Semantic Understanding of Abstract Images
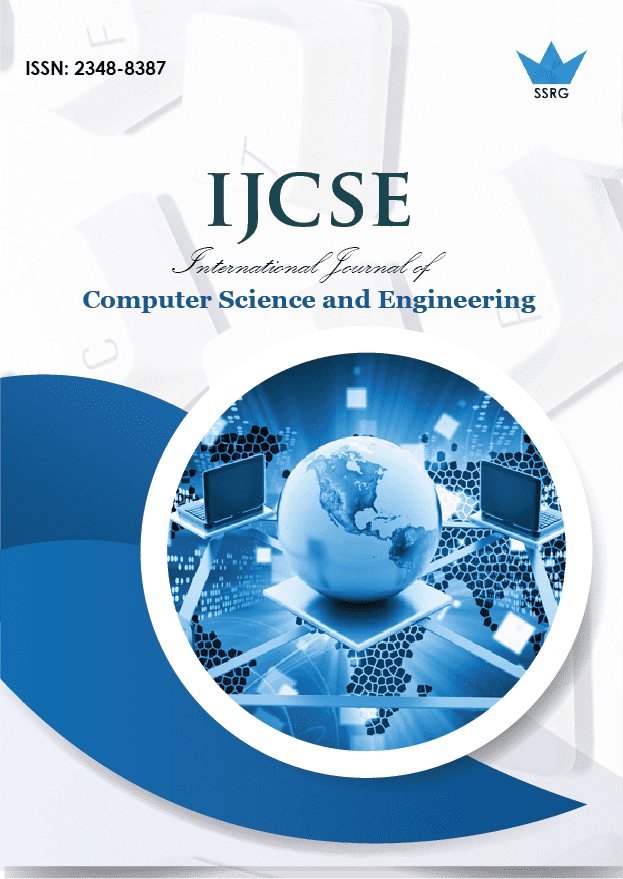
International Journal of Computer Science and Engineering |
© 2017 by SSRG - IJCSE Journal |
Volume 4 Issue 4 |
Year of Publication : 2017 |
Authors : Mrs. G. Vijayalakshmi, Sudipto Biswas, Sankeerth Reddy |
How to Cite?
Mrs. G. Vijayalakshmi, Sudipto Biswas, Sankeerth Reddy, "Semantic Understanding of Abstract Images," SSRG International Journal of Computer Science and Engineering , vol. 4, no. 4, pp. 30-34, 2017. Crossref, https://doi.org/10.14445/23488387/IJCSE-V4I4P107
Abstract:
The relation of visual information to its language-based meaning remains a testing region of research. Semantic significance of pictures relies on upon the nearness of items, ascribes and their relations to different articles. But exactly describing this dependence needs taking out of complex visual data from a picture, that is in normal is an exceptionally troublesome but then unsolved issue. During this paper, we propose learning semantic data in unique pictures made from various pictures. Unique pictures give many points of interest over genuine pictures. They take into account the immediate investigation of how to figure abnormal state data, since they wipe out the dependence on buzzing low-level question, property and connection finders, or the exhausting and depleting handnaming of honest to goodness picture. Fundamentally, conceptual pictures moreover allow the ability to make sets of syntactic near scenes. Finding comparable plans of honest to goodness pictures that are about the same would be almost inconceivable. We make nearly a similar conceptual picture with relating composed depiction. We absolutely intentionally concentrate this dataset to conceive syntactic basic parts, the relations of words to visual components and procedures for measuring semantic comparability. We concentrate the association among the boldness and notability of things and their syntactic criticalness. In this project, we have integrated word-net for analysing all possible synonyms for the keywords given. Hence search efficiency, accuracy shall be improved. we present a viewable-aspect joint hyper graph learning approach to model the relationship of all images. Our aim of the project is to develop a meaning based search engine and increase the search accuracy and relevancy of search data for both images and web URL’s.
Keywords:
Semantic searching of Images; Keyword Images; Image Search.
References:
[1] L. Itti, C. Koch, and E. Niebur, “A model of saliencybased visual attention for rapid scene analysis,” IEEE Trans. Pattern Anal. Mach. Intell., vol. 20, no. 11, pp. 1254–1259, Nov. 1998.
[2] C. Privitera and L. Stark, “Algorithms for defining visual regions- of-interest: Comparison with e y e fixations,” IEEE Trans. Pattern Anal. Mach. Intell., vol. 22, no. 9, pp. 970–982, Sep. 2000.
[3] L. Elazary a n d L . Itti, “Interesting objects are visual ly salient,” J. Vis., vol. 8, no. 3, pp. 1–15, 2008.
[4] S. Hwang and K. Grauman, “Learning the relative impor tance of objects from tagged images for retrieval and cross-modal search.
[5] M. Spain and P. Perona, “Measuring a n d predicting object importance,” Int. J. Comput. Vis., vol. 91, no. 1, pp. 59–76, 2011.
[6] A. Berg, T. Berg, H. Daume, J. Dodge, A. Goyal, X. Han, A. Mensch, M. Mitchell, A. Sood, K. Stratos, and K. Yamaguchi, “Understanding and predicting importance in images,” in Proc. IEEE Conf. Comput. Vis. Pattern Recognit., 2012, pp. 3562–3569.
[7] A. Farhadi, M. Hejrati, M. Sadeghi, P. Young, C. Rashtchian, J. Hockenmaier, and D. Forsyth, “Every picture tells a story: Gen- erating sentences from images,” in Proc. 11th Eur. Conf. Comput. Vis., 2010, pp. 15–29.
[8] V. Ordonez, G. Kulkarni, and T. Berg, “Im2text: Describing images using 1 million captioned photographs,” in Proc. Adv. Neu- ral Inf. Process. Syst., 2011, pp. 1143–1151.
[9] Y. Yang, C. Teo, H. Daum e III, and Y. Alimonies, “Corpus- guided sentence generation of natural images,” in Proc. Conf. Empirical Methods Natural Lang. Process., 2011, pp. 444–454.
[10] G . Kulkarni, V. Premraj, S. Dhar, S . Li, Y. Choi, A. Berg, and T. Berg, “Baby talk: Understanding and generating simple image descriptions,” in Proc. IEEE Conf. Comput. Vis. Pattern Recogni t ., 2011, pp. 1601–1608.
[11] A . Farhadi, I. Endres, D. Hoiem, and D. Forsyth, “Describing objects by their attributes,” in Proc. IEEE Conf. Comput. Vis. Pattern Recognit., 2009, pp. 1778–1785.
[12] T . Berg, A. Berg, and J. Shih, “Automatic attribute discovery and characterization from n o i s y we b d a t a ,” in Proc. 11th Eur. Conf. Comput. Vis., 2010, pp. 663–676.
[13] A . Gupta and L. Davis, “Beyond n o u n s : Exploiting prepositions and comparative adjectives for learning visual classifiers,” in Proc. 10th Eur. Conf. Comput. Vis., 2008, pp. 16–29.
[14] F. Heider and M. Simmel, “An experimental study of apparent behavior,” The Am. J. Psychol., vol. 57, pp. 243– 259, 1944.
[15] K . Oatley and N. Yuill, “Perception of personal and interpersonal action in a cartoon film,” B r i t . J. Soc. Psychol., vol. 24, no. 2, pp. 115–124, 2011.