A Review on Dynamic Concept Drift
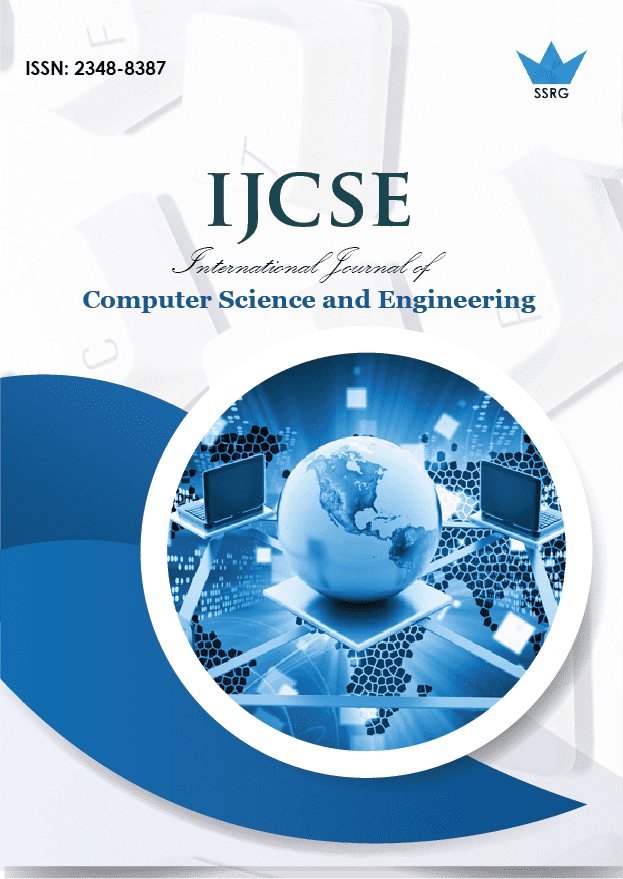
International Journal of Computer Science and Engineering |
© 2016 by SSRG - IJCSE Journal |
Volume 3 Issue 10 |
Year of Publication : 2016 |
Authors : D.Kishore Babu, Dr. Y.Ramadevi, Dr.K.V.Ramana |
How to Cite?
D.Kishore Babu, Dr. Y.Ramadevi, Dr.K.V.Ramana, "A Review on Dynamic Concept Drift," SSRG International Journal of Computer Science and Engineering , vol. 3, no. 10, pp. 41-47, 2016. Crossref, https://doi.org/10.14445/23488387/IJCSE-V3I10P113
Abstract:
In the real world concepts are frequently not stable but change with time. Typical examples of this are weather prediction rules and customers' preferences. The underlying data distribution may change as well. Over and over again these changes make the model built on old data inconsistent with the new data, and regular updating of the model is necessary. This problem, known as concept drift, complicates the task of learning a model from data and requires special approaches, different from commonly used techniques, which treat arriving instances as equally important contributors to the final concept. Dynamic concept drift is one of the techniques which will handle the concept drift effectively by using ensembles. Dynamic concept drift with Naïve base classifier is finest one.
Keywords:
concept drift, ensembles, dynamic integration, dynamic weighted majority
References:
[1].Alexey Tsymbal, Mykola Pechenizkiy, Pádraig Cunningham, Seppo Puuronen,”Handling Local Concept Drift with Dynamic Integration of Classifiers: Domain of Antibiotic Resistance in Nosocomial Infections”, Proceedings of the 19th IEEE Symposium on Computer-Based Medical Systems (CBMS'06) 0-7695-2517- 1/06, 2006 IEEE.
[2].Alexey Tsymbal Department of Computer Science Trinity College Dublin, Ireland, “The problem of concept drift: definitions and related work “, April 29, 2004.TCD-CS-2004-15.
[3].P. M. Goncalves, Silas G.T. de Carvalho Santos, Roberto S.M. Barros, Davi C.L. Vieira, (2014) "Review: A comparative study on concept drift detectors", A International Journal: Expert Systems with Applications, 8144–8156.
[4].M. G. Kelly, D. J. Hand, and N. M. Adams(1999), "The Impact of Changing Populations on Classifier performance", In Proc. of the 5th ACM SIGKDD Int. Conf. on Knowl. Disc. and Dat. Mining (KDD). ACM, 367–371.
[5]. J. Gama, I. Zliobaite, A. Bifet, M. Pechenizkiy, A. Bouchachia(2014), "A Survey on Concept Drift Adaptation ", ACM Computing Surveys, Vol. 46, No. 4, Article 44.
[6]. J. Kolter and M. A. Maloof (2007), "Dynamic Weighted Majority: An Ensemble Method for Drifting Concepts", Journal of Machine Learning Research 8, 2755-2790.
[7]. J. Gama, I. Zliobaite, A. Bifet, M. Pechenizkiy, A. Bouchachia(2014), "A Survey on Concept Drift Adaptation ", ACM Computing Surveys, Vol. 46, No. 4, Article 44.
[8]. S. Delany, P. Cunningham, A. Tsymbal, and L. Coyle. (2005),"A Case-based Technique for Tracking Concept Drift in Spam filtering", Knowledge-Based Sys. 18, 4–5, 187–195
[9].Alexey Tsymbal, Mykola Pechenizkiy, Pádraig Cunningham, Seppo Puuronen,”Handling Local Concept Drift with Dynamic Integration of Classifiers: Domain of Antibiotic Resistance in Nosocomial Infections”, Proceedings of the 19th IEEE Symposium on Computer-Based Medical Systems (CBMS'06) 0-7695-2517- 1/06, 2006 IEEE.
[10].Widmer G., Kubat M., Learning in the presence of concept drift and hidden contexts, Machine Learning, 23 (1), 1996, 69-101.
[11]. Harries M., Sammut C., Horn K., Extracting hidden context, Machine Learning, 32(2),1998, 101-126).
[12].Indre Zliobaite, "Learning under Concept Drift: an Overview". Lithuania: Faculty of Mathematics and Informatics, Vilnius University, Lithuania.
[13].D. Yeung and P. Chan. (2006). MCS Tutorial [Online]. www.ece.stevens-tech.edu/~hhe/cpe695f09/lecturenotes. [14].Mohammed Alshammeri, “Dynamic Committees for Handling Concept Drift in Databases (DCCD)”, School of Electrical Engineering and Computer Science University of Ottawa, Canada 2012.
[15]. Yamini Kadwe, Vaishali Suryawanshi Department of IT, M.I.T. College Of Engineering, Pune, India. “A Review on Concept Drift”. IOSR Journal of Computer Engineering (IOSR-JCE) e-ISSN: 2278-0661, p-ISSN: 2278-8727, Volume 17, Issue 1, Ver. II (Jan – Feb. 2015), PP 20-26.
[16].Alexey Tsymbal, Mykola Pechenizkiy, Pádraig Cunningham, Seppo Puuronen,”Handling Local Concept Drift with Dynamic Integration of Classifiers: Domain of Antibiotic Resistance in Nosocomial Infections”, Proceedings of the 19th IEEE Symposium on Computer-Based Medical Systems (CBMS'06) 0-7695-2517- 1/06, 2006 IEEE.
[17]. W. N. Street and Y. Kim (2001), “A streaming ensemble algorithm (SEA) for large-scale classification,” in Proc. 7th ACM SIGKDD Int. Conf. Knowl. Discovery Data Mining, pp. 377–382.
[18]. A. Bifet, G. Holmes, B. Pfahringer, R. Kirkby, and R. Gavaldà(2009),“New ensemble methods for evolving data streams,” in Proc. 15th ACM SIGKDD Int. Conf. Knowl. Discovery Data Mining, pp. 139-148.
[19]. D Brzezinski, J Stefanowski (2014),"Reacting to Different Types of Concept Drift: The Accuracy Updated Ensemble Algorithm" IEEE Transactions on Neural Networks and Learning Systems,” Vol. 25, pp. 81-94.
[20]. D Brzezinski, J Stefanowski(2014), "Combining block-based and online methods in learning ensembles from concept drifting data streams", An International Journal: Information Sciences 265, 50– 67.
[21].Alexey Tsymbal, Mykola Pechenizkiy, Pádraig Cunningham, Seppo Puuronen,”Dynamic Integration of Classifiers for Handling Concept Drift”, Journal, Information Fusion Volume 9 Issue 1, January, 2008, Pages 56-68, Elsevier Science Publishers.
[22].Alexey Tsymbal, Mykola Pechenizkiy, Pádraig Cunningham, Seppo Puuronen,”Dynamic Integration of Classifiers for Handling Concept Drift.”Journal, Information Fusion Volume 9 Issue 1, January, 2008, Pages 56-68, Elsevier Science Publishers.
[23]. Alexey Tsymbal, Mykola Pechenizkiy, Pádraig Cunningham, Seppo Puuronen,”Dynamic Integration of Classifiers for Handling Concept Drift”, Journal, Information Fusion Volume 9 Issue 1, January, 2008, Pages 56-68, Elsevier Science Publishers.
[24]. Alexey Tsymbal, Mykola Pechenizkiy, Pádraig Cunningham, Seppo Puuronen. “Dynamic Integration of Classifiers for Handling Concept Drift”, Journal, Information Fusion Volume 9 Issue 1, January, 2008, Pages 56-68, Elsevier Science Publishers.
[25]. Alexey Tsymbal1, Mykola Pechenizkiy, Pádraig Cunningham1, Seppo Puuronen. “Dynamic Integration of Classifiers for Tracking Concept Drift in Antibiotic Resistance Data”, Journal, Information Fusion Volume 9 Issue 1, January, 2008, Pages 56-68, Elsevier Science Publishers.
[26]. Alexey Tsymbal, Mykola Pechenizkiy, Pádraig Cunningham, Seppo Puuronen, “Dynamic Integration of Classifiers for Handling Concept Drift”, Journal, Information Fusion Volume 9 Issue 1, January, 2008, Pages 56-68, Elsevier Science Publishers.
[27].Bauer E., Kohavi R. “An empirical comparison of voting classification algorithms: bagging, boosting, and variants”, Machine Learning 36, 105–139 (1999) 1999 Kluwer Academic Publishers. Manufactured in The Netherlands.
[28].Jeremy Z. Kolter and Marcus A.Maloof Department of Computer Science Georgetown University “Dynamic Weighted Majority: A New Ensemble Method for Tracking Concept Drift ”, Proceedings of the Third International IEEE Conference on Data Mining, 123–130.Los Alamitos, CA: IEEE Press.
[29]. J. Zico Kolter, Marcus A. Maloof,”Dynamic weighted majority: an ensemble method for drifting concepts”, Journal of Machine Learning Research 8 (2007) 2755-2790, Third IEEE International Conference on Data Mining, 2003 IEEE.
[30].Leo. Breiman, Statics Department University of California Berkeley, CA94720, “Bagging Predictors,” Machine Learning, vol. 24, pp. 123-140(1996) 1996 Kluwer Academic Publishers, Boston. Manufactured in the Netherlands.
[31].Yoav Freund and Robert E. Schapire, "A decision-theoretic generalization of on-line learning and an application to boosting," Journal of Computer and System Sciences, vol. 55(1), pp 119–139, August 1997
[32].N. Littlestone and M. K. Warmuth, Department of computer science ,University of California, santa Cruz, California 95064,“The weighted majority algorithm,” Information and Computation, vol. 108, pp.212–261, 1994.
[33].Agustín Ortiz Díaz, José del Campo-Ávila, Gonzalo Ramos- Jiménez, Isvani Frías Blanco,Yailé Caballero Mota, Antonio Mustelier Hechavarría, and Rafael Morales-Bueno, “Fast Adapting Ensemble: A New Algorithm for Mining Data Streams with Concept Drift”, Hindawi, Publishing Corporation The Scientific World Journal, Volume 2015, Article ID 235810, 14 pages.
[34]. J. Zico Kolter, Marcus A. Maloof,”Dynamic weighted majority: an ensemble method for drifting concepts”, Journal of Machine Learning Research 8 (2007) 2755-2790, Third IEEE International Conference on Data Mining, 2003 IEEE.
[35].K.Woods,W.P. Kegelmeyer, K. Bowyer, “Combination of multiple classifiers using local accuracy estimates”, IEEE Transaction on PAMI, 19 (4), 1997, 405-410
[36].Alexey Tsymbal, Mykola Pechenizkiy, Pádraig Cunningham, Seppo Puuronen “Dynamic Integration of Classifiers for Tracking Concept Drift in Antibiotic Resistance Data”, Information Fusion Volume 9 Issue 1, January, 2008, Pages 56-68, Elsevier Science Publishers
[37].Bauer E., Kohavi R. “An empirical comparison of voting classification algorithms: bagging, boosting, and variants”, Machine Learning 36, 105–139 (1999) 1999 Kluwer Academic Publishers. Manufactured in The Netherlands.
[38]. Cullen Schaffer “Selecting a classification method by crossvalidation”, Machine Learning, 13, 135-143 (1993) 1993, Kluwer Academic Publishers, Boston. Manufactured in the Netherlands.
[39].G.Giacinto, F. Roli, “Dynamic classifier selection based on multiple classifier behavior,” Pattern Recognition, 34 (9), (2001), 1879-1881. Dept. of Electrical and Electronic Eng, Univ. of Cagliari, Piazza d’Armi, 09123 Cagliari, ITALY.
[40].G.Giacinto, F. Roli, “Methods for dynamic classifier selection,” In: ICIAP '99, 10th International Conference on Image Analysis and Processing, Venice, Italy, IEEE CS Press, September,1999, pp.659- 664.
[41]. Mohammed Alshammeri, “Dynamic Committees for Handling Concept Drift in Databases (DCCD)”, School of Electrical Engineering and Computer Science University of Ottawa, Canada 2012.
[42].Robnik-Sikonja M. “Improving random forests”. In: ECML 2004 J.F. 15th European Conf. on Machine Learning Pisa Italy, September 2004, 2004, pp.359-370.
[43]. Tsymbal A., M. Pechenizkiy, P. Cunningham, “Sequential genetic search for ensemble feature selection.” In: Proc. 19th Int. Joint Conf. on Artificial Intelligence IJCAI’2005, Morgan Kaufmann, August 2005, pp.877-882.
[44]. Tsymbal A., Puuronen S. “Bagging and boosting with dynamic integration of classifiers.” In: PKDD 2000,Principles of Data Mining and Knowledge Discovery, Lyon, France, September 2000, pp.116-125.