Tunnel Magnetoresistive Sensors based Current Transducer with Adaptive Blind Source Separation
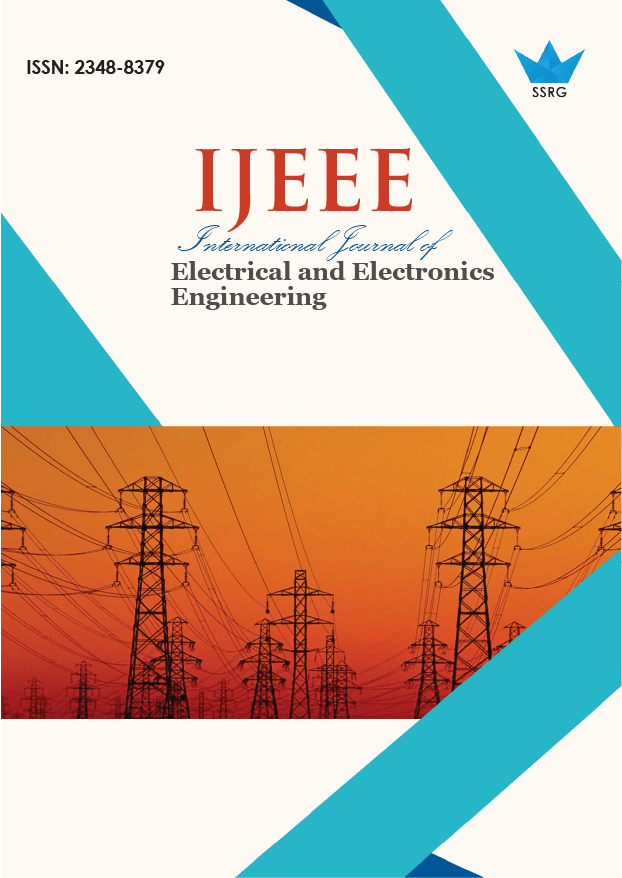
International Journal of Electrical and Electronics Engineering |
© 2017 by SSRG - IJEEE Journal |
Volume 4 Issue 11 |
Year of Publication : 2017 |
Authors : Ayambire Patrick Nyaaba, Qi Huang, Awopone Albert |
How to Cite?
Ayambire Patrick Nyaaba, Qi Huang, Awopone Albert, "Tunnel Magnetoresistive Sensors based Current Transducer with Adaptive Blind Source Separation," SSRG International Journal of Electrical and Electronics Engineering, vol. 4, no. 11, pp. 6-11, 2017. Crossref, https://doi.org/10.14445/23488379/IJEEE-V4I11P102
Abstract:
In this paper, we propose a new approach for designing a high performance and low-cost sensor array based current transducer. This is based on the use of tunnel magnetoresistive sensor array and adaptive blind source separation method. Such a sensor array is able to cancel magnetic interference from nearby current carrying conductors by giving a near perfect estimation and separation of the unknown source signals. Experiment using a hardware prototype based on an analog interface and a DSP for enhancing flexibility was used. The result obtained with the used of Tunnel Magnetoresistive sensor array is presented in this paper.
Keywords:
Adaptive blind source separation, Interference cancellation, Sensor array, Tunnel Magnetoresistive sensor.
References:
[1] R. Weiss, R. Makuch, A. Itzke, and R. Weigel, “Crosstalk in Circular Arrays of Magnetic Sensors for Current Measurement,” IEEE Trans. Ind. Electron., vol. 64, no. 6, pp. 4903–4909, 2017.
[2] F. Fir, F. Iir, T. Fir, I. Transactions, and S. Processing, “8 . Adaptive Filters.”
[3] S. Vorobyov and A. Cichocki, “Blind noise reduction for multisensory signals using ICA and subspace filtering, with application to EEG analysis,” Biol. Cybern., 2002.
[4] J. Schmidhuber, “Deep Learning in neural networks: An overview,” Neural Networks, vol. 61. pp. 85–117, 2015.
[5] Z. Ghahramani, “Unsupervised Learning BT - Advanced Lectures on Machine Learning,” Adv. Lect. Mach. Learn., vol. 3176, no. Chapter 5, pp. 72–112, 2004.
[6] S. Choi, A. Cichocki, H.-M. Park, and S.-Y. Lee, “Blind source separation and independent component analysis: A Review,” Neurocomputing, vol. 6, no. 1, pp. 1–57, 2005.
[7] C. Jutten and M. Babaie-Zadeh, “Source separation: Principles, current advances and applications,” Notes, pp. 1–10, 1997.
[8] J. L. Lacoume, M. Gaeta, and W. Kofman, “Source separation using higher order statistics,” J. Atmos. Terr. Phys., vol. 54, no. 10, pp. 1217–1226, 1992.
[9] S. Fiori, “Entropy optimization by the PFANN network: application to blind source separation.,” Network, vol. 10, no. 2, pp. 171–86, 1999.
[10] S. Amari, A. Cichocki, and H. Yang, “A new learning algorithm for blind signal separation,” Adv. Neural Inf. Process. Syst., vol. 8, pp. 757–763, 1996.
[11] P. Comon, “Independent component analysis, A new concept?,” Signal Processing, vol. 36, no. 3, pp. 287–314, 1994.
[12] S. Amari and A. Cichocki, “Adaptive blind signal processing-neural network approaches,” Proc. IEEE, vol. 86, no. 10, pp. 2026–2048, 1998.
[13] M. H. Chowdhury, “Noise separation in analog integrated circuits using blind source separation,” 2007 IEEE Int. Conf. Electro/Information Technol., pp. 16–20, 2007.
[14] M. Možek, D. Vrtačnik, D. Resnik, U. Aljančič, S. Penič, and S. Amon, “Digital self-learning calibration system for smart sensors,” Sensors Actuators, A Phys., vol. 141, no. 1, pp. 101–108, 2008.
[15] P. NEWMAN, “CONTINUOUS CALIBRATION OF MARINE SEISMIC SOURCES,” Geophys. Prospect., vol. 33, no. 2, pp. 224–232, 1985.
[16] S. C. Douglas, A. Cichocki, and S.-I. Amari, “Multichannel Blind Separation and Deconvolution of Sources with Arbitrary Distributions,” in Proc. IEEE Workshop on Neural Networks for Signal Processing (NNSP’97), 1997, pp. 436–445.
[17] J. F. Cardoso and B. H. Laheld, “Equivariant adaptive source separation,” IEEE Trans. Signal Process., vol. 44, no. 12, pp. 3017–3030, 1996.
[18] N. Murata, M. Kawanabe, A. Ziehe, K. R. Muller, and S. Amari, “On-line learning in changing environments with applications in supervised and unsupervised learning,” Neural Netw, vol. 15, no. 4–6, pp. 743–760, 2002.