A Control Method for Nonlinear Systems Using Combined Sliding Mode Control and RBF Neural Network
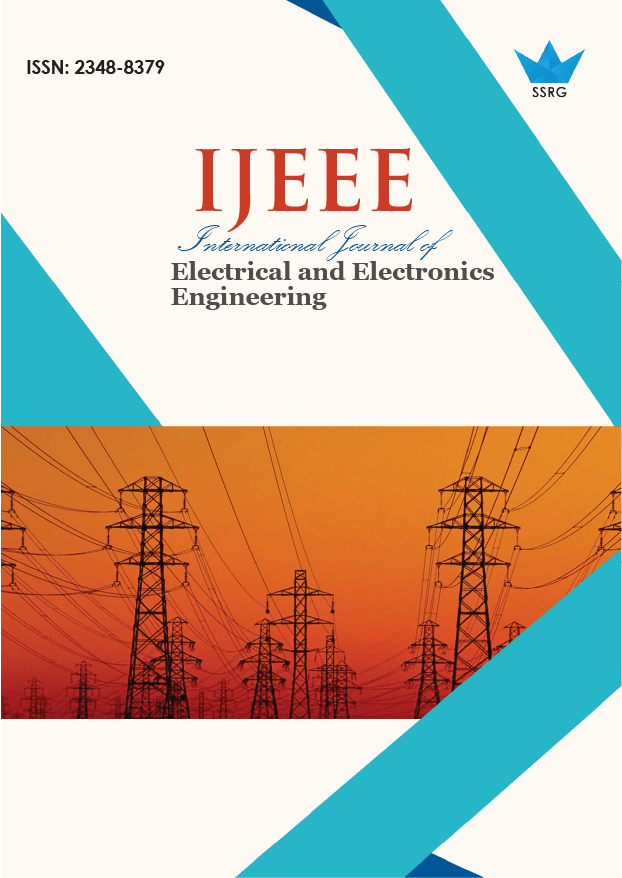
International Journal of Electrical and Electronics Engineering |
© 2018 by SSRG - IJEEE Journal |
Volume 5 Issue 6 |
Year of Publication : 2018 |
Authors : Ngoc Trung Dang and Huyen Linh Le Thi |
How to Cite?
Ngoc Trung Dang and Huyen Linh Le Thi, "A Control Method for Nonlinear Systems Using Combined Sliding Mode Control and RBF Neural Network," SSRG International Journal of Electrical and Electronics Engineering, vol. 5, no. 6, pp. 11-15, 2018. Crossref, https://doi.org/10.14445/23488379/IJEEE-V5I6P103
Abstract:
AIn general, the industrial systems are uncertain nonlinear systems with the influence of external disturbance factors. This paper has developed a control method for nonlinear systems combining Sliding Mode Control with Radial Basic Function (RBF) Neural Network to ensure robustness and interference resistance. This paper has developed a control method for nonlinear systems with external measureless disturbance by combining Sliding Mode Control with RBF Neural Network to ensure robustness and interference resistance. The obtained Sliding Mode Control algorithms and weight update rules of the Network have ensured the existence and stability of the Sliding Mode system. The efficiency and feasibility of the proposed method have verified by simulation results in Matlab-Simulink.
Keywords:
RBF Neural Network, Sliding Mode Control, Lyapunov stability, nonlinear systems, adaptive control.
References:
[1] Doan Phuoc Nguyen, Analysis and control of nonlinear systems, Hanoi Science and Technology Publishing House, 2012.
[2] J. Liu, Radial Basis Function (RBF) Neural Network Control for Mechanical Systems, Springer Venlag, 2013.
[3] J. Q. Gang, and Bin Yao, ―Neural Network Adaptive Rosbust Control of Nonlinear System in semi – strict feedback form,‖ Automatica, no. 37, pp. 1149-1160, 2001.
[4] W. Dong, Y. Zhao, and J. A. Farrell, Neural Networks and Learning system,‖ IEEE Trans. On, Vol. 23, No. 2, pp. 223-235, 2012.
[5] Haykin S, Neural Networks a comprehensive Foundation, Macmillan College Public Co., New York, 1994.
[6] W. S. Lin, and C. S. Chen, ―Robust adaptive Sliding Mode control using fuzzy modelling for a class of uncertain MIMO nonlinear systems,‖ IEEE Proc. Cotrol Theory Application, Vol. 149, No. 3, pp. 193-201, 2002.
[7] Suzana Uran, and Riko Safaric, ―Neural Network estimation of the Variable Plant for Adaptive Sliding Mode Controller,” Mechanical Engineering, no. 58, pp. 93-101, 2012.
[8] S. N. Huang, K.K. Tan, and T. H. Lee, ―A combined PID/ Adaptive Controller for a class of nonlinear systems,‖ Automatica, no. 37, pp. 611-618, 2001.
[9] T. H. Cao, V. Nguyen, T. K. Ngo, T. N. C Ngo, ―A method to synthesis adaptive control system for nonlinear objects under external disturbances,‖ Journal of Military Science Research and Technology, pp. 6-15, no 17/02, 2012.
[10] T. H. L. Le, K. L. Lai, ―An identify method for state-dependence disturbance in time-delay nonlinear system,‖ Control and Automation Specialize, pp. 53-57, no. 11, 2014.
[11] J. Fei, H. Ding, ―Adaptive sliding mode control of dynamic system using RBF Neural Network,‖ Springer Science + Business Media B.V., Nonlinear Dyn 70: pp. 1563–1573, 2012.
[12] H. Zhang, M. Du, and W. Bu, ―Sliding Mode Controller with RBF Neural Network for Manipulator Trajectory Tracking,‖ IAENG International Journal of Applied Mathematics, Advance online publication 45:4, 14 November, 2015.
[13] Y. Tao, J. Zheng, Y. Lin, ―A Sliding Mode Control-based on a RBF Neural Network for Deburring Industry Robotic Systems,‖ International Journal of Advanced Robotic Systems, doi: 10.5772/62002, 2016.
[14] T. N. C Ngo, ―An identify and compensating method for uncertain terms of nonlinear objects,‖ Journal of Military Science Research and Technology, pp. 53-61, no. 50/08, 2017.
[15] Utkin V. I, Sliding Mode in Control Optimazation, Springer – Verlag, Berlin, 1992
[16] Christopher E., and Sarah K., Sliding Model Control Theory and Applications, Taylor & Francis, UK, 1998.