Speech to Image Conversion
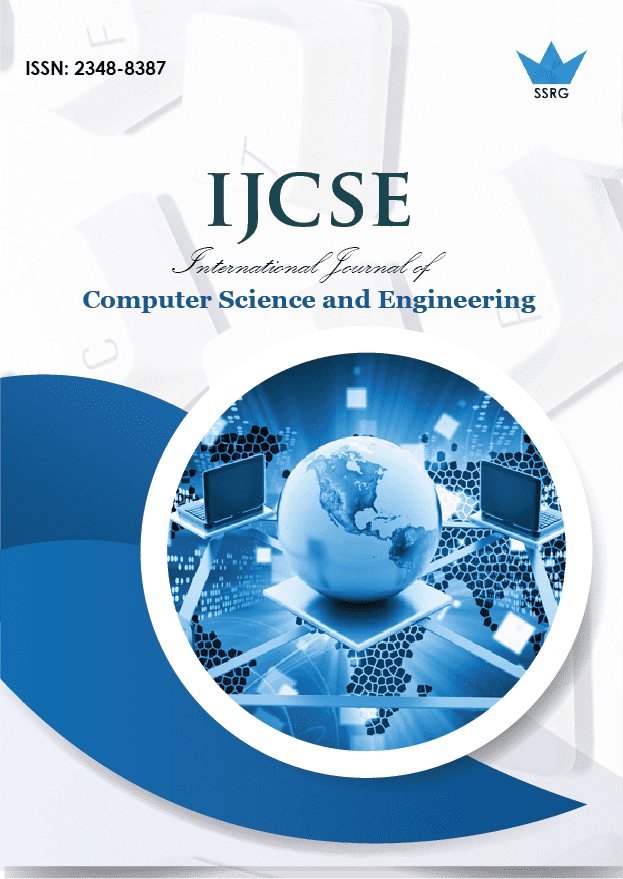
International Journal of Computer Science and Engineering |
© 2023 by SSRG - IJCSE Journal |
Volume 10 Issue 10 |
Year of Publication : 2023 |
Authors : Shaik Karishma, Siddu Devi Naga Susmitha, Nanditha Katari, G. Sirisha |
How to Cite?
Shaik Karishma, Siddu Devi Naga Susmitha, Nanditha Katari, G. Sirisha, "Speech to Image Conversion," SSRG International Journal of Computer Science and Engineering , vol. 10, no. 10, pp. 1-5, 2023. Crossref, https://doi.org/10.14445/23488387/IJCSE-V10I10P101
Abstract:
Translating spoken language into corresponding visual representations is complex and multifaceted. It begins with a systematic analysis of the spoken language, from which necessary elements are extracted and then translated into visually appealing representations that make sense. This thorough approach broadens our comprehension and gives us the tools to communicate complex concepts in a way that is more engaging and intuitive. We are delving deeply into the inner workings of this advanced technology, closely analyzing its intricate mechanisms, investigating its valuable applications in various fields, and discovering the plethora of fascinating opportunities it presents for promoting creativity and more efficient forms of communication as part of our continuous investigation.
Keywords:
Speech, Image, OpenAI, SpeechRecognition, Base64.
References:
[1] S. Morishima, and H. Harashima, “Speech-to-Image Media Conversion based on VQ and Neural Network,” In Acoustics, Speech, and Signal Processing, IEEE International Conference on IEEE Computer Society, pp. 2865-2866, 1991.
[CrossRef] [Google Scholar] [Publisher Link]
[2] H. Yang, S. Chen, and R. Jiang, “Deep Learning-Based Speech-to-Image Conversion for Science Course,” In INTED2021 Proceedings, pp. 2910-2917, 2021.
[CrossRef] [Google Scholar] [Publisher Link]
[3] Jiguo Li et al., “Direct Speech-to-Image Translation,” IEEE Journal of Selected Topics in Signal Processing, vol. 14, no. 3, pp. 517-529, 2020.
[CrossRef] [Google Scholar] [Publisher Link]
[4] Stanislav Frolov et al., “Adversarial Text-to-Image Synthesis: A Review,” Neural Networks, vol. 144, pp. 187-209, 2021.
[CrossRef] [Google Scholar] [Publisher Link]
[5] Xinsheng Wang et al., “Generating Images from Spoken Descriptions,” IEEE/ACM Transactions on Audio, Speech, and Language Processing, vol. 29, pp. 850-865, 2021.
[CrossRef] [Google Scholar] [Publisher Link]
[6] Lakshmi Prasanna Yeluri et al., “Automated Voice-to-Image Generation Using Generative Adversarial Networks in Machine Learning,” In E3S Web of Conferences, 15th International Conference on Materials Processing and Characterization (ICMPC 2023), vol. 430, 2023.
[CrossRef] [Google Scholar] [Publisher Link]
[7] Uday Kamath, John Liu, and James Whitaker, Deep learning for NLP and Speech Recognition, Springer Nature Switzerland, 2019.
[CrossRef] [Google Scholar] [Publisher Link]
[8] Santosh K. Gaikwad, Bharti W. Gawali, and Pravin Yannawar, “A Review on Speech Recognition Technique,” International Journal of Computer Applications, vol. 10, no. 3, pp. 16-24, 2010.
[CrossRef] [Google Scholar] [Publisher Link]
[9] Dong Yu, and Li Deng, Automatic Speech Recognition, A Deep Learning Approach, Springer-Verlag London, 2015.
[CrossRef] [Google Scholar] [Publisher Link]
[10] M. Halle, and K. Stevens, “Speech Recognition: A Model and a Program for Research,” In IRE Transactions on Information Theory, vol. 8, no. 2, pp. 155-159, 1962.
[CrossRef] [Google Scholar] [Publisher Link]