Predicting Water Content Outcomes in Natural Gas Dehydration Systems Using Artificial Intelligence
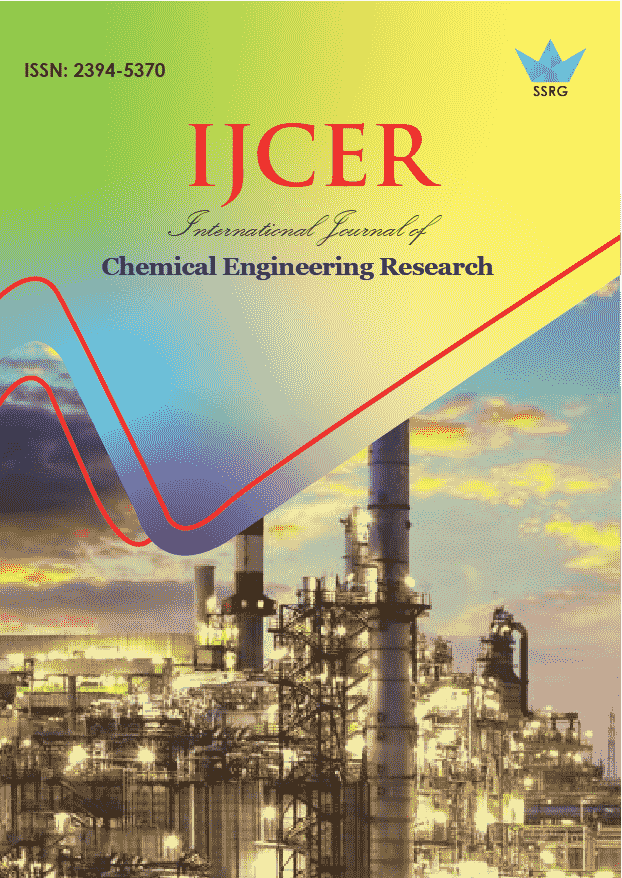
International Journal of Chemical Engineering Research |
© 2021 by SSRG - IJCER Journal |
Volume 8 Issue 1 |
Year of Publication : 2021 |
Authors : Olayemi Kehinde Miracle, Oduola Mujeeb Koyejo |
How to Cite?
Olayemi Kehinde Miracle, Oduola Mujeeb Koyejo, "Predicting Water Content Outcomes in Natural Gas Dehydration Systems Using Artificial Intelligence," SSRG International Journal of Chemical Engineering Research, vol. 8, no. 1, pp. 15-21, 2021. Crossref, https://doi.org/10.14445/23945370/IJCER-V8I1P103
Abstract:
This paper offers a comprehensive evaluation aimed at predicting the water content outcome of natural gas systems using artificial intelligence. In this study, an artificial intelligence model- a three layer artificial neural network model- has been developed using past gas dehydration process data to predict the water content outcomes in natural gas dehydration systems. The water content outcomes are Class 0 which represent data points that meet the water content specification of 7 lb/MMscf or Class 1, which do not. The input features of the model are temperature of the reboiler in ºF, stripping gas flow rate in scf/gal triethylene glycol (TEG), number of equilibrium stages in the contactor, and TEG circulation rate in gal TEG/lb H2O. An exploratory data analysis was carried out on the training data and the optimum process parameters found are TEG recirculation rates between 3.2 and 3.8 gal TEG/lb H20, reboiler temperatures between 380ºF and 400ºF, stripping rate of 0 – 3 scf/gal TEG, and two and three equilibrium stage contactors. The model was evaluated against test data and experimental data from literature and F1 scores of 0.969 and 0.987 were obtained respectively. This showed that the model was able to predict correctly the expected water content outcomes of new gas dehydration data points.
Keywords:
Natural gas dehydration, water content, artificial intelligence, artificial neural network, modelling.
References:
[1] Twu, C. H., Tassone, V., Sim, W. D., & Watanasiri, S., Advanced equation of state method for modeling TEG–water for glycol gas dehydration. Fluid Phase Equil. (2005) 228–229, 213–221. https://doi.org/10.1016/j.fluid.2004.09.031
[2] Amir, H. M., & Dominique, R., Use of artificial neural networks for estimating water content of natural gases. Ind. Eng. Chem. Res, 46(0) (2007) 1431–1438.
[3] McKetta, J. J., & Wehe, A. H., Petroleum refiner (Hydrocarbon Processing), 37(8)(1958) 153.
[4] Elgibaly, A. A., & Elkamel, A. M., A new correlation for predicting hydrate formation conditions for various gas mixtures and inhibitors. Fluid Phase Equilibria, 152(1998) 23–42. https://doi.org/10.1016/S0378-3812(98)00368-9
[5] Psichogios, D. C., & Ungar, L. H., Hybrid neural network-first principles approach to process modeling. AIChE Journal, 38(10)(1992) 1499–1511. https://doi.org/10.1002/aic.690381003
[6] Kingma, D. P., & Ba, J., Adam: A method for stochastic optimization [paper presentation]. International Conference for Learning Representations (ICLR), San Diego, USA. arXiv:1412.6980 (2015).
[7] Venkatasubramanian, V., The promise of artificial intelligence in chemical engineering: Is it here, finally? AIChE Journal, 65(2)(2018) 479–482. https://doi.org/10.1002/aic.16489
[8] Banares-Alcantara, R., Westerberg, A. W., Ko, E. I., & Rychener, M. D., Decade-A hybrid expert system for catalyst selection: Expert system consideration. Comput. Chem. Eng., 11(3)(1987) 265–277. https://doi.org/10.1016/0098-1354(87)85008-1
[9] Banares-Alcantara, R., Westerberg, A. W., Ko, E.I., & Rychener, M. D., Development of an expert system for physical property predictions. Comput. Chem. Eng., 9(2)(1985) 127–142. https://doi.org/10.1016/0098-1354(85)85003-1
[10] Basila JR, M. R., Stefanek, G., & Cinar, A., A model-object based supervisory expert system for fault tolerant chemical reactor control. Comput. Chem. Eng., 14(15)(1990) 551–560. https://doi.org/10.1016/0098-1354(90)87026-L
[11] Takagi T, Sugeno M. Fuzzy identification of systems and its applications to modeling and control. IEEE Transactions on Systems, Man, and Cybernetics. 1985;15:116-132
[12] Kramer, M. A., Malfunction diagnosis using quantitative models with non-boolean reasoning in expert systems. AIChE Journal, 33(1)(1987) 130–140. https://doi.org/10.1002/aic.690330115
[13] Hoskins, J. C., Kaliyur. K. M., & Himmelblau, D. M., Fault diagnosis in complex chemical plants using artificial neural network. AIChE Journal, 37(1)(1991) 137–141. https://doi.org/10.1002/aic.690370112
[14] Hajjar Z, Kazemeini M, Rashidi A, Tayyebi S. Artificial intelligence techniques for modeling and optimization of the HDS process over a new graphene based catalyst. Phosphorus, Sulfur, and Silicon and the Related Elements. (2016) 191:1256-1261
[15] Hajjar Z, Khodadadi A, Mortazavi Y, Tayyebi S, Soltanali S. Artificial intelligence modeling of DME conversion to gasoline and light olefins over modified nano ZSM-5 catalysts.Fuel. (2016)179:79-86
[16] Gibbs MS, Morgan N, Maier HR, Dandy GC, Nixon JB, Holmes M. Investigation into the relationship between chlorine decay and water
distribution parameters using data driven methods. Mathematical and Computer Modelling. (2006) 44:485-498
[17] Tayyebi S, Shahrokhi M, Boozarjomehry RB. Fault diagnosis in a yeast fermentation bioreactor by genetic fuzzy system. Iranian journal of chemistry and chemical engineering.2010;29:61-72
[18] Thompson, M. L., & Kramer, M. A., Modeling chemical processes using prior knowledge and neural networks. AIChE Journal, 40(8) (1994) 1328–1340. https://doi.org/10.1002/aic.690400806
[19] Bakshi, B. R., & Stephanopoulos, G., Wave-Net: A multiresolution, hierarchical neural network with localized learning. AIChE Journal, 39(1)(1993) 57–81. https://doi.org/10.1002/aic.690390108
[20] Katare, S., Caruthers, J. M., Delgass, W. N. & Venkatasubramanian, V., An intelligent system for reaction kinetic modeling and catalyst design. Ind. Eng. Chem. Res., 43(14)(2004) 3484–3512. https://doi.org/10.1021/ie034067h
[21] Sundaram, A., Ghosh, P., Caruthers, J. M., &Venkatasubramanian, V., Design of fuel additives using neural networks and evolutionary algorithms. AIChE Journal, 47(6)(2004) 1387–1406. https: //doi.org/10.1002/aic.690470615
[22] Salaheddine, K., Ghalib, L., Si Ali, B., & Daud, W., Developing an artificial neural network model to predict carbon steel’s corrosion potential in carbonated solution mixture of amines [Paper presentation].CORCON Conference 2015, Chennai, India.,(2016).
[23] Rumelhart, D. E., Hinton, G. E., & Williams, R. J., Learning representations by back-propagating errors. Nature, 323(1986) 533–536. https://doi.org/10.1038/323533a0
[24] LeCun, Y., Bengio, Y., & Hinton, H., Deep learning. Nature, 521(2015), 436–444. https://doi.org/10.1038/nature14539., (2015).
[25] Ahmadi, M. A., & Bahadori, A., Prediction performance of natural gas dehydration units for water removal efficiency using least square support vector machine. International Journal of Ambient Energy, 37(5)(2015) 486–494. https://doi.org/10.1080/01430750.2015.1004105
[26] Ghiasi, M. M., Bahadori, A., & Zendehboudi, S., Estimation of the water content of natural gas dried by solid calcium chloride dehydrator units. Fuel, 117(2014)(2013) 33–42. https://doi.org/10.1016/j.fuel.2013.09.086
[27] Tatar, A., Nasery, S., Bahadori, M., Bahadori, A., Bahadori, M., Barati-Harooni, A., & Najafi-Marghmaleki, A., Prediction of water removal rate in a natural gas dehydration system using radial basis function neural network. Petroleum Science and Technology, 34(10) (2016) 951–960. https://doi.org/10.1080/10916466.2016.1166131
[28] Hernandez-Valencia, V. N., Hlavinka, M. W., & Bullin, J. A., Design glycol units for maximum efficiency [Paper presentation]. Gas Processors Association (GPA) Annual Convention 1992, Oklahoma, USA., (1992).
[29] Arubi, I. M., & Duru, U. I., Optimizing glycol dehydration system for maximum efficiency: A case study of a gas plant in Nigeria. Society of Petroleum Engineers, SPE 113781., (2008);
[30] Berry, M. J., & Linoff, G. S., Data mining techniques: For marketing, sales, and customer support. John Wiley & Sons, Inc., (1997).
[31] He, K., Zhang, X., Ren, S. & Sun, J., Delving deep into rectifiers: Surpassing human-level performance on ImageNet classification. arXiv:1502.01852 [cs.CV]., (2015).
[32] Srinivasan Suresh., Prediction of Average Annual Daily Traffic Using Machine Learning Methods., SSRG International Journal of Computer Science and Engineering 6.11(2019) 51-54.