., A method of mapping densities of population: With Cape Cod as an example. Geographical Review, 1936. 26(1): p. 103-110.
[17]. Petrov, A.N., Setting the Record Straight: On the Russian Origins of Dasymetric Mapping. Cartographica: The International Journal for Geographic Information and Geovisualization, 2008. 43(2): p. 133-136.
[18]. Langford, M. and D.J. Unwin, Generating and mapping population density surfaces within a geographical information system. The Cartographic Journal, 1994. 31(1): p. 21-26.
[19]. Langford, M. and G. Higgs, Measuring potential access to primary healthcare services: the influence of alternative spatial representations of population. The Professional Geographer, 2006. 58(3): p. 294-306.
[20]. Xie, Y., The overlaid network algorithms for areal interpolation problem. Computers, environment and urban systems, 1995. 19(4): p. 287-306.
[21]. Mrozinski, R.D. and R.G. Cromley, Singly‐ and Doubly‐ Constrained Methods of Areal Interpolation for Vector‐ based GIS. Transactions in GIS, 1999. 3(3): p. 285-301.
[22]. Maantay, J.A., A.R. Maroko, and C. Herrmann, Mapping Population Distribution in the Urban Environment: The Cadastral-based Expert Dasymetric System (CEDS). Cartography and Geographic Information Science, 2007. 34(2): p. 77-102.
[23]. Zandbergen, P.A. and D.A. Ignizio, Comparison of dasymetric mapping techniques for small-area population estimates. Cartography and Geographic Information Science, 2010. 37(3): p. 199-214.
[24]. Tapp, A.F., Areal interpolation and dasymetric mapping methods using local ancillary data sources. Cartography and Geographic Information Science, 2010. 37(3): p. 215-228.
[25]. Kim, H. and X. Yao, Pycnophylactic interpolation revisited: integration with the dasymetric-mapping method. International Journal of Remote Sensing, 2010. 31(21): p. 5657-5671.
[26]. Cockings, S., P.F. Fisher, and M. Longford, Parameterization and visualization of the errors in areal interpolation. Geographical Analysis, 1997. 29(4): p. 314-328.
[27]. Fisher, P.F. and M. Langford, Modelling the errors in areal interpolation between zonal systems by Monte Carlo simulation. Environment and Planning A, 1995. 27(2): p. 211-224.
[28]. Langford, M., P. Fisher, and D. Troughear. Comparative accuracy measurements of the cross areal interpolation of population. in Proceedings of EGIS. 1993.
[29]. Harris, R.J. and P.A. Longley, New data and approaches for urban analysis: modelling residential densities. Transactions in GIS, 2000. 4(3): p. 217-234.
[30]. Green, N.E., Aerial photographic analysis of residential neighborhoods: An evaluation of data accuracy. Soc. F., 1956. 35: p. 142.
[31]. Bentley, G.C., R.G. Cromley, and C. Atkinson-Palombo, The Network Interpolation of Population for Flow Modeling Using Dasymetric Mapping. Geographical Analysis, 2013. 45(3): p. 307-323.
[32]. Goodchild, M.F., L. Anselin, and U. Deichmann, A framework for the areal interpolation of socioeconomic data. Environment and Planning A, 1993. 25(3): p. 383-397.
[33]. Zola K. Moon, F.L.F., Population Density Surface: A New Approach to an Old Problem. Society & Natural Resources, 2001. 14(1): p. 39-51.
[34]. Leyk, S., N.N. Nagle, and B.P. Buttenfield, Maximum Entropy Dasymetric Modeling for Demographic Small Area Estimation. Geographical Analysis, 2013. 45(3): p. 285-306.
[35]. Harvey, J.T., 3opulation Estimation Models 3ased on Individual TM Pixels. Photogrammetric Engineering & Remote Sensing, 2002. 68(11): p. 1181-1192.
[36]. Holt, J.B., C.P. Lo, and T.W. Hodler, Dasymetric Estimation of Population Density and Areal Interpolation of Census Data. Cartography and Geographic Information Science, 2004. 31(2): p. 103-121.
[37]. Chen, K., An approach to linking remotely sensed data and areal census data. International Journal of Remote Sensing, 2002. 23(1): p. 37-48.
[38]. Liu, X., K. Clarke, and M. Herold, Population density and image texture. Photogrammetric Engineering & Remote Sensing, 2006. 72(2): p. 187-196.
[39]. Ward, D., S.R. Phinn, and A.T. Murray, Monitoring growth in rapidly urbanizing areas using remotely sensed data. The Professional Geographer, 2000. 52(3): p. 371-386.
[40]. Pozzi, F., C. Small, and G. Yetman, Modeling the distribution of human population with nighttime satellite imagery and gridded population of the world. Earth Observation Magazine, 2003. 12(4): p. 24-30.
[41]. Briggs, D.J., et al., Dasymetric modelling of small-area population distribution using land cover and light emissions data. Remote Sensing of Environment, 2007. 108(4): p. 451- 466.
[42]. Wu, S.-s., X. Qiu, and L. Wang, Population Estimation Methods in GIS and Remote Sensing: A Review. GIScience & Remote Sensing, 2005. 42(1): p. 80-96.
[43]. Sridharan, H. and F. Qiu, A Spatially Disaggregated Areal Interpolation Model Using Light Detection and Ranging- Derived Building Volumes. Geographical Analysis, 2013. 45(3): p. 238-258.
[44]. Curtis, S. and A. Taket, The development of geographical information systems for locality planning in health care. Area, 1989: p. 391-399.
[45]. Mennis, J., Dasymetric mapping for estimating population in small areas. Geography Compass, 2009. 3(2): p. 727-745.
[46]. Jega, I.M., Estimating population surfaces in areas where actual distributions are unknown: dasymetric mapping and pycnophylactic interpolation across different spatial scales. 2015, Department of Geography.
[47]. Cromley, R.G., A.Y. Ebenstein, and D.M. Hanink, Estimating components of population change from census data for incongruent spatial/ temporal units and attributes. Journal of Spatial Science, 2009. 54(2): p. 89-99.
[48]. Commission, N.P., Nigeria: National Population Census Priority tables, N.P. Commission, Editor. 2006: Nigeria.
[49]. International, A., Forced eviction in Port-Harcourt, Nigeria, “Just move them”. 2010, Amnesty International publication: London.
[50]. Cromley, R.G., D.M. Hanink, and G.C. Bentley, A Quantile Regression Approach to Areal Interpolation. Annals of the Association of American Geographers, 2012. 102(4): p. 763- 777.
[51]. Langford, M., D.J. Maguire, and D.J. Unwin, The areal interpolation problem: estimating population using remote sensing in a GIS framework. Handling geographical information: Methodology and potential applications, 1991: p. 55-77.
[52]. Hakimi, S.L., Optimum locations of switching centers and the absolute centers and medians of a graph. Operations research, 1964. 12(3): p. 450-459.
[53]. Teitz, M.B. and P. Bart, Heuristic Methods for Estimating the Generalized Vertex Median of a Weighted Graph. Operations Research, 1968. 16(5): p. 955-961.
[54]. ReVelle, C.S. and R.W. Swain, Central facilities location. Geographical analysis, 1970. 2(1): p. 30-42.
[55]. Church, R.L. and P. Sorensen, Integrating normative location models into GIS: problems and prospects with thep-median model. Spatial analysis: modelling in a GIS environment, 1996: p. 179-190.
[56]. Narula, S.C., U.I. Ogbu, and H.M. Samuelsson, Technical Note—An Algorithm for thep-Median Problem. Operations Research, 1977. 25(4): p. 709-713.
[57]. Maranzana, F., On the location of supply points to minimize transport costs. OR, 1964: p. 261-270.
[58]. Holland, J.H., Adaptation in natural and artificial systems: an introductory analysis with applications to biology, control, and artificial intelligence. 1975: U Michigan Press.
[59]. Glover, F., Tabu search: A tutorial. Interfaces, 1990. 20(4): p. 74-94.
[60]. Policy, C.f.H., The determination of need norms for health services, Part 2. A summary of norms in other countries, N.H.a.P. Development, Editor. 1993.
[61]. Rispel, L., et al., A description and evaluation of primary care services delivered by the Alexandra Health Centre and University Clinic. 1995, Technical Report. Johannesburg: The Centre for Health Policy, Department of Community Health, University of the Witwatersrand.
[62]. Doherty, J., L. Rispel, and N. Webb, Developing a plan for primary health care facilities in Soweto, South Africa. Part II: Applying locational criteria. Health policy and planning, 1996. 11(4): p. 394-405.
[63]. Fisher, P.F. and M. Langford, Modeling Sensitivity to Accuracy in Classified Imagery: A Study of Areal Interpolation by Dasymetric Mapping∗. The Professional Geographer, 1996. 48(3): p. 299-309.
[64]. Seidel, J.E., et al., Location of residence associated with the likelihood of patient visit to the preoperative assessment clinic. BMC Health Serv Res, 2006. 6: p. 13.
[65]. Kohli, S., et al., Distance from the Primary Health Center: a GIS method to study geographical access to health care. Journal of Medical Systems, 1995. 19(6): p. 425-436.
[66]. Payne, S., N. Jarrett, and D. Jeffs, The impact of travel on cancer patients‟ experiences of treatment: a literature review. European Journal of Cancer Care, 2000. 9(4): p. 197-203.
[67]. Brewer, N., et al., Travel time and distance to health care only partially account for the ethnic inequalities in cervical cancer stage at diagnosis and mortality in New Zealand. Australian and New Zealand Journal of Public Health, 2012. 36(4): p. 335- 342.
[68]. Jamil, K., et al., The immunization programme in Bangladesh: impressive gains in coverage, but gaps remain. Health Policy and Planning, 1999. 14(1): p. 49-58.
[69]. Acharya, L.B. and J. Cleland, Maternal and child health services in rural Nepal: does access or quality matter more? Health Policy and Planning, 2000. 15(2): p. 223-229.
[70]. Tanser, F., B. Gijsbertsen, and K. Herbst, Modelling and understanding primary health care accessibility and utilization in rural South Africa: an exploration using a geographical information system. Soc Sci Med, 2006. 63(3): p. 691-705.
[71]. Kristensen, I., et al., Routine vaccinations and child survival: follow up study in Guinea-Bissau, West AfricaCommentary: an unexpected finding that needs confirmation or rejection. Bmj, 2000. 321(7274): p. 1435.
[72]. Arevshatian, L., et al., An evaluation of infant immunization in Africa: is a transformation in progress? Bulletin of the World Health Organization, 2007. 85(6): p. 449-457.
[73]. Gu, W., X. Wang, and S.E. McGregor, Optimization of preventive health care facility locations. International Journal of Health Geographics, 2010. 9(1): p. 1.
[74]. Hemat, S., et al., Health-care provision factors associated with child immunization coverage in a city centre and a rural area in Kabul, Afghanistan. Vaccine, 2009. 27(21): p. 2823-9.
[75]. Programme, U.N.D., Millennium Development Goal, U.N.D. Programme, Editor. 2000.