Sentiment Analysis Based Named Entity Recognition with Tweet Segmentation
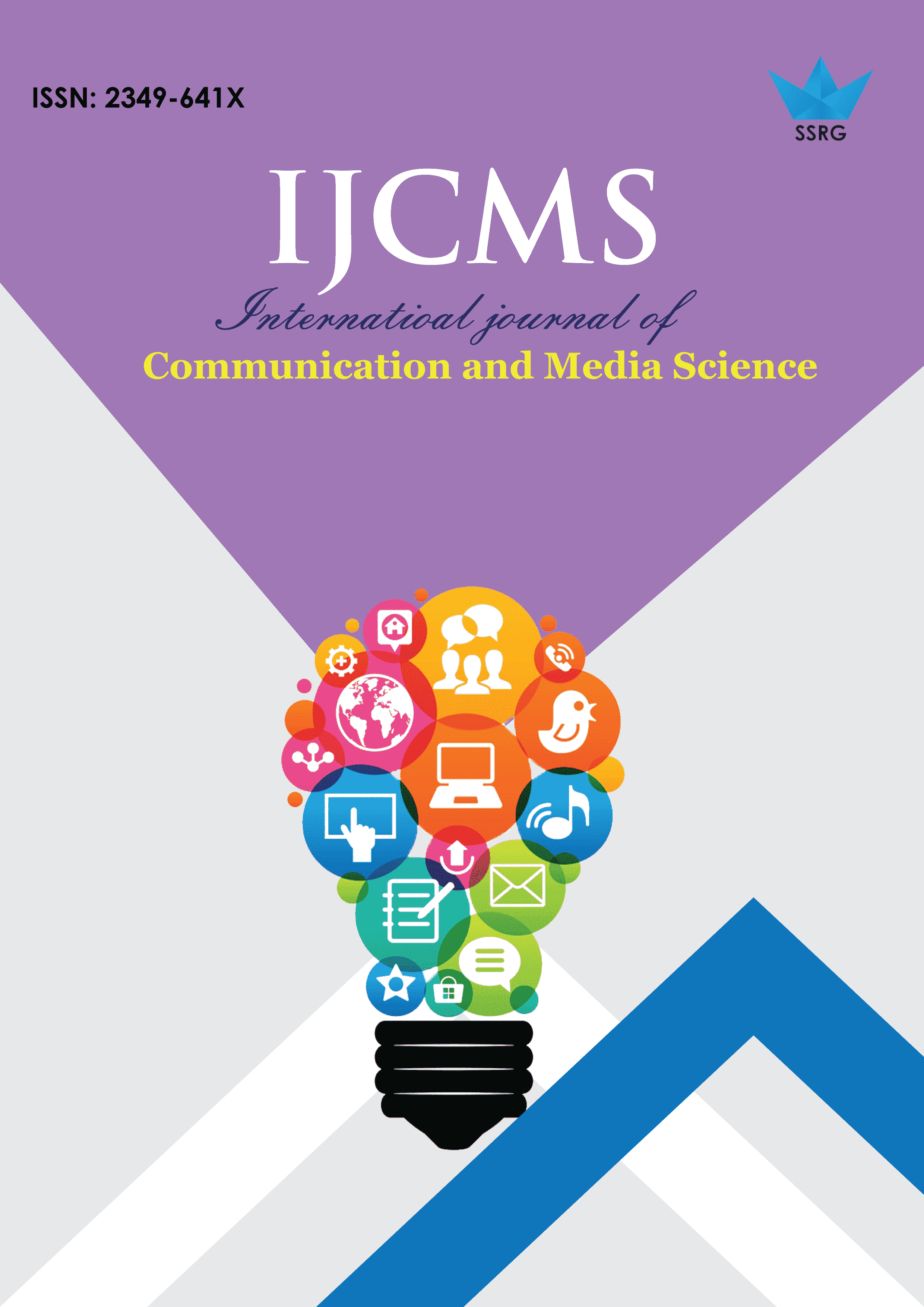
International Journal of Communication and Media Science |
© 2016 by SSRG - IJCMS Journal |
Volume 3 Issue 3 |
Year of Publication : 2016 |
Authors : K.Divya and R.Kiruba Kumari |
How to Cite?
K.Divya and R.Kiruba Kumari, "Sentiment Analysis Based Named Entity Recognition with Tweet Segmentation," SSRG International Journal of Communication and Media Science, vol. 3, no. 3, pp. 10-13, 2016. Crossref, https://doi.org/10.14445/2349641X/IJCMS-V3I5P103
Abstract:
Sentiment analysis or opinion mining is a machine learning approach in which machines examine and characterize the human's assessments, feelings, sentiments and so forth about some theme which are communicated as either content or discourse. The legendary information accessible in the web is increasing day by day. So as to upgrade the offers of an item and to enhance the consumer loyalty, the majority of the on-line shopping destinations give the chance to clients to compose surveys about items. These audits are extensive in number and to mine the general assessment or conclusion extremity from every one of them, opinion mining can be utilized. Manual examination of such vast number of surveys is essentially unthinkable. Accordingly mechanized methodology of a machine has critical part in illuminating this hard issue. The significant test of the region of Sentiment investigation and Opinion mining lies in recognizing the feelings communicated in these writings. In this paper a review is carried out to study the insight examination of the issues in and out and to familiarize with the concepts of sentiment analysis.
Keywords:
Tweet Data, sentiment analysis, opinion mining, classifiers.
References:
[1] S. Lee and J. Kim, ―WarningBird: Detecting Suspicious URLs in Twitter Stream, Proc. 19th Network and Distributed System Security Symp. (NDSS), 2012.
[2] C. Grier, K. Thomas, V. Paxson, and M. Zhang, ―@spam: The Underground on 140 Characters or Les, Proc. 17th ACM Conf. Computer and Comm. Security (CCS), 2010.
[3] C. Yang, R. Harkreader, and G. Gu, ―Die Free or Live Hard? Empirical Evaluation and New Design for Fighting Evolving Twitter Spammers, Proc. 14th Int’l Symp. Recent Advances in Intrusion Detection (RAID), 2011.
[4] C.Y.R. Harkreader, J. Zhang, S. Shin, and G. Gu, ―Analyzing Spammers’ Social Networks for Fun and Profit—a Case Study of Cyber Criminal Ecosystem on Twitter, Proc. 21st Int’l World Wide Web Conf. (WWW), 2012.
[5] F. Benevenuto, G. Magno, T. Rodrigues, and V. Almeida,―Detecting Spammers on Twitter, Proc. Seventh Collaboration,Electronic Messaging, Anti-Abuse and Spam Conf. (CEAS), 2010.
[6] G. Stringhini,C. Kruegel, and G. Vigna, ―Detecting Spammers on Social Networks, Proc. 26th Ann. Computer Security Applications Conf. (ACSAC), 2010.
[7] H. Gao, Y. Chen, K. Lee, D. Palsetia, and A. Choudhary, ―Towards Online Spam Filtering in Social Networks, Proc. 19th Network and Distributed System Security Symp. (NDSS), 2012.
[8] J. Ma, L.K. Saul, S. Savage, and G.M. Voelker, ―Beyond Blacklists: Learning to Detect Malicious Web Sites from Suspicious URLs, Proc. 15th ACM SIGKDD Conf. Knowledge Discovery and Data Mining (KDD), 2009.
[9] J. Ma, L.K. Saul, S. Savage, and G.M. Voelker, ―Identifying Suspicious URLs: An Application of Large-Scale Online Learning,Proc. 26th Int’l Conf. Machine Learning (ICML), 2009.
[10] J. Song, S. Lee, and J. Kim, ―Spam Filtering in Twitter Using Sender-Receiver Relationship, Proc. 14th Int’l Symp. Recent Advances in Intrusion Detection (RAID), 2011
[11] M. Cova, C. Kruegel, and G. Vigna, ―Detection and Analysis of Drive-by-Download Attacks and Malicious JavaScript Code,Proc. 19th Int’l World Wide Web Conf. (WWW), 2010
[12] S. Ghosh, B. Viswanath, F. Kooti, N.K. Sharma, G. Korlam, F.Benevenuto, N. Ganguly, and K.P. Gummadi, ―Understanding and Combating Link Farming in the Twitter Social Network,Proc. 21st Int’l World Wide Web Conf. (WWW), 2012.