Phoneme Modeling for Speech Recognition in Kannada using Multivariate Bayesian Classifier
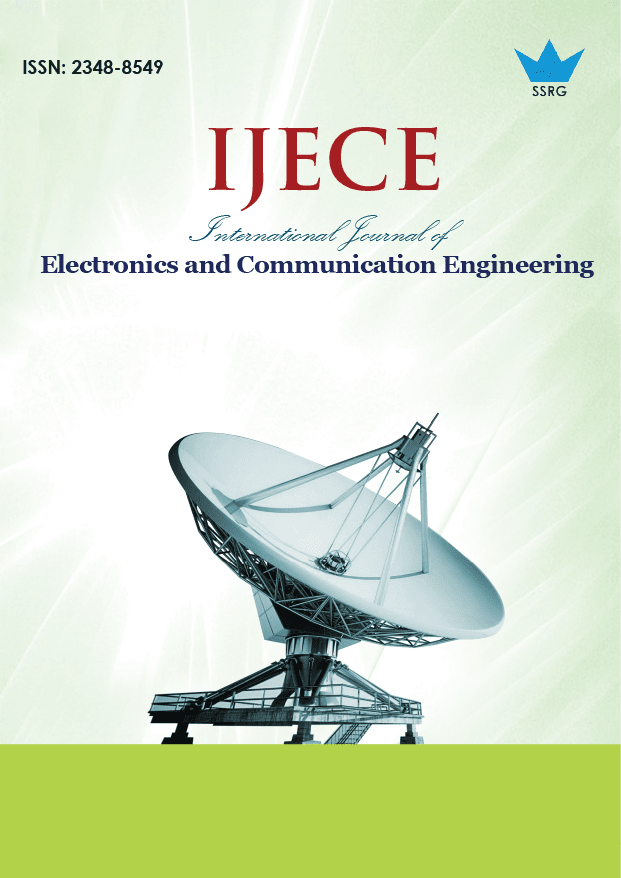
International Journal of Electronics and Communication Engineering |
© 2014 by SSRG - IJECE Journal |
Volume 1 Issue 9 |
Year of Publication : 2014 |
Authors : Prashanth Kannadaguli and Vidya Bhat |
How to Cite?
Prashanth Kannadaguli and Vidya Bhat, "Phoneme Modeling for Speech Recognition in Kannada using Multivariate Bayesian Classifier," SSRG International Journal of Electronics and Communication Engineering, vol. 1, no. 9, pp. 1-4, 2014. Crossref, https://doi.org/10.14445/23488549/IJECE-V1I9P101
Abstract:
We build an automatic phoneme recognition system based on Bayesian Multivariate Modeling which is a static scheme. Phoneme models were built by using stochastic pattern recognition and acoustic phonetic schemes to recognise phonemes. Since our native language is Kannada, a rich South Indian Language, we have used 15 Kannada phonemes to train and test these models. As Mel – Frequency Cepstral Coefficients (MFCC) are well known acoustic features of speech, we have used the same in speech feature extraction. Finally performance analysis of models in terms of Phoneme Error Rate (PER) justifies the fact that though static modeling yields good results, improvization is necessary in order to use it in developing Automatic Speech Recognition systems
Keywords:
Bayesian Classification, Kannada, MFCC, Pattern Recognition; PER, Phoneme Modeling
References:
[1] Lawrence R. Rabiner, B. H. Juang, “Fundamentals of Speech recognition”, 2nd Indian Reprint, Pearson Education, pp 103-455, Delhi, 1993.
[2] Y. Lee and K.W. Hwang, “Selecting Good Speech Features for Recognition” ETRI, vol. 18, Apr. 1996.
[3] K. F. Lee and H. W. Hon, “Speaker-independent phone recognition using hidden Markov models”, IEEE Transactions on Acoustics, Speech and Signal Processing, vol. 37, pp. 1641-1648, 1989.
[4] S. Young, “The general use of tying in phoneme based HMM speech recognition”, proceedings of ICASSP, 1992, pp. 569-572.
[5] S. A. Zahorian, P. Silsbee, and X. Wang, “Phone classification with segmental features and a binarypair partitioned neural network classifier” proceedings of IEEE International Conference on Acoustics, Speech, and Signal Processing (ICASSP-97), 1997, pp. 1011 -1014.
[6] G. Saha, Sandipan, “A new silence removal and endpoint detection algorithm for speech and speaker recognition applications”, Department of Electronics and Electrical Communication Engineering Indian Institute of Technology, Khragpur, Kharagpur, India.
[7] M. A. Anusuya and S. K. Katti, "Mel frequency discrete wavelet coefficients for kannada speech recognition using PCA" in Proceedings of International Conference on Advances in Computer Science, 2010.
[8] J. O. Berger, “Statistical decision theory and bayesian analysis”, Springer, 1993.
[9] Xuechuan Wang and Douglas O’Shaughnessy, Fellow, IEEE "Environmental independent asr model adaptation / compensation by bayesian parametric representation", IEEE Transactions On Audio, Speech, And Language Processing, VOL. 15, NO. 4, MAY 2007.
[10] J. MacQueen, “Some methods for classification and Analysis of multivariate observations”, Proc. Of Fifth Berkely symposium on Mathematical Statistics and Probability, June 21-July 18,1965 and December 27,pp-281-297, 7th January- 1966.