Machine Learning-Based Practical Social-Sensor Provision for Psychological Well-Being Intensive Care Consuming Twitter Data
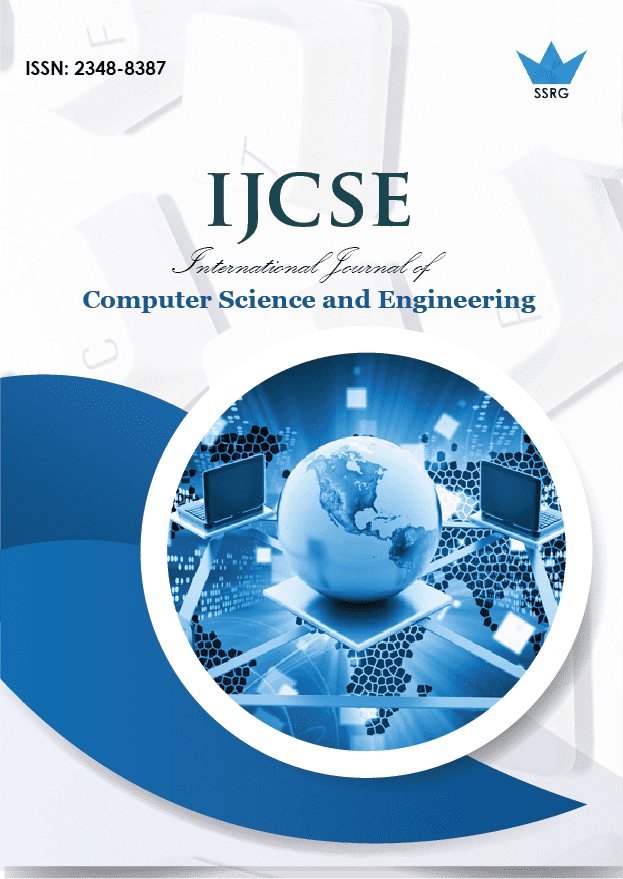
International Journal of Computer Science and Engineering |
© 2023 by SSRG - IJCSE Journal |
Volume 10 Issue 3 |
Year of Publication : 2023 |
Authors : S. Amutha |
How to Cite?
S. Amutha, "Machine Learning-Based Practical Social-Sensor Provision for Psychological Well-Being Intensive Care Consuming Twitter Data," SSRG International Journal of Computer Science and Engineering , vol. 10, no. 3, pp. 1-10, 2023. Crossref, https://doi.org/10.14445/23488387/IJCSE-V10I3P101
Abstract:
Respectively, using social media platforms remains viewed as a cloud of public sensors inside the social sensor ecosystem that makes up social media platforms. To overcome the restrictions of conventional health administration systems used for large-scale mental health surveillance. Although the current methods in the literature offer online mental disease screening, they are challenging to use in early detection. This study develops a general framework to facilitate proactive mental health monitoring, focusing on the Twitter platform. To achieve reliable results in sentiment analysis, comprehensive data spring scrubbing and preprocessing of the tweets are provided by consuming unvarying terms founded on experiential patterns. To circumvent the shortcomings of conventional classifiers, a machine learning apparatus, particularly LSTM, remains utilized for the initial discovery of at-risk public devices grounded on unique occasion explanations. Experimental results show that the suggested mechanism works better for accurate prediction than the current methods.
Keywords:
Machine learning, Sentiment analysis, Large-scale mental health surveillance, Data, Social media
References:
[1] Min Chen et al., “Negative Information Measurement At Ai Edge: A New Perspective For Mental Health Monitoring," ACM Transactions on Internet Technology, vol. 22, no. 3, pp. 1-16, 2020.
[CrossRef] [Google Scholar] [Publisher Link]
[2] P. Marquez, and S. Dutta, "Mental Health," Expert Systems, 2017.
[3] G David Batty, "Psychological Distress in Relation to Site Specific Cancer Mortality: Pooling of Unpublished Data from 16 Prospective Cohort Studies," British Medical Journal, 2017.
[CrossRef] [Google Scholar] [Publisher Link]
[4] Shaoxiong Ji et al., "Suicidal Ideation Detection: A Review of Machine Learning Methods and Applications," IEEE Transactions on Computational Social Systems, vol. 8, no. 1, pp. 214-226, 2020.
[CrossRef] [Google Scholar] [Publisher Link]
[5] J. Zhu et al., "First-Onset Mental Disorders after Cancer Diagnosis and Cancer-Specific Mortality: A Nationwide Cohort Study," Annals of Oncology, vol. 28, no. 8, pp. 1964-1969, 2017.
[CrossRef] [Google Scholar] [Publisher Link]
[6] Aparna Sharma, Kavish Sanghvi, and Prathamesh Churi, "The Impact of Instagram on Young Adult's Social Comparison, Colorism, and Mental Health: Indian Perspective," International Journal of Information Management Data Insights, vol. 2, no. 1, 2022.
[CrossRef] [Google Scholar] [Publisher Link]
[7] Surendra Kumar Reddy Koduru, "Prediction of Severity of an Accident Based on the Extent of Injury using Machine Learning," International Journal of Computer Trends and Technology, vol. 70, no. 9, pp. 43-49, 2022.
[CrossRef] [Publisher Link]
[8] I. Twitter, Wikimedia Foundation.
[9] Nick Drydakis, "Mobile Applications Aim to Facilitate Immigrants' Societal Integration and Overall Level of Integration, Health, And Mental Health, Does Artificial Intelligence Enhance Outcomes?," Computers in Human Behavior, vol. 117, 2021.
[CrossRef] [Google Scholar] [Publisher Link]
[10] Andreas Holzinger et al., "Causability and Explainability of Artificial Intelligence in Medicine," Wiley Interdisciplinary Reviews: Data Mining and Knowledge Discovery, vol. 9, no. 4, 2019.
[CrossRef] [Publisher Link]
[11] Jina Kim et al., "A Deep Learning Model for Detecting Mental Illness from User Content on Social Media," Scientific Reports, 2020.
[CrossRef] [Google Scholar] [Publisher Link]
[12] Shakti Chourasiya, and Suvrat Jain, "A Study Review on Supervised Machine Learning Algorithms," SSRG International Journal of Computer Science and Engineering, vol. 6, no. 8, pp. 16-20, 2019.
[CrossRef] [Google Scholar] [Publisher Link]
[13] Ana Valdivia et al., "Consensus Vote Models for Detecting and Filtering Neutrality in Sentiment Analysis," Information Fusion, vol. 44, pp. 126-135, 2018.
[CrossRef] [Google Scholar] [Publisher Link]
[14] Steven Loria, Textblob: Simplified Text Processing.
[Google Scholar]
[15] Zhao Jianqiang, and Gui Xiaolin, "Comparison Research On Text Pre-Processing Methods on Twitter Sentiment Analysis," IEEE Access, vol. 5, pp. 2870-2879, 2017.
[CrossRef] [Google Scholar] [Publisher Link]
[16] R.Anil Kumar, Shaik Zakir Ahamed, and S.Syed Basha, "A Survey on Machine Learning," International Journal of P2P Network Trends and Technology, vol. 9, no. 1, pp. 9-12, 2019.
[CrossRef] [Publisher Link]
[17] Chetan Harichandra Mendhe et al., "A Scalable Platform to Collect, Store, Visualize, and Analyze Big Data In Real-Time," IEEE Transactions on Computational Social Systems, vol. 8, no. 1, pp. 260-269, 2021.
[CrossRef] [Google Scholar] [Publisher Link]
[18] Zhiwei Guo et al., "Deep Learning-Embedded Social Internet of Things for Ambiguity-Aware Social Recommendations," IEEE Transactions on Network Science and Engineering, vol. 9, no. 3, pp. 1067-1081, 2022.
[CrossRef] [Google Scholar] [Publisher Link]
[19] Satyanarayan Raju Vadapalli, "Monitoring the Performance of Machine Learning Models in Production," International Journal of Computer Trends and Technology, vol. 70, no. 9, pp. 38-42, 2022.
[CrossRef] [Publisher Link]
[20] Shih-Chia Huang et al., "Big Data Analytics and Business Intelligence in Industry," Information Systems Frontiers, vol. 19, pp. 1229-1232, 2017.
[CrossRef] [Google Scholar] [Publisher Link]
[21] Ashwin Sanjay Neogi et al., "Sentiment Analysis and Classification of Indian Farmers' Protest Using Twitter Data," International Journal of Information Management Data Insights, vol. 1, no. 2, 2021.
[CrossRef] [Google Scholar] [Publisher Link]
[22] Leonard Bickman, "Improving Mental Health Services: A 50-Year Journey From Randomized Experiments to Artificial Intelligence and Precision Mental Health," Administration and Policy in Mental Health and Mental Health Services Research, vol. 47, no. 5, pp. 795-843, 2020.
[CrossRef] [Google Scholar] [Publisher Link]
[23] Preetha S, and Musqan Arshad, "Survey on Plants Disease Detection Using Machine Learning," International Journal of Recent Engineering Science, vol. 7, no. 2, pp. 27-29, 2020.
[CrossRef] [Publisher Link]
[24] Graham, S., Depp, C., Lee, E. E., Nebeker, C., Tu, X., Kim, H.-C., & Jeste, D. V, "Artificial Intelligence for Mental Health and Mental Illnesses: An Overview," Current Psychiatry Report, vol. 21, no. 11, p. 116, 2019.
[CrossRef] [Google Scholar] [Publisher Link]
[25] E. Garcia-Ceja et al., "Mental Health Monitoring with Multimodal Sensing and Machine Learning: A Survey,” Pervasive and Mobile Computing, vol. 51, pp. 1-26, 2018.
[CrossRef] [Google Scholar] [Publisher Link]
[26] Satyaki Banerjee, and Nuzhat F. Shaikh, “A Survey on Mental Health Monitoring System via Social Media Data Using Deep Learning Framework,” Techno-Societal, pp. 879-887, 2021.
[CrossRef] [Google Scholar] [Publisher Link]
[27] Dhivya Karmegam, Thilagavathi Ramamoorthy, and Bagavandas Mappillairajan, “A Systematic Review of Techniques Employed for Determining Mental Health Using Social Media in Psychological Surveillance During Disasters,” Disaster Medicine and Public Health Preparedness, vol. 14, no. 2, pp. 265-272, 2020.
[CrossRef] [Google Scholar] [Publisher Link]