Implementation of Medical Image Fusion using Neuro-Fuzzy Logic
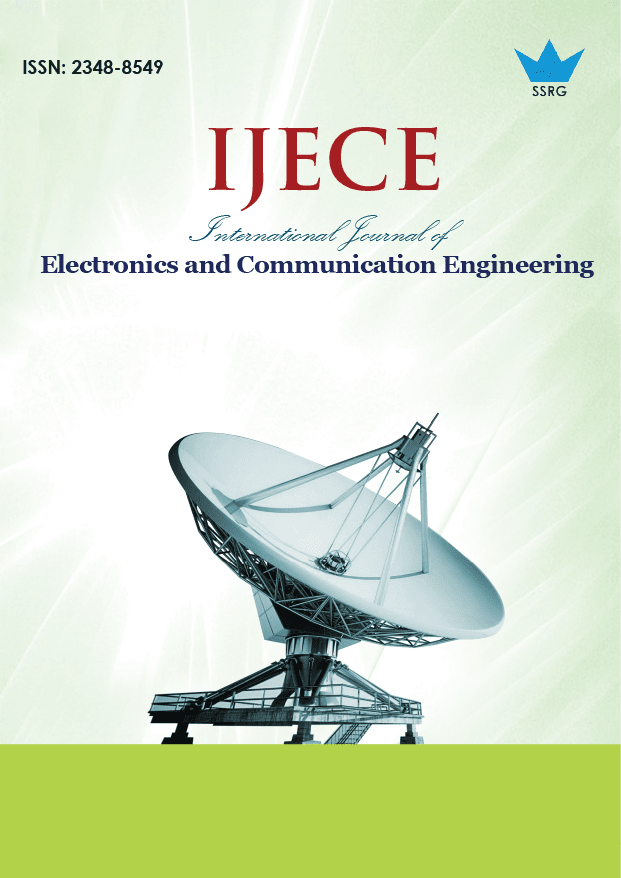
International Journal of Electronics and Communication Engineering |
© 2018 by SSRG - IJECE Journal |
Volume 5 Issue 7 |
Year of Publication : 2018 |
Authors : Mr.Phapale Harish Anil and Prof.Bansode Rahul Sitaram |
How to Cite?
Mr.Phapale Harish Anil and Prof.Bansode Rahul Sitaram, "Implementation of Medical Image Fusion using Neuro-Fuzzy Logic," SSRG International Journal of Electronics and Communication Engineering, vol. 5, no. 7, pp. 1-6, 2018. Crossref, https://doi.org/10.14445/23488549/IJECE-V5I7P101
Abstract:
This paper gets a handle on medical image fusion (MIF) problems. These problems are simply known as image fusion problems.We can use RPCNN(Reduced Pulse coupled neural network)to provide better performance .The RPCNN linking dependents on fuzzy membership values. This value serves their significance in the analogous source image. The RPCNN has less complex structurekey advantage of such structure is that RPCNN require less number of parameters. The less complex structure and less parameter improve computational efficiency. This computational efficiency is important requirement of point-of-care (POC) health care technologies. The proposed scheme is free from the trivial defalcation of the modernization of MIF techniques: contrast reduction, loss of image fine details and unwanted image degradations etc.
Keywords:
Artificial neural network, fuzzy logic, image analysis,image fusion (IF), medical imaging (MI).
References:
[1] B. Solaiman, R. Debon, F. Pipelier, J. M. Cauvin, and C. Roux, “Informationfusion: Application to data and model fusion for ultrasound image segmentation,” IEEE Trans. Biomed. Eng., vol. 46, no. 10, pp. 1171–1175, Oct. 1999.
[2] V.Barra and J. Y.Boire, “Ageneral framework for the fusion of anatomicaland functional medical images,” NeuroImage, vol. 13, no. 3, pp. 410–424,2001.
[3] S. Das andM. K. Kundu, “NSCT-based multimodal medical image fusion using pulse-coupled neural network and modified spatial frequency,” Med. Biol. Eng. Comput., vol. 50, no. 10, pp. 1105–1114, 2012.
[4] Z.Wang and Y. Ma, “Medical image fusion using m-PCNN,” Inf. Fusion, vol. 9, no. 2, pp. 176–185, Apr. 2008.
[5] R. Eckhorn, H. J. Reitboeck, M. Arndt, and P. Dicke, “Feature linking via synchronization among distributed assemblies: Simulations of results from cat visual cortex,” Neural Comput., vol. 2, pp. 293–307, 1990.
[6] F. C. Z. Wang, Y. Ma, and L. Yang, “Review of pulse-coupled neural networks,” Image Vis. Comput., vol. 28, no. 1, pp. 5–13, 2010.
[7] J. Johnson andM. Padgett, “PCNNmodels and applications,” IEEE Trans.NeuralNetw., vol. 10, no. 3, pp. 480–498, May 1999.
[8] Q. Xiao-Bo, Y. Jing-Wen, X. Hong-Zhi, and Z. Zi-Qian, “Image fusion algorithm based on spatial frequency-motivated pulse coupled neural networks in nonsubsampledcontourlet transform domain,” ActaAutomaticaSinica, vol. 34, no. 12, pp. 1508–1514, 2008.
[9] Z. Wang, Y. Ma, and J. Gu, “Multi-focus image fusion using PCNN,” Pattern Recognit., vol. 43, no. 6, pp. 2003–2016, Jun. 2010.
[10] J. M. Kinser, “Foveation by a pulse-coupled neural network,” IEEE Trans. Neural Netw., vol. 10, no. 3, pp. 621–626, 1999.
[11] M. K. Kundu and S. K. Pal, “Automatic selection of object enhancement operator with quantitative justification based on fuzzy set theoretic measure,” Pattern Recognit. Lett., vol. 11, pp. 811–829, 1990.
[12] J. A. Karvonen, “Baltic sea ice SAR segmentation and classification using modified pulse-coupled neural networks,” IEEE Trans. Geosci. Remote Sens., vol. 42, no. 7, pp. 1566–1574, Jul. 2004.
[13] C. Barillot, D. Lemoine, L. L. Briquer, F. Lachmann, B. Gibaud, Data fusion in medical imaging: merging multimodal and multipatient images, identification of structures and 3D display aspects, European journal of radiology 17 (1) (1993) 22–27.