A Deep Learning Approach for Enhanced Power Management using Artificial Intelligence
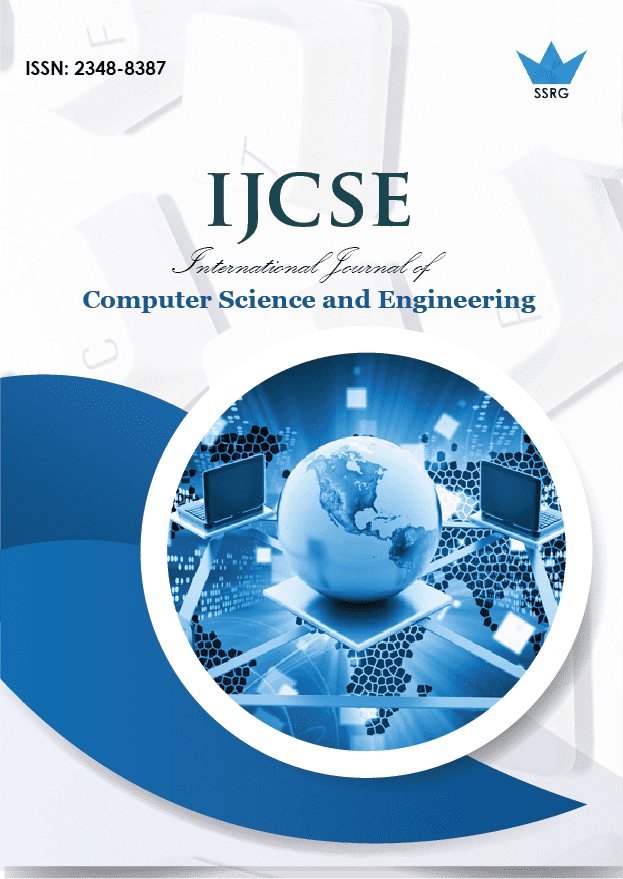
International Journal of Computer Science and Engineering |
© 2023 by SSRG - IJCSE Journal |
Volume 10 Issue 5 |
Year of Publication : 2023 |
Authors : Dikko Elisha Sylvanus, Shehu Mohammed Ahmed, Bamanga Mahmud Ahmad, Adepetun Oluwaseun Ibukun |
How to Cite?
Dikko Elisha Sylvanus, Shehu Mohammed Ahmed, Bamanga Mahmud Ahmad, Adepetun Oluwaseun Ibukun, "A Deep Learning Approach for Enhanced Power Management using Artificial Intelligence," SSRG International Journal of Computer Science and Engineering , vol. 10, no. 5, pp. 26-32, 2023. Crossref, https://doi.org/10.14445/23488387/IJCSE-V10I5P104
Abstract:
Electricity is one of the most beneficial and widely utilized modern innovations. It is the foundation upon which practically everything in our homes and enterprises runs. Electricity moves from point "A" to point "B," implying a supply source and a receiver. Several issues may emerge when electricity travels from the source to the receiving end, resulting in permanent and costly damage to appliances and dwellings. Over the years, there has always been a persistent struggle in Nigeria, often driven by generation and transmission issues. As a result, the researcher is continuously confronted with complete power outages, low or high voltage, and irregular power supply. To mitigate these negative impacts, many devices such as stabilizers and Automatic Voltage Regulators (AVRs) have been developed, although these have been found to fail most of the time, resulting in damage to the home and industrial appliances. This research is not being conducted to provide a dependable power supply. The unfortunate reality is that we will have to live with this for the foreseeable future in Nigeria. This research focuses on reducing the stress associated with constantly changing power supplies by developing a real-time system that can alert the power supply user, constantly monitor the voltage of the power supplied, and alert the user of the action taken as a result of a surge/spike or irregularity in the power supplied via the internet. It basically means that the user will be notified whenever and wherever there are power outages.
Keywords:
Microcontroller, Voltage, Appliance, Electricity, and Safety.
References:
[1] Jingang Wang et al., “Research on Transmission Line Voltage Measurement Method of D-Dot Sensor Based on Gaussian Integral,” Sensors, vol. 18, no. 8, p. 2455, 2018.
[CrossRef] [Google Scholar] [Publisher Link]
[2] Kumar Chandrasekaran, Prabaakaran Kandasamy, and Srividhyan Ramanathan, “Deep Learning and Reinforcement Learning Approach on Microgrid,” International Transactions on Electrical Energy Systems, vol. 30, no. 10, p. e12531, 2020.
[CrossRef] [Google Scholar] [Publisher Link]
[3] K. Shyam Sunder Reddy et al., “Power Management using AI-based IOT Systems,” Measurement: Sensors, vol. 24, p. 100551, 2022.
[CrossRef] [Google Scholar] [Publisher Link]
[4] Ivan Mutis, Abhijeet Ambekar, and Virat Joshi, “Real-time Space Occupancy Sensing and Human Motion Analysis using Deep Learning for Indoor Air Quality Control,” Automation in Construction, vol. 116, p. 103237, 2020.
[CrossRef] [Google Scholar] [Publisher Link]
[5] Paige Wenbin Tien et al., “Machine Learning and Deep Learning Methods for Enhancing Building Energy Efficiency and Indoor Environmental Quality - A Review,” Energy and AI, vol. 10, p. 100198, 2022.
[CrossRef] [Google Scholar] [Publisher Link]
[6] Qin Xin et al., “A Deep Learning Architecture for Power Management in Smart Cities,” Energy Reports, vol. 8, pp. 1568-1577, 2022.
[CrossRef] [Google Scholar] [Publisher Link]
[7] Sherali Zeadally et al., “Design Architectures for Energy Harvesting in the Internet of Things,” Renewable and Sustainable Energy Reviews, vol. 128, p. 109901, 2020.
[CrossRef] [Google Scholar] [Publisher Link]
[8] Gowri Suryanarayana et al., “Thermal Load Forecasting in District Heating Networks using Deep Learning and Advanced Feature Selection Methods,” Energy, vol. 157, pp. 141-149, 2018.
[CrossRef] [Google Scholar] [Publisher Link]
[9] Nouman Ashraf et al., “Energy Management in Harvesting Enabled Sensing Nodes: Prediction and Control,” Journal of Network and Computer Applications, vol. 132, pp. 104-117, 2019.
[CrossRef] [Google Scholar] [Publisher Link]
[10] Jaehyun Ahn et al., “Indoor Air Quality Analysis Using Deep Learning with Sensor Data,” Sensors, vol. 17, no. 11, p. 2476, 2017.
[CrossRef] [Google Scholar] [Publisher Link]
[11] Changhai Peng, and Jinfu Huang, “A Home Energy Monitoring and Control System based on ZigBee Technology,” International Journal of Green Energy, vol. 13, no. 15, pp. 1615-1623, 2016.
[CrossRef] [Google Scholar] [Publisher Link]
[12] Ersan Kabalcı, Yasin Kabalcı, and Pierluigi Siano, “Design and Implementation of a Smart Metering Infrastructure for Low Voltage Microgrids,” International Journal of Electrical Power & Energy Systems, vol. 134, p. 107375, 2022.
[CrossRef] [Google Scholar] [Publisher Link]
[13] Shinya Yoshizawa, and Yasuhiro Hayashi, “Advanced Voltage Control based on Short-time Ahead Voltage Fluctuation Estimation in Distribution System,” Electric Power Systems Research, vol. 188, p. 106559, 2020.
https://doi.org/10.1016/j.epsr.2020.106559
[CrossRef] [Google Scholar] [Publisher Link]
[14] Cheng Fan et al., “Analytical Investigation of Autoencoder-based Methods for Unsupervised Anomaly Detection in Building Energy Data,” Applied Energy, vol. 211, pp. 1123-1135, 2018.
[CrossRef] [Google Scholar] [Publisher Link]
[15] Wali Ullah Khan et al., “Efficient Power Allocation for NOMA-enabled IoT Networks in 6G Era,” Physical Communication, vol. 39, p. 101043, 2020.
[CrossRef] [Google Scholar] [Publisher Link]
[16] S. Supraja, and P. Ranjith Kumar, “An Intelligent Traffic Signal Detection System Using Deep Learning,” SSRG International Journal of VLSI & Signal Processing, vol. 8, no. 1, pp. 5-9, 2021.
[CrossRef] [Publisher Link]
[17] Taofeek Olanrewaju Adebobola, Adewole Oyewale Adetunmbi, and Olaoluwa Omoniyi, “Cost Challenges Facing Nigerian Manufacturing Industries Using Generating Sets as Main Source of Power Supply,” ABUAD Journal of Engineering Research and Development, vol. 6, no. 1, pp. 22-30, 2023.
[CrossRef] [Publisher Link]
[18] Jaime Ortegon-Aguilar et al., “Multimodal Power Management Based on Decision Tree for Internet of Wearable Things Systems,” Applied Sciences, vol. 13, no. 7, p. 4351, 2023.
[CrossRef] [Google Scholar] [Publisher Link]
[19] V.V. Narendra Kumar, and T. Satish Kumar, “Smarter Artificial Intelligence with Deep Learning,” SSRG International Journal of Computer Science and Engineering, vol. 5, no. 6, pp. 10-16, 2018.
[CrossRef] [Google Scholar] [Publisher Link]
[20] Edet Okon Anwana, and Boniface Akpan, “Power Sector Reforms and Electricity Supply Growth in Nigeria,” Asian Journal of Economics and Empirical Research, vol. 3, no. 1, pp. 94–102, 2016.
[CrossRef] [Google Scholar] [Publisher Link]
[21] Cheng Fan et al., “Discovering Gradual Patterns in Building Operations for Improving Building Energy Efficiency,” Applied Energy, vol. 224, pp. 116-123, 2018.
[CrossRef] [Google Scholar] [Publisher Link]
[22] Praveen Kumar Reddy Maddikunta et al., “Green Communication in IoT Networks using a Hybrid Optimization Algorithm,” Computer Communications, vol. 159, pp. 97-107, 2020.
[CrossRef] [Google Scholar] [Publisher Link]