RSF: Roughset Theory Based Fuzzy Classification in Randomized Dimensionality Feature Selection
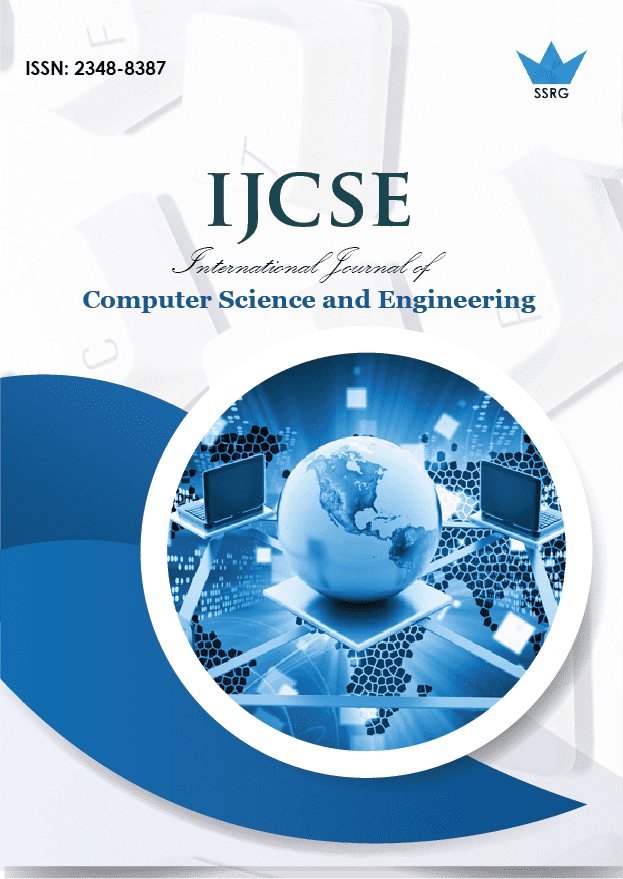
International Journal of Computer Science and Engineering |
© 2019 by SSRG - IJCSE Journal |
Volume 6 Issue 4 |
Year of Publication : 2019 |
Authors : D. Barathi |
How to Cite?
D. Barathi, "RSF: Roughset Theory Based Fuzzy Classification in Randomized Dimensionality Feature Selection," SSRG International Journal of Computer Science and Engineering , vol. 6, no. 4, pp. 25-28, 2019. Crossref, https://doi.org/10.14445/23488387/IJCSE-V6I4P106
Abstract:
Feature Selection is a pattern dimensionality using feature mining and feature selection fit in to the data mining. To improve the robustness of the feature selection algorithm and for visualization point the dimension reduction techniques may be analyzed. The randomized feature selection is the transformation of high-dimensional data into animportantdesign of reduced dimensionality that corresponds to the fundamental dimensionality of the data. The normal reduction algorithm often not well for large datasets and fault dimensionality reduction, hence, to enhance the efficiency, the proposed system apply Roughset theory based fuzzy classification on original data set and obtain a reduced dataset containing possibly uncorrelated variables. In this paper, Roughset theory for feature selection and fuzzy based classification for Feature selection non-linear conversion is used for reduce the dimensionality and primary crisp value is calculated, then it is applied to classification algorithm.
Keywords:
Rough set, Fuzzy, preprocessing.
References:
[1] D. Aha and D. Kibler, “Instance-based learning algorithms,” Mach. Learn., vol. 6, no. 1, pp. 37–66, 1991.
[2] F. Alonso-Atienza, J. L. Rojo-Alvare, A. Rosado-Mu~noz, J. J. Vinagre, A. Garcia-Alberola, and G. Camps-Valls, “Feature selection using support vector machines and bootstrap methods for ventricular fibrillation detection,” Expert Syst. Appl., vol. 39, no. 2, pp. 1956–1967, 2012.
[3] D. Dernoncourt, B. Hanczar, and J. D. Zucker, “Analysis of feature selection stability on high dimension and small sample data,” Comput. Statist. Data Anal., vol. 71, pp. 681–693, 2014.
[4] J. Fan and Y. Fan, “High dimensional classification using features annealed independence rules,” Ann. Statist., vol. 36, no. 6, pp. 2605–2637, 2008.
[5] A. J. Ferreira and M. A. T. Figueiredo, “Efficient feature selection filters for high dimensional data,” Pattern Recog. Lett., vol. 33, no. 13, pp. 1794–1804, 2012.
[6] Y. Han and L. Yu, “A variance reduction framework for stable feature selection,” Statist. Anal. Data Mining, vol. 5, no. 5, pp. 428–445, 2012.
[7] J. Hua, W. D. Tembe, and E. R. Dougherty, “Performance of feature-selection methods in the classification of high-dimension data,” Pattern Recognit., vol. 42, no. 3, pp. 409–424, 2009.
[8] L. Yu and H. Liu, “Efficient feature selection via analysis of relevance and redundancy,” The J. Mach. Learn. Res., vol. 5, no. 2, pp. 1205–1224, 2004.