A Deep Learning Based Methodological Analysis for Breast Cancer Classification
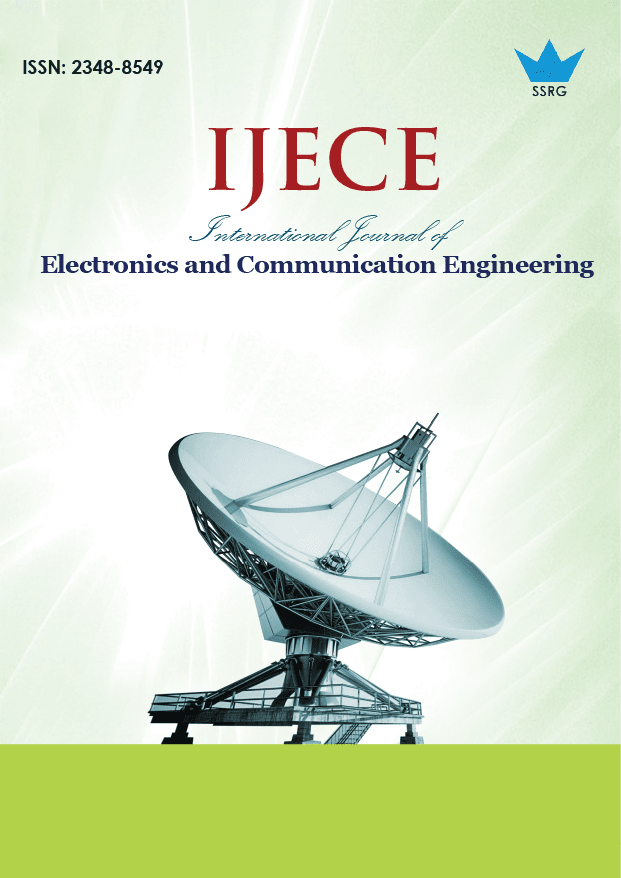
International Journal of Electronics and Communication Engineering |
© 2023 by SSRG - IJECE Journal |
Volume 10 Issue 6 |
Year of Publication : 2023 |
Authors : T. Rajendran, G. Divya, S. Sridhar, T. Anitha |
How to Cite?
T. Rajendran, G. Divya, S. Sridhar, T. Anitha, "A Deep Learning Based Methodological Analysis for Breast Cancer Classification," SSRG International Journal of Electronics and Communication Engineering, vol. 10, no. 6, pp. 52-68, 2023. Crossref, https://doi.org/10.14445/23488549/IJECE-V10I6P106
Abstract:
Breast cancer (BC) is the world’s most common and rapidly spreading disease. BC can be controlled, reducing the death rate if detected early. As a result, some researchers have recently developed deep-learning-based efficient algorithms for predicting cancer cell growth using different modalities of medical imaging, for example, mammograms, tomosynthesis, MRI ultrasound, etc., for their efficiency and accuracy. Only a few review articles on BC diagnosis synthesize some existing studies. These investigations, unfortunately, are unable to identify new structures and modalities in the diagnosis of BC. The changing frameworks of DL for BC detection are the subject of this review. This assessment explores the strengths and limitations of earlier deep-learning (DL) based methods, investigates the datasets employed, and examines image preprocessing approaches based on different medical imaging modalities. The performance results with evaluation, obstacles, and further enhancement are also provided.
Keywords:
Breast cancer, Dataset, Deep learning, Image classification, Medical imaging modalities.
References:
[1] Krishan K. Thakur, Devivasha Bordoloi, and Ajaikumar B. Kunnumakkara, “Alarming Burden of Triple-Negative Breast Cancer in India,” Clinical Breast Cancer, vol. 18, no. 3, pp. E393-E399, 2018.
[CrossRef] [Google Scholar] [Publisher Link]
[2] Cancer Statistics-Indian Against Cancer, Statistics - India Against Cancer, 2018. [Online]. Available: http://cancerindia.org.in/cancer-statistics/
[3] Globocan IAC, Globocan 2018_ India Factsheet - India Against Cancer, 2018. [Online]. Available: http://cancerindia.org.in/globocan-2018-india-factsheet/
[4] Xiaoli Yao et al., “A Comparison of Mammography, Ultrasonography, and Far-Infrared Thermography with Pathological Results in Screening and Early Diagnosis of Breast Cancer,” Asian Biomedicine, vol. 8, no. 1, pp. 11-19, 2014.
[CrossRef] [Google Scholar] [Publisher Link]
[5] PBCR, Trends of Breast Cancer in India, 2020. [Online]. Available: https://www.breastcancerindia.net/statistics/trends.html [6] Iqbal H. Sarker, “Machine Learning: Algorithms, Real-World Applications and Research Directions,” SN Computer Science, vol. 2, 2021.
[CrossRef] [Google Scholar] [Publisher Link]
[7] Gunjan Chugh, Shailender Kumar, and Nanhay Singh, “Survey on Machine Learning and Deep Learning Applications in Breast Cancer Diagnosis,” Cognitive Computation, vol. 13, no. 6, pp. 1451-1470, 2021.
[CrossRef] [Google Scholar] [Publisher Link]
[8] Mariam Shadan et al., “Histological Categorization of Stromal Desmoplasia in Breast Cancer and Its Diagnostic and Prognostic Utility,” SSRG International Journal of Medical Science, vol. 4, no. 6, pp. 8-11, 2017.
[CrossRef] [Google Scholar] [Publisher Link]
[9] Nidhi Mongoriya, and Vinod Patel, “Review the Breast Cancer Detection Technique using Hybrid Machine Learning,” SSRG International Journal of Computer Science and Engineering, vol. 8, no. 6, pp. 5-8, 2021.
[CrossRef] [Publisher Link]
[10] Seung Ja Kim et al., “Comparison of Two Software Versions of A Commercially Available Computer-Aided Detection (CAD) System for Detecting Breast Cancer,” Acta Radiologica, vol. 51, no. 5, pp. 482-490, 2010.
[CrossRef] [Google Scholar] [Publisher Link]
[11] Kaizhu Huang et al., Deep Learning: Fundamentals, Theory and Applications, Cognitive Computation Trends (COCT), Springer, vol. 2, pp. 1-163, 2019.
[CrossRef] [Google Scholar] [Publisher Link]
[12] S. Kevin Zhou et al., “A Review of Deep Learning in Medical Imaging: Imaging Traits, Technology Trends, Case Studies with Progress Highlights, and Future Promises,” Proceedings of the IEEE, vol. 109, no. 5, pp. 820-838, 2021.
[CrossRef] [Google Scholar] [Publisher Link]
[13] Zulaiha Parveen A, and T. Senthil Kumar, “The Deep Learning Methodology for Improved Breast Cancer Diagnosis in MRI,” International Journal of Computer and Organization Trends, vol. 11, no. 3, pp. 11-14, 2021.
[Publisher Link]
[14] Brotobor Deliverance, Edeawe Isaac Osahogie, and Brotobor Onoriode, “Awareness and Practice of Student Nurses on Breast Self Examination: A Risk Assessment Tool for Breast Cancer,” SSRG International Journal of Nursing and Health Science, vol. 6, no. 1, pp. 66-69, 2020.
[CrossRef] [Publisher Link]
[15] Nan Wu et al., “Deep Neural Networks Improve Radiologists’ Performance in Breast Cancer Screening,” IEEE Transactions on Medical Imaging, vol. 39, no. 4, pp. 1184-1194, 2019.
[CrossRef] [Google Scholar] [Publisher Link]
[16] Yan Wang et al., “Feature-Sensitive Deep Convolutional Neural Network for Multi-instance Breast Cancer Detection,” IEEE/ACM Transactions on Computational Biology and Bioinformatics, vol. 19, no. 4, pp. 2241-2251, 2022.
[CrossRef] [Google Scholar] [Publisher Link]
[17] Zhiqiong Wang et al., “Breast Cancer Detection using Extreme Learning Machine Based on Feature Fusion with CNN Deep Available Datasets,” IEEE Access, vol. 7, pp. 105146-105158, 2019.
[CrossRef] [Google Scholar] [Publisher Link]
[18] Jing Zheng et al., “Deep Learning Assisted Efficient AdaBoost Algorithm for Breast Cancer Detection and Early Diagnosis,” IEEE Access, vol. 8, pp. 96946-96954, 2020.
[CrossRef] [Google Scholar] [Publisher Link]
[19] H. S. Laxmisagar, and M. C. Hanumantharaju, “Evaluating the Statistical Stability of POSIT Arithmetic and IEEE 754 Float to Accelerate Data for Detection of Breast Cancer,” SSRG International Journal of Electrical and Electronics Engineering, vol. 9, no. 9, pp. 47-53, 2022.
[CrossRef] [Publisher Link]
[20] Md. Nawaj Sharif et al., “Design and Simulation of a Circular Microstrip Patch Antenna for Breast Cancer Diagnosis,” International Journal of Recent Engineering Science, vol. 7, no. 3, pp. 57-60, 2020.
[CrossRef] [Google Scholar] [Publisher Link]
[21] Shahan Yamin Siddiqui et al., “IoMT Cloud-Based Intelligent Prediction of Breast Cancer Stages Empowered with Deep Learning,” IEEE Access, vol. 9, pp. 146478-146491, 2021.
[CrossRef] [Google Scholar] [Publisher Link]
[22] Muayad Sadik Croock et al., “Early Detection of Breast Cancer using Mammography Images and Software Engineering Process,” Telkomnika, vol. 18, no. 4, pp. 1784-1794, 2020.
[CrossRef] [Google Scholar] [Publisher Link]
[23] Abeer Saber et al., “A Novel Deep-Learning Model for Automatic Detection and Classification of Breast Cancer using the TransferLearning Technique,” IEEE Access, vol. 9, pp. 71194-71209, 2021.
[CrossRef] [Google Scholar] [Publisher Link]
[24] Xin Shu et al., “Deep Neural Networks with Region-Based Pooling Structures for Mammographic Image Classification,” IEEE Transactions on Medical Imaging, vol. 39, no. 6, pp. 2246-2255, 2020.
[CrossRef] [Google Scholar] [Publisher Link]
[25] Dehua Chen, Meihua Huang, and Weimin Li, “Knowledge-Powered Deep Breast Tumour Classification with Multiple Medical Reports,” IEEE/ACM Transactions on Computational Biology and Bioinformatics, vol. 18, no. 3, pp. 891-901, 2019.
[CrossRef] [Google Scholar] [Publisher Link]
[26] Heyi Li et al., “Dual Convolutional Neural Networks for Breast Mass Segmentation and Diagnosis in Mammography,” IEEE Transactions on Medical Imaging, vol. 41, no. 1, pp. 3-13, 2021.
[CrossRef] [Google Scholar] [Publisher Link]
[27] Tianyu Shen et al., “Hierarchical Fused Model with Deep Learning and Type-2 Fuzzy Learning for Breast Cancer Diagnosis,” IEEE Transactions on Fuzzy Systems, vol. 28, no. 12, pp. 3204-3218, 2020.
[CrossRef] [Google Scholar] [Publisher Link]
[28] Xiaofei Zhang et al., “Classification of Whole Mammogram and Tomosynthesis Images using Deep Convolutional Neural Networks,” IEEE Transactions on NanoBioscience, vol. 17, no. 3, pp. 237-242, 2018.
[CrossRef] [Google Scholar] [Publisher Link]
[29] Sharaf J. Malebary, and Arshad Hashmi, “Automated Breast Mass Classification System using Deep Learning and Ensemble Learning in Digital Mammogram,” IEEE Access, vol. 9, pp. 55312-55328, 2021.
[CrossRef] [Google Scholar] [Publisher Link]
[30] Juan Chen et al., “A Novel Multi-Scale Adversarial Networks for Precise Segmentation of X-Ray Breast Mass,” IEEE Access, vol. 8, pp. 103772-103781, 2020.
[CrossRef] [Google Scholar] [Publisher Link]
[31] T. Kavitha et al., “Deep Learning Based Capsule Neural Network Model for Breast Cancer Diagnosis using Mammogram Images,” Interdisciplinary Sciences: Computational Life Sciences, vol. 14, pp. 113-129, 2021.
[CrossRef] [Google Scholar] [Publisher Link]
[32] Rajeshwari S. Patil, and Nagashettappa Biradar, “Automated Mammogram Breast Cancer Detection using the Optimized Combination of Convolutional and Recurrent Neural Network,” Evolutionary Intelligence, vol. 14, no. 4, pp. 1459-1474, 2021.
[CrossRef] [Google Scholar] [Publisher Link]
[33] S. S. Ittannavar, and R. H. Havaldar, “Detection of Breast Cancer using the Infinite Feature Selection with Genetic Algorithm and Deep Neural Network,” Distributed and Parallel Databases, vol. 40, no. 4, pp. 675-697, 2021.
[CrossRef] [Google Scholar] [Publisher Link]
[34] Idowu Sunday Oyetade et al., “Hybridized Deep Convolutional Neural Network and Fuzzy Support Vector Machines for Breast Cancer Detection,” SN Computer Science, vol. 3, no. 1, pp. 1-14, 2022.
[CrossRef] [Google Scholar] [Publisher Link]
[35] Jayesh George Melekoodappattu et al., “Breast Cancer Detection in Mammogram: Combining Modified CNN and Texture FeatureBased Approach,” Journal of Ambient Intelligence and Humanized Computing, pp. 1-10, 2022.
[CrossRef] [Google Scholar] [Publisher Link]
[36] Enas M. F. El Houby, and Nisreen I. R. Yassin, “Malignant and Nonmalignant Classification of Breast Lesions in Mammograms using Convolutional Neural Networks,” Biomedical Signal Processing and Control, vol. 70, 2021.
[CrossRef] [Google Scholar] [Publisher Link]
[37] Chunbo Xu et al., “ARF-Net: An Adaptive Receptive Field Network for Breast Mass Segmentation in Whole Mammograms and Ultrasound Images,” Biomedical Signal Processing and Control, vol. 71, 2022.
[CrossRef] [Google Scholar] [Publisher Link]
[38] Chang-Min Kim, Roy C. Park, and Ellen J. Hong, “Breast Mass Classification using eLFA Algorithm Based on CRNN Deep Learning Model,” IEEE Access, vol. 8, pp. 197312-197323, 2020.
[CrossRef] [Google Scholar] [Publisher Link]
[39] Yi Wang et al., “Deeply-Supervised Networks with Threshold Loss for Cancer Detection in Automated Breast Ultrasound,” IEEE Transactions on Medical Imaging, vol. 39, no. 4, pp. 866-876, 2019.
[CrossRef] [Google Scholar] [Publisher Link]
[40] Sampa Misra et al., “Bi-Modal Transfer Learning for Classifying Breast Cancers via Combined B-mode and Ultrasound Strain Imaging,” IEEE Transactions on Ultrasonics, Ferroelectrics, and Frequency Control, vol. 69, no. 1, pp. 222-232, 2021.
[CrossRef] [Google Scholar] [Publisher Link]
[41] Moi Hoon Yap et al., “Automated Breast Ultrasound Lesions Detection using Convolutional Neural Networks,” IEEE Journal of Biomedical and Health Informatics, vol. 22, no. 4, pp. 1218-1226, 2017.
[CrossRef] [Google Scholar] [Publisher Link]
[42] Xiaofeng Qi et al., “Automated Diagnosis of Breast Ultrasonography Images using Deep Neural Networks,” Medical Image Analysis, vol. 52, pp. 185-198, 2019.
[CrossRef] [Google Scholar] [Publisher Link]
[43] Aleksandar Vakanski, Min Xian, and Phoebe E. Freer, “Attention-Enriched Deep Learning Model for Breast Tumour Segmentation in Ultrasound Images,” Ultrasound in Medicine & Biology, vol. 46, no. 10, pp. 2819-283, 2020.
[CrossRef] [Google Scholar] [Publisher Link]
[44] Mehedi Masud et al., “Pre-Trained Convolutional Neural Networks for Breast Cancer Detection using Ultrasound Images,” ACM Transactions on Internet Technology, vol. 21, no. 4, pp. 1-17, 2021.
[CrossRef] [Google Scholar] [Publisher Link]
[45] Bashir Zeimarani et al., “A Novel Breast Tumour Classification in Ultrasound Images, using Deep Convolutional Neural Network,” XXVI Brazilian Congress on Biomedical Engineering, pp. 89-94, 2019.
[CrossRef] [Google Scholar] [Publisher Link]
[46] Yong Pi et al., “Automated Diagnosis of Multi-Plane Breast Ultrasonography Images using Deep Neural Networks,” Neurocomputing, vol. 403, pp. 371-382, 2020.
[CrossRef] [Google Scholar] [Publisher Link]
[47] Tanzila Saba et al., “Optimizing the Transfer‐Learning with Pre-Trained Deep Convolutional Neural Networks for First Stage Breast Tumour Diagnosis using Breast Ultrasound Visual Images,” Microscopy Research and Technique, vol. 85, no. 4, pp. 1444-1453, 2022.
[CrossRef] [Google Scholar] [Publisher Link]
[48] Heqing Zhang et al., “Diagnostic Efficiency of the Breast Ultrasound Computer-Aided Prediction Model Based on Convolutional Neural Network in Breast Cancer,” Journal of Digital Imaging, vol. 33, no. 5, pp. 1218-1223, 2020.
[CrossRef] [Google Scholar] [Publisher Link]
[49] Yi Wang et al., “Breast Cancer Classification in Automated Breast Ultrasound using A Multiview Convolutional Neural Network with Transfer Learning,” Ultrasound in Medicine & Biology, vol. 46, no. 5, pp. 1119-1132, 2020.
[CrossRef] [Google Scholar] [Publisher Link]
[50] Hongwei Feng, “A Knowledge-Driven Feature Learning and Integration Method for Breast Cancer Diagnosis on Multi-Sequence MRI,” Magnetic Resonance Imaging, vol. 69, pp. 40-48, 2020.
[CrossRef] [Google Scholar] [Publisher Link]
[51] Deepak Ranjan Nayak et al., “A Deep Stacked Random Vector Functional Link Network Autoencoder for Diagnosis of Brain Abnormalities and Breast Cancer,” Biomedical Signal Processing and Control, vol. 58, 2020.
[CrossRef] [Google Scholar] [Publisher Link]
[52] Mehmet Ufuk Dalmış et al., “Fully Automated Detection of Breast Cancer in Screening MRI using Convolutional Neural Networks,” Journal of Medical Imaging, vol. 5, no. 1, p. 014502, 2018.
[CrossRef] [Google Scholar] [Publisher Link]
[53] Reza Rasti, Mohammad Teshnehlab, and Son Lam Phung, “Breast Cancer Diagnosis in DCE-MRI using Mixture Ensemble of Convolutional Neural Networks,” Pattern Recognition, vol. 72, pp. 381-390, 2017.
[CrossRef] [Google Scholar] [Publisher Link]
[54] Yang Zhang et al., “Prediction of Breast Cancer Molecular Subtypes on DCE-MRI using A Convolutional Neural Network with Transfer Learning Between Two Centers,” European Radiology, vol. 31, no. 4, pp. 2559-2567, 2021.
[CrossRef] [Google Scholar] [Publisher Link]
[55] Rachel Anderson et al., “Evaluating Deep Learning Techniques for Dynamic Contrast-Enhanced MRI in the Diagnosis of Breast Cancer,” Proceedings Medical Imaging 2019: Computer-Aided Diagnosis, vol. 10950, pp. 26-32, 2019.
[CrossRef] [Google Scholar] [Publisher Link]
[56] Yang Zhang et al., “Automatic Detection and Segmentation of Breast Cancer on MRI using Mask R-CNN Trained on Non–Fat-Sat Images and Tested on Fat-Sat Images,” Academic Radiology, vol. 29, no. 1, pp. S135-S144, 2022.
[CrossRef] [Google Scholar] [Publisher Link]
[57] Mina Yousefi, Adam Krzyżak, and Ching Y. Suen, “Mass Detection in Digital Breast Tomosynthesis Data using Convolutional Neural Networks and Multiple Instance Learning,” Computers in Biology and Medicine, vol. 96, pp. 283-293, 2018.
[CrossRef] [Google Scholar] [Publisher Link]
[58] Tomoyuki Fujioka et al., “Deep-Learning Approach with Convolutional Neural Network for Classification of Maximum Intensity Projections of Dynamic Contrast-Enhanced Breast Magnetic Resonance Imaging,” Magnetic Resonance Imaging, vol. 75, pp. 1-8, 2021.
[CrossRef] [Google Scholar] [Publisher Link]
[59] Ravi K. Samala et al., “Evolutionary Pruning of Transfer Learned Deep Convolutional Neural Network for Breast Cancer Diagnosis in Digital Breast Tomosynthesis,” Physics in Medicine and Biology, vol. 63, no. 9, 2018.
[CrossRef] [Google Scholar] [Publisher Link]
[60] Vitoantonio Bevilacqua et al., “A Performance Comparison between Shallow and Deeper Neural Networks Supervised Classification of Tomosynthesis Breast Lesions Images,” Cognitive Systems Research, vol. 53, pp. 3-19, 2019.
[CrossRef] [Google Scholar] [Publisher Link]
[61] Ravi K. Samala et al., “Breast Cancer Diagnosis in Digital Breast Tomosynthesis: Effects of Training Sample Size on Multi-Stage Transfer Learning using Deep Neural Nets,” IEEE Transactions on Medical Imaging, vol. 38, no. 3, pp. 686-696, 2019.
[CrossRef] [Google Scholar] [Publisher Link]
[62] Ming Fan et al., “Computer-Aided Detection of Mass in Digital Breast Tomosynthesis using A Faster Region-Based Convolutional Neural Network,” Methods, vol. 166, pp. 103-111, 2019.
[CrossRef] [Google Scholar] [Publisher Link]
[63] Kayla Mendel et al., “Transfer Learning from Convolutional Neural Networks for Computer-Aided Diagnosis: A Comparison of Digital Breast Tomosynthesis and Full-Field Digital Mammography,” Academic Radiology, vol. 26, no. 6, pp. 735-743, 2019.
[CrossRef] [Google Scholar] [Publisher Link]
[64] Gongfa Jiang et al., “Synthesis of Mammogram from Digital Breast Tomosynthesis using Deep Convolutional Neural Network with Gradient Guided cGANs,” IEEE Transactions on Medical Imaging, vol. 40, no. 8, pp. 2080-2091, 2021.
[CrossRef] [Google Scholar] [Publisher Link]
[65] Hu Zilong et al., “Deep Learning for Image-Based Cancer Detection and Diagnosis-A Survey,” Pattern Recognition, vol. 83, pp. 134- 149, 2018.
[CrossRef] [Google Scholar] [Publisher Link]
[66] Ravi Aggarwal et al., “Diagnostic Accuracy of Deep Learning in Medical Imaging: A Systematic Review and Meta-Analysis,” NPJ Digital Medicine, vol. 4, no. 1, pp.1-23, 2021.
[CrossRef] [Google Scholar] [Publisher Link]
[67] Naveed Chouhan et al., “Deep Convolutional Neural Network and Emotional Learning Based Breast Cancer Detection using Digital Mammography,” Computers in Biology and Medicine, vol. 132, 2021.
[CrossRef] [Google Scholar] [Publisher Link]
[68] Xin Li et al., “Digital Breast Tomosynthesis versus Digital Mammography: Integration of Image Modalities Enhances Deep LearningBased Breast Mass Classification,” European Radiology, vol. 30, no. 2, pp. 778-788, 2020.
[CrossRef] [Google Scholar] [Publisher Link]
[69] R. Ricciardi et al., “A Deep Learning Classifier for Digital Breast Tomosynthesis,” Physica Medica, vol. 83, pp. 184-193, 2021.
[CrossRef] [Google Scholar] [Publisher Link]