Dental Diseases Segmentation
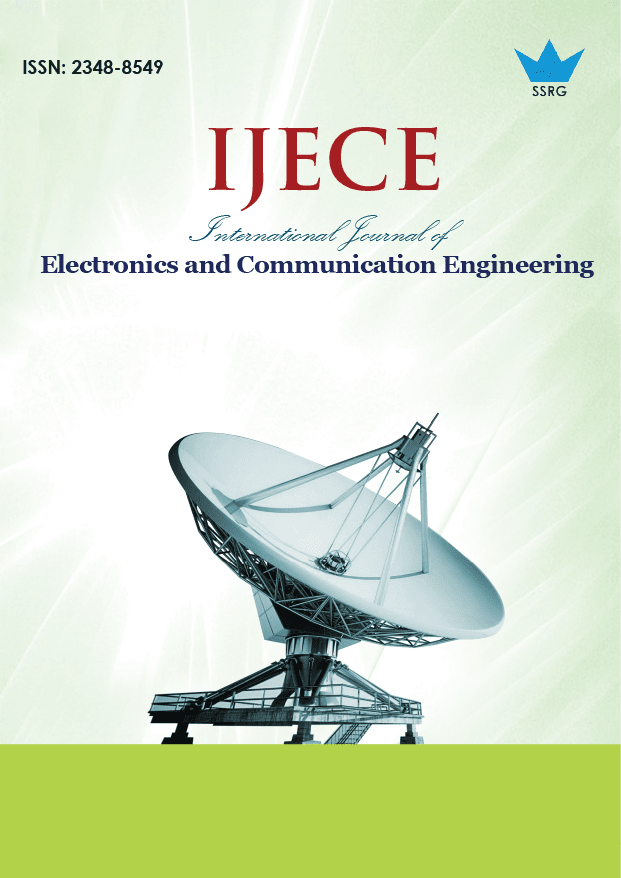
International Journal of Electronics and Communication Engineering |
© 2022 by SSRG - IJECE Journal |
Volume 9 Issue 2 |
Year of Publication : 2022 |
Authors : S. Hemalatha, R. Karthika Devi |
How to Cite?
S. Hemalatha, R. Karthika Devi, "Dental Diseases Segmentation," SSRG International Journal of Electronics and Communication Engineering, vol. 9, no. 2, pp. 1-5, 2022. Crossref, https://doi.org/10.14445/23488549/IJECE-V9I2P101
Abstract:
In recent times, dental care detection has been much needed for society. The dental care prediction is performed with innovative automation using Deep learning (DL) techniques. The earlier prediction is the main problem in the dental field that also leads to heavy illness of health. To overcome these issues, segmentation is to be performed effectively. Therefore, this paper presents the DL-based Butterfly-net model to perform an efficient dental care prediction. This butterfly Net algorithm is based on Deep Convolutional Neural Network (DCNN) that is used to classify dental images. This butterfly net algorithm can be evaluated as a dental degree of membership. The proposed work is compared with the previous work where the Butterfly-net has the highest classification accuracy among all.
Keywords:
Dental care, DCNN, Butterfly net, Classification, Prediction.
References:
[1] Wil G.M.Geraets, and Paul F.Van der Stelt, “Analysis of the Radiographic Trabecular Pattern,” Pattern Recognition Letters, vol. 12, no. 9, pp. 575-581, 1991. Crossref, https://doi.org/10.1016/0167-8655(91)90168-L
[2] Elizabeth B. Claus et al., “Dental X-Rays and Risk of Meningioma,” Cancer, vol. 118, no. 18, pp. 4530-4537, 2012. Crossref, https://doi.org/10.1002/cncr.26625
[3] W T Longstreth Jr et al., “Dental X-Rays and the Risk of Intracranial Meningioma: A Population-Based Case-Control Study,” Cancer, vol. 100, no. 5, pp. 1026-1034, 2004. Crossref, https://doi.org/10.1002/cncr.20036
[4] Wil G. M. Geraets et al., “A New Method for Automatic Recognition of the Radiographic Trabecular Pattern,” Journal of Bone Mineral and Research, vol. 5, no. 3, pp. 227-233, 1990. Crossref, https://doi.org/10.1002/jbmr.5650050305
[5] Wil G. M. Geraets et al., “Detecting Bone Loss Along with Dental Implants by Subtraction of Panoramic Radiographs,” Clinical Oral Implants Research, vol. 23, no. 7, pp. 861-865, 2012. Crossref, https://doi.org/10.1111/j.1600-0501.2011.02215.x
[6] “A Grand Challenge for Automated Detection of Anatomical Landmarks and Analysis for Diagnosis in Cephalometric X-ray Image,” 2015. [Online]. Available: http://wwwo. ntust.edu.tw/~cweiwang/ISBI2015
[7] Abdolvahab Ehsani Rad et al., “Digital Dental X-Ray Database for Caries Screening,” 3D Research, vol. 7, 2016. Crossref, https://doi.org/10.1007/s13319-016-0096-5
[8] Mahmoud Al-Ayyoub, Ismail Heidi, and Haya Rababah, “Detecting Hand Bone Fractures in X-Ray Images,” Journal of Multimedia Processing and Technologies, vol. 4, no. 3, pp. 155-168, 2013.
[9] Weng-Kong Tam, and Hsi-Jian Lee, “Improving Tooth Outline Detection by Active Appearance Model with IntensityDiversification in Intraoral Radiographs,” Journal of Information Science and Engineering, vol. 32, pp. 643-659, 2016.
[10] Sudhakar T et al., “Automatic Detection and Classification of Brain Tumor using Image Processing Techniques,” Research Journal of Pharmacy and Technology, vol. 10, no. 11, pp. 3692-3696, 2017. Crossref, https://doi.org/10.5958/0974-360X.2017.00669.2
[11] Abdolvahab Ehsani Rad et al., “Evaluation of Current Dental Radiographs Segmentation Approaches in Computer-Aided Applications,” IETE Technical Review, vol. 30, no. 3, pp. 210-222, 2013. Crossref, https://doi.org/10.4103/0256-4602.113498
[12] R BalaSubramanyam et al., “Different Image Segmentation Techniques for Dental Image Extraction,” International Journal of Engineering Research and Applications, vol. 4, no. 7, pp. 173-177, 2014.
[13] Y. H. Lai, and P. L. Lin, “Effective Segmentation for Dental X-Ray Images Using Texture Based Fuzzy Inference System,” ACIVS LNCS Springer Verlag Berlin Heidelberg, pp. 936-948, 2008. Crossref, https://doi.org/10.1007/978-3-540-88458-3_85
[14] Abdolvahab Ehsani Rad et al., “Digital Dental X-Ray Image Segmentation and Feature Extraction,” Telkomnika, vol. 11, no. 6, pp. 3109-3114, 2013. Crossref, https://doi.org/10.11591/telkomnika.v11i6.2655
[15] Bethanney Janney J, and S. Emalda Roslin, “Classification of Melanoma from Dermoscopic Data Using Machine Learning Techniques,” Multimedia Tools and Applications, vol. 79, pp. 3713-3728, 2020. Crossref, https://doi.org/10.1007/s11042-018-6927-z
[16] Sukumar Ponnusamy, and Ramasamy Kannan Gnanamurthy, “Computer-Aided Detection of Cervical Cancer Using Pap Smear Images Based on Adaptive Neuro-Fuzzy Inference System Classifier,” Journal of Medical Imaging and Health Informatics, vol. 6, no. 2, pp. 312-319, 2016. Crossref, https://doi.org/10.1166/jmihi.2016.1690