Industrial Pipelines Breakdown Prediction
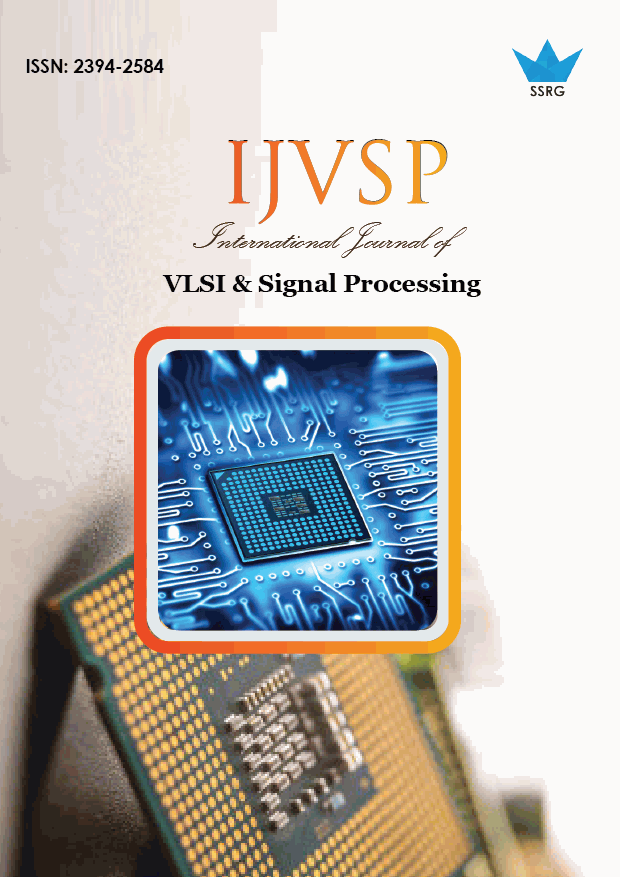
International Journal of VLSI & Signal Processing |
© 2021 by SSRG - IJVSP Journal |
Volume 8 Issue 1 |
Year of Publication : 2021 |
Authors : V.Lohalakshmi , B.Sureka, D.Krishnaveni, P.Shunmugapriya, K.Karthik |
How to Cite?
V.Lohalakshmi , B.Sureka, D.Krishnaveni, P.Shunmugapriya, K.Karthik, "Industrial Pipelines Breakdown Prediction," SSRG International Journal of VLSI & Signal Processing, vol. 8, no. 1, pp. 14-20, 2021. Crossref, https://doi.org/10.14445/23942584/IJVSP-V8I1P104
Abstract:
An imbalanced order issue is an illustration of an arrangement issue where the conveyance of models across the realized classes is one-sided or slanted. The dissemination can shift from a slight predisposition to an extreme awkwardness where there is one model in the minority class for hundreds, thousands, or millions of models in the larger part class or classes. Imbalanced orders represent a test for prescient displaying as the vast majority of the AI calculations utilized for characterization were planned around the presumption of an equivalent number of models for each class. These outcomes in models that have poor prescient execution, explicitly for the minority class. This is an issue on the grounds that regularly, the minority class is more significant, and thusly the issue is more delicate to characterization blunders for the minority class than the dominant part class. We proposed a model which handles the imbalanced information and anticipates the shortcoming events and their answer for redress the deficiency just as refreshing the new pipeline disappointment information in the model. In the business issue, correction requires a lot of measure of time and exertion. Finding the mistake is likewise a drawn-out measure. This will lessen the expense and time proficiency in the oil and gas creation ventures.
Keywords:
Oversampling, SMOTE, ADASYN, BORDERLINE SMOTE, SAFE LEVEL SMOTE, class awkwardness issue.
References:
[1] G. Weiss., Mining with Rarity: A Unified Framework, SIGKDD Explorations,6(1)(2004) 7-19.
[2] A. Akarma, F. Sonar., THE USAGE OF RADAR IMAGES IN OIL SPILL DETECTION, ISPRS, 37(2008) Part B8. Beijing.
[3] T. Jo and N. Japkowicz, Class uneven characters versus little disjoints, ACM SIGKDD Explorations Newsletter., 6(1)(2004) 40–49.
[4] S.Maheshwari, Agrawal and Sharma., New methodology for the arrangement of profoundly imbalanced datasets utilizing developmental calculations'', Int. J. Sci. Eng. Res., 2(7)(2011) 1–5.
[5] V. Chawla et al., Destroyed: Synthetic Minority Over Sampling Technique, Journal of Artificial Intelligence Research, 16(2002) 321-357.
[6] N. V. Chawla., Data digging for imbalanced datasets: An outline, Data Mining and Knowledge Discovery Handbook. Springer, (2005) 853–867.
[7] J. A. Saez et al., Overseeing Borderline and Noisy models in Imbalanced Classification by consolidating SMOTE with Ensemble Filtering, IDEAL2014, Springer LNCS, 8669(2014) 61-68.
[8] B.X. Wang and N. Japkowicz, Imbalanced Data Set Learning with Synthetic Samples, Proc. IRIS Machine Learning Workshop, (2004).
[9] H. He, Y. Bai, E. A. Garcia, and S. Li, ADASYN: Adaptive engineered testing approach for imbalanced learning, in Proc. IEEE Int. Joint Conf. Neural Netw., IEEE World Congr. Comput. Intell., (2008) 1322–1328.
[10] C. Bunkhumpornpat, K. Sinapiromsaran, and C. Lursinsap., Safe-
Level-SMOTE: Safe Level-Synthetic MI Over-Sampling Technique for dealing with the Class Imbalance Problem, PADD2009, Springer LNAI, 5476(2009) 475-482.
[11] Guo, X., et al., On the class irregularity issue, Natural Computation, ICNC'08. IEEE, Fourth International Conference, (2008).
[12] Vaishali, G., An Overview Of Classification Algorithms For Imbalanced Datasets, Int Journal of Emerging innovation and Advanced Engineering, 2(4)(2012).
[13] K. P. N. V. Satyashree, and J. V. R. Murthy, "An Exhaustive Literature Review on Class Imbalance Problem", Int. Diary of Emerging Trends and Technology in Computer Science 2(3)(2013) 109-118.
[14] G. E. A. P. A Batista et al., An investigation of the conduct of a few strategies for adjusting AI preparing information", SIGKDD Expel. Newly, 6(1)(2004) 20-29.
[15] G. E. Batista, R. C. Prate, and M. C. Monardo., An investigation of the conduct of a few strategies for adjusting AI preparing information, ACM SIGKDD Explorations Newsletter., 6(1)(2004) 20–29.
[16] NikeshChawla, Nathalie Japkowicz and AlexanderKlotz., Publication: Special Issue on Learning from Imbalanced Data Sets, SIGKDD Explorations 6(1)(2004) 1-6.
[17] J. Huang and C.X. Ling., Utilizing AUC and Accuracy in Evaluating Learning, IEEE Transactions on Knowledge and Data Engineering, 17(3)(2005).
[18] J. A. Hanley, and B. J. McNeil., A strategy for contrasting the regions under recipient working trademark bends got from similar cases", Radiology, 148(3)(1983) 839-843.
[19] A. Amin, S. Anwar., Standing out Oversampling Technique from Handle the Class Imbalance Problem: A Customer Churn Prediction Case Study., IEEE Access.
[20] A. Ali, S.M. Shams Uddin and A. L. Rescue, Classification with class Algorithms awkwardness issue: A Review, Int. J. Advance Soft Compo. Apple,7(3)(2015).