An Intelligent Model for Improved Breast Cancer Prognosis
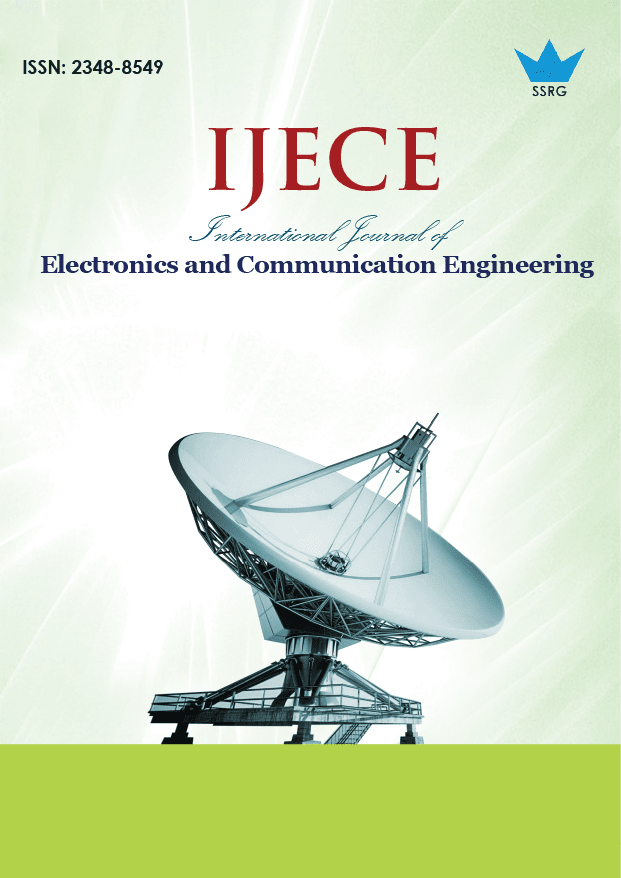
International Journal of Electronics and Communication Engineering |
© 2023 by SSRG - IJECE Journal |
Volume 10 Issue 8 |
Year of Publication : 2023 |
Authors : C. O. Iloghalu, U. F. Eze, A. M. John-Otumu, O. C. Nwokonkwo, E. O. Oshoiribhor, J. C. Onyeakazi, A. P. Aliga, S. A. Okolie, E. C. Nwokorie, L. C. Nnadi |
How to Cite?
C. O. Iloghalu, U. F. Eze, A. M. John-Otumu, O. C. Nwokonkwo, E. O. Oshoiribhor, J. C. Onyeakazi, A. P. Aliga, S. A. Okolie, E. C. Nwokorie, L. C. Nnadi, "An Intelligent Model for Improved Breast Cancer Prognosis," SSRG International Journal of Electronics and Communication Engineering, vol. 10, no. 8, pp. 36-47, 2023. Crossref, https://doi.org/10.14445/23488549/IJECE-V10I8P104
Abstract:
This research suggests developing a deep learning model using customized CNN to categorize and predict breast cancer in a timely period. The model utilizes a large dataset of breast cancer images obtained from Kaggle, an online research repository. Pre-processing techniques were applied to the images to eliminate noise, such as shadows on the images, and resize the images to lessen the high computation cost. The dataset was separated into training set 80% (48, 852) and test set 20% (16, 284). CNN was employed to mine meaningful features from the images and to classify them based on predefined criteria, assessing the presence and severity of breast cancer. Additionally, the model could provide treatment recommendations depending on the patient's health account and other pertinent aspects. The model's performance was evaluated using a confusion matrix, revealing a 95% accuracy rate, 100% recall value, 90% precision value, and 95% F1 score. The classifier's AUC value was 88%, indicating high reliability for breast cancer prognosis. The proposed methodology may significantly increase diagnostic speed and accuracy, resulting in earlier detection and better patient outcomes.
Keywords:
Breast cancer, Classification, CNN, Deep learning, Prognosis.
References:
[1] HealthThink, Breast Cancer and the Nigerian Woman, 2021. [Online]. Available: https://healththink.org/breast-cancer-and-the-nigerian-woman/
[2] Kornelia Polyak, “Heterogeneity in Breast Cancer,” Journal of Clinical Investigation, vol. 121, no. 10, pp. 3786-3788, 2020.
[CrossRef] [Google Scholar] [Publisher Link]
[3] Douglas G. Altman, “Prognostic Models: A Methodological Framework and Review of Models for Breast Cancer,” Cancer Investigation, vol. 27, no. 3, pp. 235-243, 2019.
[CrossRef] [Google Scholar] [Publisher Link]
[4] CDC (Centers for Disease Control and Prevention), 2021. [Online]. Available: https://www.cdc.gov/cancer/breast/basic_info/what-is-breast-cancer.htm
[5] Noreen Fatima et al., “Prediction of Breast Cancer, Comparative Review of Machine Learning Techniques, and their Analysis,” IEEE Access, vol. 8, pp. 150360-150376, 2020.
[CrossRef] [Google Scholar] [Publisher Link]
[6] Seong Ho Park, and Kyunghwa Han, “Methodologic Guide for Evaluating Clinical Performance and Effect of Artificial Intelligence Technology for Medical Diagnosis and Prediction,” Radiology, vol. 286, no. 3, pp. 800-809, 2018.
[CrossRef] [Google Scholar] [Publisher Link]
[7] Md. Milon Islam et al., “Breast Cancer Prediction: A Comparative Study using Machine Learning Techniques,” SN Computer Science, vol. 1, no. 5, 2020.
[CrossRef] [Google Scholar] [Publisher Link]
[8] Varsha Nemade, Sunil Pathak, and Ashutosh Kumar Dubey, “Hybrid Deep Convolutional Neural Network Approach for Detecting Breast Cancer in Mammography Images,” SSRG International Journal of Electrical and Electronics Engineering, vol. 10, no. 5, pp. 102-119, 2023.
[CrossRef] [Publisher Link]
[9] Lulu Wang, “Early Diagnosis of Breast Cancer,” Sensors, vol. 17, no. 7, pp. 1-20, 2017.
[CrossRef] [Google Scholar] [Publisher Link]
[10] NHS, Causes, Breast Cancer in Women, 2021. [Online]. Available: https://www.nhs.uk/conditions/breast-cancer/causes/
[11] B. Johnson, P. Keerthi Vasan, and V. Thillaivendan, “Performance of Hyperthermia for Breast Cancer,” SSRG International Journal of Applied Physics, vol. 3, no. 2, pp. 6-10, 2016.
[CrossRef] [Publisher Link]
[12] Mogana Darshini Ganggayah et al., “Predicting Factors for Survival of Breast Cancer Patients using Machine Learning Techniques,” BMC Medical Informatics and Decision Making, vol. 19, no. 1, pp. 24-34, 2019.
[CrossRef] [Google Scholar] [Publisher Link]
[13] Mariam Shadan et al., “Histological Categorization of Stromal Desmoplasia in Breast Cancer and Its Diagnostic and Prognostic Utility,” SSRG International Journal of Medical Science, vol. 4, no. 6, pp. 8-11, 2017.
[CrossRef] [Google Scholar] [Publisher Link]
[14] Maxine A. Papadakis et al., Current Medical Diagnosis and Treatment, 59th ed., McGraw-Hill Education, 2019.
[15] Niharika G. Maity, and Sreerupa Das, “Machine Learning for Improved Diagnosis and Prognosis in Healthcare,” 2017 IEEE Aerospace Conference, pp. 1-9, 2017.
[CrossRef] [Google Scholar] [Publisher Link]
[16] Meriem Amrane et al., “Breast Cancer Classification using Machine Learning,” 2018 Electric Electronics, Computer Science, Biomedical Engineerings’ Meeting (EBBT), Istanbul, Turkey, pp. 1-4, 2018.
[CrossRef] [Google Scholar] [Publisher Link]
[17] Samuel O. Azubuike et al., “Rising Global Burden of Breast Cancer: The Case of Sub-Saharan Africa (with Emphasis on Nigeria) and Implications for Regional Development: A Review,” World Journal of Surgical Oncology, vol. 16, no. 1, pp. 222-231, 2018.
[CrossRef] [Google Scholar] [Publisher Link]
[18] Pelumi E. Oguntunde, Adebowale O. Adejumo, and Hilary I. Okagbue, “Breast Cancer Patients in Nigeria: Data Exploration Approach,” Data in Brief, vol. 15, pp. 47-57, 2017.
[CrossRef] [Google Scholar] [Publisher Link]
[19] Constance D. Lehman et al., “National Performance Benchmarks for Modern Screening Digital Mammography: Update from the Breast Cancer Surveillance Consortium,” Radiology, vol. 283, no. 1, pp. 49-58, 2017.
[CrossRef] [Google Scholar] [Publisher Link]
[20] Habib Dhahri et al., “Automated Breast Cancer Diagnosis Based on Machine Learning Algorithms,” Journal of Healthcare Engineering, vol. 2019, pp. 1-11, 2019.
[CrossRef] [Google Scholar] [Publisher Link]
[21] G. Rajasekaran, and C. Sunitha Ram, “Effective Breast Cancer Prediction Based on Feature Extraction, Fusion and Selection using Hybrid Methodologies,” SSRG International Journal of Electrical and Electronics Engineering, vol. 10, no. 5, pp. 131-142, 2023.
[CrossRef] [Publisher Link]
[22] Shafaq Abbas et al., “BCD-WERT: A Novel Approach for Breast Cancer Detection using Whale Optimization Based Efficient Features and Extremely Randomized Tree Algorithm,” PeerJ Computer Science, vol. 7, pp. 1-20, 2021.
[CrossRef] [Google Scholar] [Publisher Link]
[23] Hiba Asri et al., “Using Machine Learning Algorithms for Breast Cancer Risk Prediction and Diagnosis,” Procedia Computer Science, vol. 83, pp. 1064-1069, 2016.
[CrossRef] [Google Scholar] [Publisher Link]
[24] Saad Awadh Alanazi et al., “Boosting Breast Cancer Detection using Convolutional Neural Network,” Journal of Healthcare Engineering, vol. 2021, pp. 1-11, 2021.
[CrossRef] [Google Scholar] [Publisher Link]
[25] Ahmad Taher Azar, and Shaimaa Ahmed El-Said, “Probabilistic Neural Network for Breast Cancer Classification,” Neural Computing and Applications, vol. 23, pp. 1737-1751, 2013.
[CrossRef] [Google Scholar] [Publisher Link]
[26] Emina Aličković, and Abdulhamit Subasi, “Breast Cancer Diagnosis using GA Feature Selection and Rotation Forest,” Neural Computing and Applications, vol. 28, pp. 753-763, 2017.
[CrossRef] [Google Scholar] [Publisher Link]
[27] Jeffrey A. Tice et al., “Validation of the Breast Cancer Surveillance Consortium Model of Breast Cancer Risk,” Breast Cancer Research and Treatment, vol. 175, no. 2, pp. 519-523, 2019.
[CrossRef] [Google Scholar] [Publisher Link]
[28] Essam Rashed, and M. Samir Abou El Seoud, “Deep Learning Approach for Breast Cancer Diagnosis,” ICSIE '19: Proceedings of the 8 th International Conference on Software and Information Engineering, pp. 243-247, 2019.
[CrossRef] [Google Scholar] [Publisher Link]
[29] Mohammad Mehdi Keikha, and Yahya Kord Tamandani, “Breast Cancer Detection using Deep Multilayer Neural Networks,” Journal of Epigenetics, vol. 3, no. 1, pp. 27-34, 2022.
[CrossRef] [Google Scholar] [Publisher Link]
[30] K. Shwetha et al., “Breast Cancer Detection using Deep Learning Technique,” International Journal of Engineering Research & Technology (IJERT), vol. 6, no. 13, pp. 1-4, 2018.
[Publisher Link]
[31] R. Chtihrakkannan et al., “Breast Cancer Detection using Machine Learning,” International Journal of Innovative Technology and Exploring Engineering (IJITEE), vol. 8, no. 11, pp. 3123-3126, 2019.
[CrossRef] [Publisher Link]