Leveraging Optical Character Recognition Technology for Enhanced Anti-Money Laundering (AML) Compliance
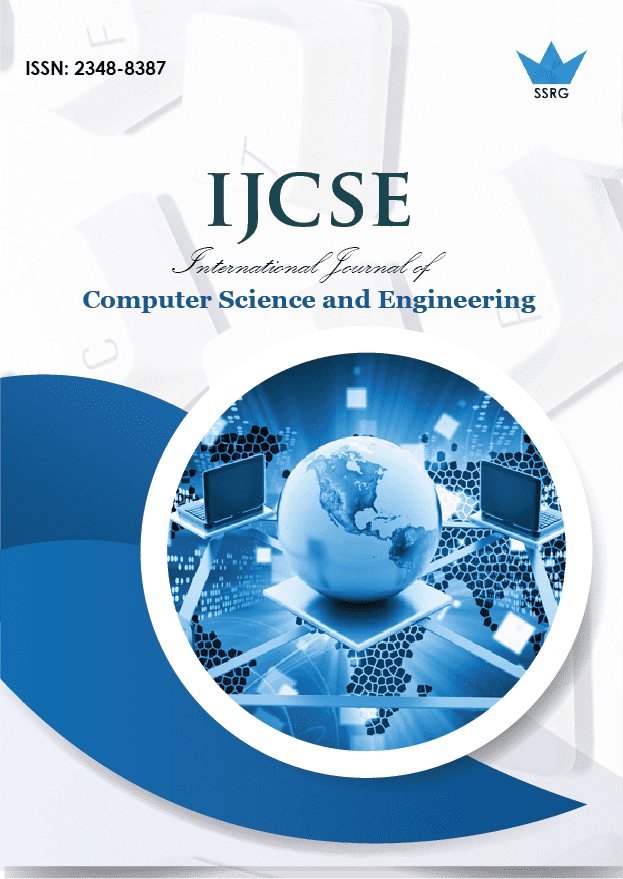
International Journal of Computer Science and Engineering |
© 2023 by SSRG - IJCSE Journal |
Volume 10 Issue 5 |
Year of Publication : 2023 |
Authors : Saikiran Subbagari |
How to Cite?
Saikiran Subbagari, "Leveraging Optical Character Recognition Technology for Enhanced Anti-Money Laundering (AML) Compliance," SSRG International Journal of Computer Science and Engineering , vol. 10, no. 5, pp. 1-7, 2023. Crossref, https://doi.org/10.14445/23488387/IJCSE-V10I5P102
Abstract:
The surge of financial crimes, such as money laundering and terrorist financing, has led to increased regulatory oversight and compliance obligations for financial institutions. Money laundering involves concealing the origins of unlawful funds and presenting them as legitimate. Anti-Money Laundering (AML) regulations aim to prevent the exploitation of financial systems for money laundering purposes. Optical Character Recognition (OCR) technology, combined with AI and machine learning, offers significant benefits in enhancing AML processes. OCR can automate data extraction from documents and help financial institutions identify and report suspicious transactions. This paper explores the use of OCR in AML, discussing various OCR techniques and their advantages and limitations. It also highlights how OCR improves accuracy in customer data screening and addresses challenges in implementing OCR-based AML systems. Additionally, it emphasizes the importance of adapting OCR systems to changing AML regulations. The integration of OCR with other AML technologies and the future trends in AI and machine learning advancements are also discussed. Overall, OCR technology plays a crucial role in automating AML processes, improving accuracy, and enhancing compliance in the fight against money laundering. Staying informed about regulatory changes and adopting advancements in OCR technology is essential for financial institutions to effectively combat emerging risks and protect against financial crime.
Keywords:
Compliance, Financial Crimes, Anti-Money Laundering, Machine Learning, Fraud Detection, Optical Character Recognition.
References:
[1] Zippia, 20 Money Laundering Statistics, Facts about Money Laundering in the U.S., 2023. Zippia.com. [Online]. Available: https://www.zippia.com/advice/money-laundering-statistics/
[2] M. Chui, V. Kamalnath, and B. McCarthy, The Transformative Power of Automation in Banking, McKinsey & Company, 2021. [Online]. Available: https://www.mckinsey.com/industries/financial-services/our-insights/the-transformative-power-of-automation-in-banking
[3] Financial Action Task Force, About FATF, 2021. [Online]. Available: https://www.fatf-gafi.org/about/
[4] U.S. Department of the Treasury. (n.d.). The Bank Secrecy Act. [Online]. Available: https://home.treasury.gov/policy-issues/financial-sanctions/faqs/312
[5] U.S. Department of the Treasury. (n.d.). The USA PATRIOT Act. [Online]. Available: https://home.treasury.gov/policy-issues/financial-sanctions/faqs/312
[6] D. H. Shepard, “Optical Character Recognition: A Status Report,” Proceedings of the IEEE, vol. 53, no. 10, pp. 1194-1204, 1965.
[7] Ray Kurzweil, The Age of Intelligent Machines, MIT Press, 1990.
[Google Scholar] [Publisher Link]
[8] S. Mori, C.Y. Suen, and K. Yamamoto, “Historical Review of OCR Research and Development,” Proceedings of the IEEE, vol. 80, no. 7, pp. 1029-1058, 1992.
[CrossRef] [Google Scholar] [Publisher Link]
[9] R. Smith, “An Overview of the Tesseract OCR Engine,” Proceedings of the Ninth International Conference on Document Analysis and Recognition, vol. 2, pp. 629-633, 2007.
[CrossRef] [Google Scholar] [Publisher Link]
[10] Kartik Dutta et al., "Offline Handwriting Recognition on Devanagari Using a New Benchmark Dataset," 13th IAPR International Workshop on Document Analysis Systems, Vienna, Austria, pp. 25-30, 2018.
[CrossRef] [Google Scholar] [Publisher Link]
[11] Nitin Ramesh, Aksha Srivastava, and K Deeba, “Improving Optical Character Recognition Techniques,” International Journal of Engineering & Technology, vol. 7, no. 2.24, 2018.
[CrossRef] [Google Scholar] [Publisher Link]
[12] Bank Secrecy Act. [Online]. Available: https://www.occ.treas.gov/topics/supervision-and-examination/bsa/index-bsa.html
[13] Five Pillars of AML Compliance. [Online]. Available: https://withpersona.com/blog/five-pillars-aml-compliance
[14] Beyond the Five Pillars: Taking a Holistic Approach to AML. [Online]. Available: https://www.acamstoday.org/beyond-the-five-pillars-taking-a-holistic-approach-to-aml/
[15] What is OCR?. https://aws.amazon.com/what-is/ocr/
[16] A. Ratha, and P. K. Behera, “Role of Artificial Intelligence in Anti-Money Laundering,” Advances in Intelligent Systems and Computing, Springer, vol. 1245, pp. 441-447, 2021.
[17] Avinash Malladhi, "Transforming Information Extraction: AI and Machine Learning in Optical Character Recognition Systems and Applications Across Industries," International Journal of Computer Trends and Technology, vol. 71, no. 4, pp. 81-90, 2023.
[CrossRef] [Publisher Link]
[18] J. J. Hull, and M. R. Segal, “OCR History: From Mechanical Aids to Electronic Brains,” Proceedings of the IEEE, vol. 83, no. 11, pp. 1512-1529, 1995.
[19] Noman Islam, Zeeshan Islam, and Nazia Noor, “A Survey on Optical Character Recognition Technology,” Journal of Information & Communication Technology, 2016.
[CrossRef] [Google Scholar] [Publisher Link]
[20] V. K. Govindan, and S. P. Mohanty, “A Review on Optical Character Recognition Techniques,” International Journal of Computer Science and Information Security, vol. 13, no. 3, pp. 20-26, 2015.
[21] Sachin Kumar, H.S. Bhadauria, and Annapurna Singh, “A Review of Character Recognition and Text Detection: Neural-Fuzzy Approach,” Journal of Basic and Applied Engineering Research, vol. 1, no. 7, pp. 61-64, 2014.
[Publisher Link]
[22] R. J. Linn, “OCR in the Financial Industry,” OCR Technology for Data Interpretation, Springer, Cham, pp. 117-127, 2017.
[23] M. Gupta, and D. Kohli, “Artificial Intelligence Techniques in Anti-Money Laundering (AML) Compliance,” Artificial Intelligence for Enhanced Business Analytics, Springer, pp. 307-326, 2020.
[24] T. Khatibi, and M. M. Deris, “A Study on the Advancement of Anti-Money Laundering (AML) Techniques,” International Conference on Information Technology and Digital Applications, IEEE, pp. 1-6, 2020.
[25] V. Agarwal, and F. Elayan, “Anti-Money Laundering Compliance using Intelligent Document Processing,” IEEE 3rd International Conference on Computing, Mathematics and Engineering Technologies, IEEE, pp. 1-5, 2021.