Conducting Sentiment Analysis on Twitter Tweets to Predict the Outcomes of the Upcoming Karnataka State Elections
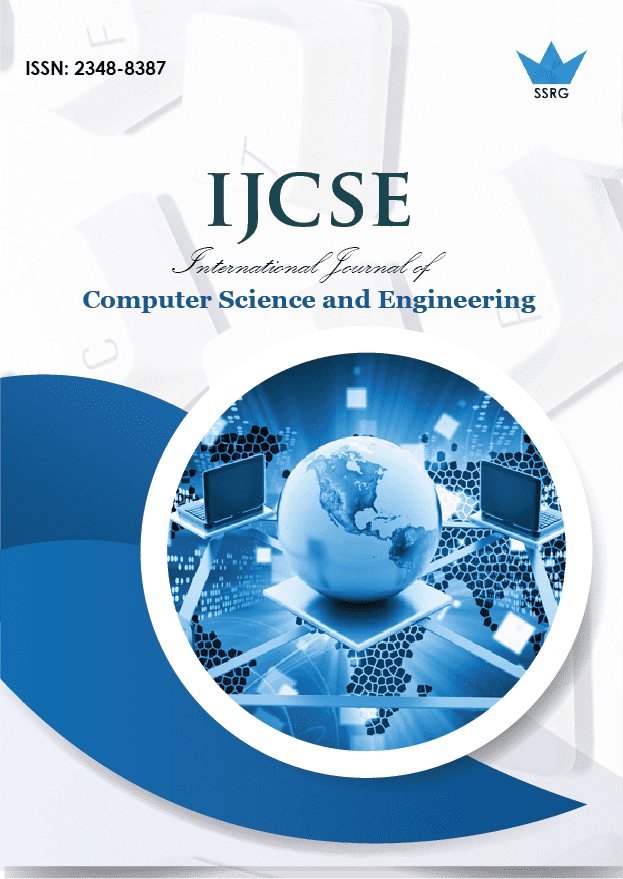
International Journal of Computer Science and Engineering |
© 2023 by SSRG - IJCSE Journal |
Volume 10 Issue 6 |
Year of Publication : 2023 |
Authors : Prajwal Madhusudhana Reddy |
How to Cite?
Prajwal Madhusudhana Reddy, "Conducting Sentiment Analysis on Twitter Tweets to Predict the Outcomes of the Upcoming Karnataka State Elections," SSRG International Journal of Computer Science and Engineering , vol. 10, no. 6, pp. 22-35, 2023. Crossref, https://doi.org/10.14445/23488387/IJCSE-V10I6P104
Abstract:
This research paper aims to predict how Twitter tweets from a specific politician correlate to their winning a seat in a state election. To understand this effect, sentiment analysis has been conducted on tweets by politicians in Karnataka to help predict who will win in the upcoming 2023 Karnataka Legislative Assembly election. Though previous research has already been done in this area, most studies have only focussed on the sentiment analysis of tweets. This paper goes further as it also looks at other factors, including the number of retweets and comments a tweet garners, which measures the tweet's engagement. A model has been created that weighs each factor to help predict who will win an election for a particular constituency. Through this model, a 72.7% accuracy has been achieved. However, the Twitter API severely limited the quantity and quality of data collected. These results can be expanded to help predict elections for other states. They could potentially help understand the effect of positive and negative sentiment on the winnability of a political candidate.
Keywords:
Election, Karnataka, Sentiment analysis, Twint, Twitter.
References:
[1] Apoorv Agarwal et al., “Sentiment Analysis of Twitter Data,” Proceedings of the Workshop on Language in Social Media, pp. 30- 38, 2011.
[Google Scholar] [Publisher Link]
[2] Adam Bermingham, and Alan F. Smeaton, “On Using Twitter to Monitor Political Sentiment and Predict Election Results,” ACL Anthology, 2011.
[Google Scholar] [Publisher Link]
[3] Anastasia Giachanou, and Fabio Crestani, “Like It or Not: A Survey of Twitter Sentiment Analysis Methods,” ACM Computing Surveys, vol. 49, no. 2, pp. 1–41, 2016.
[CrossRef] [Google Scholar] [Publisher Link]
[4] Bhumika Gupta et al., “Study of Twitter Sentiment Analysis Using Machine Learning Algorithms on Python,” International Journal of Computer Applications, vol. 165, no. 9, pp. 29–34, 2017.
[CrossRef] [Google Scholar] [Publisher Link]
[5] Bhumika Pahwa, S. Taruna, and Neeti Kasliwal, “Sentiment Analysis- Strategy for Text Pre-Processing,” International Journal of Computer Applications, vol. 180, no. 34, pp. 15–18, 2018.
[CrossRef] [Google Scholar] [Publisher Link]
[6] Daniel Gayo-Avello, Panagiotis Metaxas, and Eni Mustafaraj, “Limits of Electoral Predictions Using Twitter,” Proceedings of the International AAAI Conference on Web and Social Media, vol. 5, no. 1, pp. 490–493, 2021.
[CrossRef] [Google Scholar] [Publisher Link]
[7] Francisca Onaolapo Oladipo, Ogunsanya Funmilayo Blessing, and Ezendu Ariwa, "Terrorism Detection Model using Naive Bayes Classifier," SSRG International Journal of Computer Science and Engineering, vol. 7, no. 12, pp. 9-15, 2020.
[CrossRef] [Publisher Link]
[8] Luca Buccoliero et al., “Twitter and Politics: Evidence from the US Presidential Elections 2016,” Journal of Marketing Communications, vol. 26, no. 1, pp. 88–114, 2020.
[CrossRef] [Google Scholar] [Publisher Link]
[9] Malhar Anjaria, and Ram Mohana Reddy Guddeti, “A Novel Sentiment Analysis of Social Networks Using Supervised Learning,” Social Network Analysis and Mining, vol. 4, 2014.
[CrossRef] [Google Scholar] [Publisher Link]
[10] Payal Khurana Batra et al., “Election Result Prediction using Twitter Sentiments Analysis,” Sixth International Conference on Parallel, Distributed and Grid Computing (PDGC), pp. 182-185, 2020.
[CrossRef] [Google Scholar] [Publisher Link]
[11] Saifuddin Ahmed, Jaeho Cho, and Kokil Jaidka, “Leveling the Playing Field: The Use of Twitter by Politicians during the 2014 Indian General Election Campaign,” Telematics and Informatics, vol. 34, no. 7, pp. 1377–1386, 2017.
[CrossRef] [Google Scholar] [Publisher Link]
[12] S. Rajeswari et al., "Aspect Based Polarity Extraction in Tamil Tweets using Tree-Based Recursive Partitioning Techniques," International Journal of Engineering Trends and Technology, vol. 70, no. 12, pp. 421-430, 2022.
[CrossRef] [Publisher Link]
[13] V. Rohini, M. Thomas, and C. A. Latha, “Domain Based Sentiment Analysis in Regional Language-Kannada using Machine Learning Algorithm,” IEEE International Conference on Recent Trends in Electronics, Information & Communication Technology, pp. 503-507, 2016.
[CrossRef] [Google Scholar] [Publisher Link]
[14] Yelena Mejova, Sentiment Analysis: An Overview, 2009.
[Google Scholar] [Publisher Link]
[15] A Go, L Huang, and R Bhayani, Twitter Sentiment Analysis, Entropy, 2009.
[Google Scholar]
[16] Farha Nausheen, and Sayyada Hajera Begum, “Sentiment Analysis to Predict Election Results using Python,” 2nd International Conference on Inventive Systems and Control, pp. 1259-1262, 2018.
[CrossRef] [Google Scholar] [Publisher Link]
[17] Vishal A. Kharde, and Sheetal Sonawane, “Sentiment Analysis of Twitter Data: A Survey of Techniques,” International Journal of Computer Applications, vol. 139, no. 11, pp. 5–15, 2016.
[CrossRef] [Google Scholar] [Publisher Link]