An Efficient Machine Learning based Algorithm for Preventing Phishing Websites
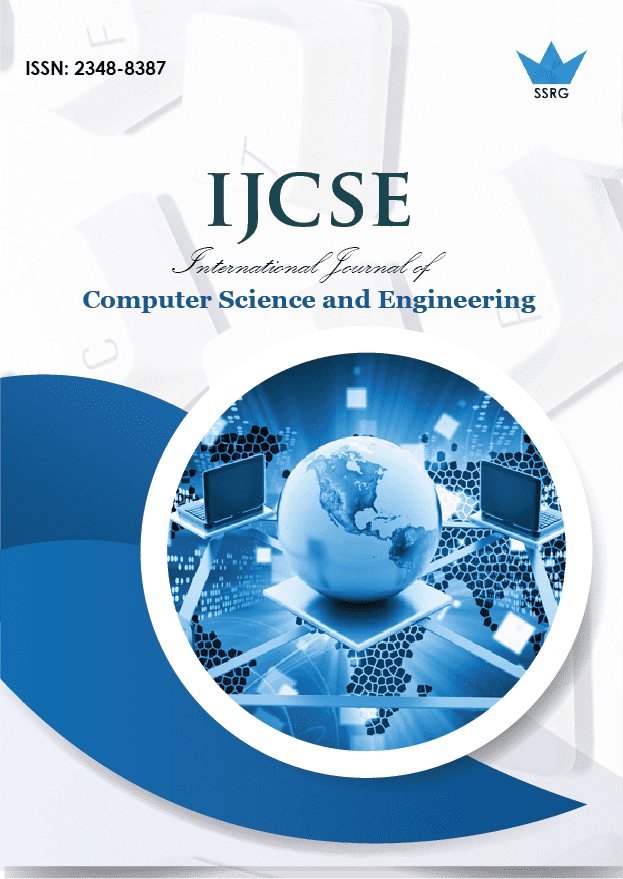
International Journal of Computer Science and Engineering |
© 2018 by SSRG - IJCSE Journal |
Volume 5 Issue 12 |
Year of Publication : 2018 |
Authors : Peravali Kavya |
How to Cite?
Peravali Kavya, "An Efficient Machine Learning based Algorithm for Preventing Phishing Websites," SSRG International Journal of Computer Science and Engineering , vol. 5, no. 12, pp. 10-13, 2018. Crossref, https://doi.org/10.14445/23488387/IJCSE-V5I12P102
Abstract:
Now a day’s Internet technology is growing more pervasive for online technologies. By considering we cannot control the security of the application. Because of this, we can face security threats of the network system that is mostly encountered is Phishing. Phishing is one of the most attacked of web-based which try to reveal some sensitive information. So many people or companies information is attacked by attackers by using these techniques. To prevent phishing damages we can provide the most secure networks or to get awareness of the people. To detect or preventing the phishing attacks we can build strong mechanism before they cause too much damage. In this paper, we are proposed an efficient machine learning based detection schema to detect or prevent the phishing attack. By implementing this technique we can get zero hour phishing attacks and they have superior adaption for new types of phishing attacks, therefore they are mainly preferred.
Keywords:
Phishing Attack, Entropy, Gain, Machine learning, URL, Domain Names.
References:
[1] H.Huang, S. Zhong, J. Tan, “Browser-side countermeasures for deceptive phishing attack,” Fifth International Conference on Information Assurance and Security IAS'09, vol. 1, pp. 352-355, IEEE, 2009.
[2] M.A.U.H.Tahir, S. Asghar, A. Zafar, S. Gillani, “A Hybrid Model to Detect Phishing-Sites Using Supervised LearningAlgorithms,” International Conference on Computational Science and Computational Intelligence (CSCI), pp. 1126-1133, IEEE, 2016.
[3] R.M.Mohammad, F. Thabtah, L. McCluskey, “Predicting phishing websites based on self-structuring neural network,” Neural Computing and Applications, vol. 25(2), pp. 443-458, 2014.
[4] M.He, S.J. Horng, P. Fan, M.K. Khan, R.S. Run, J.L. Lai, R.J. Chen, Sutanto, “An Efficient Phishing Webpage Detector,” Expert Systems with Applications, vol. 38(10), pp. 12018-12027, 2011.
[5] H.H.Nguyen, D. T. “Nguyen, Machine learning based phishing web sites detection,” In AETA 2015: Recent Advances in Electrical Engineering and Related Sciences, pp. 123-131, Springer International Publishing, 2016.
[6] N.Abdelhamid, A. Ayesh, F. Thabtah, “Phishing detection based associative classification data mining,” Expert Systems with Applications, vol. 41(13), pp. 5948-5959, 2014.
[7] R.M.Mohammad, F. Thabtah, L. McCluskey, “Tutorial and critical analysis of phishing websites methods,” Computer Science Review, vol. 17, pp. 1-24, 2015.
[8] S.Nawafleh, W. Hadi (2012). Multi-class associative classification to predicting phishing websites. International Journal of Academic Research Part A; 2012;4(6), 302-306J. Clerk Maxwell, A Treatise on Electricity and Magnetism, 3rd ed., vol. 2. Oxford: Clarendon, 1892, pp.68–73.
[9] Sadeh N, Tomasic A, Fette I. Learning to detect phishing emails. Proceedings of the 16th international conference on World Wide Web. 2007: p. 649-656.
[10] Andr Bergholz, Gerhard Paa, Frank Reichartz, Siehyun Strobel, and Schlo Birlinghoven. Improved phishing detection using model-based features. In Fifth Conference on Email and Anti-Spam, CEAS, 2008
[11] S.Sheng, B. Wardman, G. Warner, L. F. Cranor, J. Hong, and C. Zhang, “An empirical analysis of phishing blacklists,” in Proceedings of the 6th Conference in Email and Anti-Spam, ser. CEAS’09, Mountain view, CA, July 2009.
[12] P.Prakash, M. Kumar, R. R. Kompella, and M. Gupta, “Phishnet: predictive blacklisting to detect phishing attacks,” in INFOCOM’10: Proceedings of the 29th conference on Information communications. Piscataway, NJ, USA: IEEE Press, 2010, pp. 346–350.