Design and Evaluation of Image Quality Enhancement to Augment Object Detection and Tracking Framework to Support Visually Impaired Persons
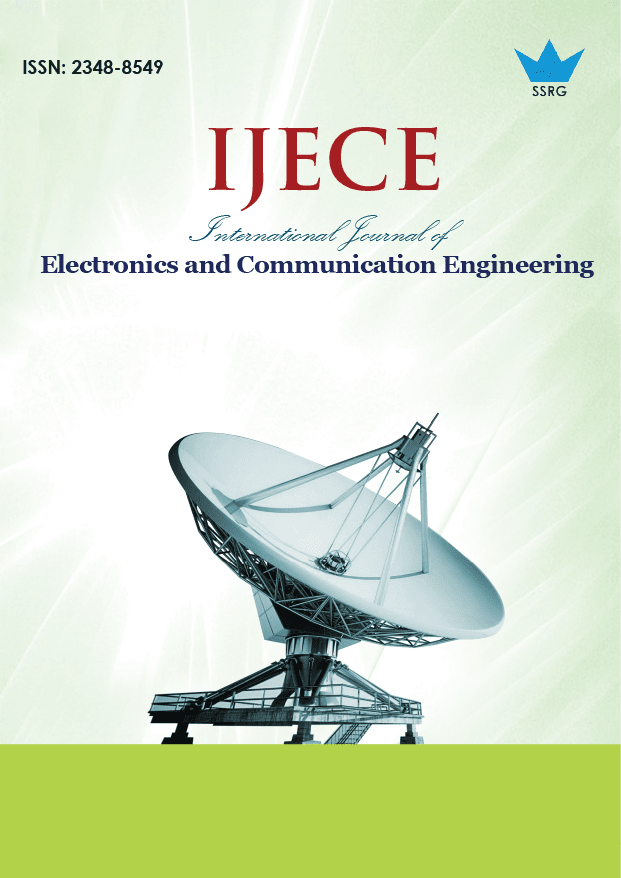
International Journal of Electronics and Communication Engineering |
© 2023 by SSRG - IJECE Journal |
Volume 10 Issue 9 |
Year of Publication : 2023 |
Authors : S. Sajini, B. Pushpa |
How to Cite?
S. Sajini, B. Pushpa, "Design and Evaluation of Image Quality Enhancement to Augment Object Detection and Tracking Framework to Support Visually Impaired Persons," SSRG International Journal of Electronics and Communication Engineering, vol. 10, no. 9, pp. 52-62, 2023. Crossref, https://doi.org/10.14445/23488549/IJECE-V10I9P106
Abstract:
Object Tracking after Prediction for the Visually Impaired was intricate, especially when identifying the right object with the right identity. The Image Quality enhancement from captured images acquired from external devices significantly impacts the prediction of objects and tracking of their movement in Visual impairment devices. The foremost objective of the study was to design a framework for enhanced predictions in object tracking after prediction for visually impaired people. Initially, the captured image was applied with image quality enhancement measures in terms of filtering, segmentation and feature extraction to ascertain a quality image that could be used for object detection and tracking with enhanced prediction efficiency like accuracy, sensitivity, specificity, etc. The experimental analysis showed that the captured frame had been enhanced to a quality level that enhanced the prediction evaluation measures based on the confusion matrix assessment techniques. The research showed an enhanced accuracy level of 98.3% in comparison to benchmark models like Detectron2 (97.3%), YOLOv5 (84%), Faster RCNN (46%), HFYOLO (95.5%) to prove that when the image quality was augmented, Object Tracking after Prediction could be augmented being directly proportional to each other.
Keywords:
Object Tracking after Prediction, Visually impaired, Evaluation measures, Image quality enhancement, Confusion matrix.
References:
[1] John-Ross Rizzo et al., “The Global Crisis of Visual Impairment: An Emerging Global Health Priority Requiring Urgent Action,” Disability and Rehabilitation: Assistive Technology, vol. 18, no. 3, pp. 240-245, 2023.
[CrossRef] [Google Scholar] [Publisher Link]
[2] Seid Worku et al., “The Magnitude of Refractive Error and its Associated Factors Among Patients Visiting Ophthalmology Clinics in Southern Ethiopia, 2022,” Clinical Ophthalmology, pp. 1801-1811, 2023.
[CrossRef] [Google Scholar] [Publisher Link]
[3] Fabiana Sofia Ricci et al., “Virtual Reality as a Means to Explore Assistive Technologies for the Visually Impaired,” PLOS Digital Health, vol. 2, no. 6, 2023.
[CrossRef] [Google Scholar] [Publisher Link]
[4] Varun Kumar Reja, Koshy Varghese, and Quang Phuc Ha, “Computer Vision-Based Construction Progress Monitoring,” Automation in Construction, vol. 138, 2022.
[CrossRef] [Google Scholar] [Publisher Link]
[5] Fahad Ashiq et al., “CNN-Based Object Recognition and Tracking System to Assist Visually Impaired People,” IEEE Access, vol. 10, pp. 14819-14834, 2022.
[CrossRef] [Google Scholar] [Publisher Link]
[6] Wei Chen et al., “2D and 3D Object Detection Algorithms from Images: A Survey,” Array, vol. 19, 2023.
[CrossRef] [Google Scholar] [Publisher Link]
[7] Imran Ahmed et al., “Towards Collaborative Robotics in Top View Surveillance: A Framework for Multiple Object Tracking by Detection Using Deep Learning,” IEEE/CAA Journal of Automatica Sinica, vol. 8, no. 7, pp. 1253-1270, 2021.
[CrossRef] [Google Scholar] [Publisher Link]
[8] Jingwei Liu et al., “Feature Rescaling and Fusion for Tiny Object Detection,” IEEE Access, vol. 9, pp. 62946-62955, 2021.
[CrossRef] [Google Scholar] [Publisher Link]
[9] Zhengxia Zou et al., “Object Detection in 20 Years: A Survey,” Proceedings of the IEEE, vol. 111, no. 3, pp. 257-276, 2023.
[CrossRef] [Google Scholar] [Publisher Link]
[10] K.V. Sriram, and R.H. Havaldar, “Analytical Review and Study on Object Detection Techniques in the Image,” International Journal of Modeling, Simulation, and Scientific Computing, vol. 12, no. 5, 2021.
[CrossRef] [Google Scholar] [Publisher Link]
[11] Pingli Ma et al., “A State-of-the-Art Survey of Object Detection Techniques in Microorganism Image Analysis: From Classical Methods to Deep Learning Approaches,” Artificial Intelligence Review, vol. 56, no. 2, pp. 1627-1698, 2023.
[CrossRef] [Google Scholar] [Publisher Link]
[12] Kunyi Li, and Lu Cao, “A Review of Object Detection Techniques,” 5th International Conference on Electromechanical Control Technology and Transportation, pp. 385-390, 2020.
[CrossRef] [Google Scholar] [Publisher Link]
[13] Xuesong Li, and Jose E. Guivant, “Efficient and Accurate Object Detection with Simultaneous Classification and Tracking under Limited Computing Power,” IEEE Transactions on Intelligent Transportation Systems, vol. 24, no. 6, pp. 5740-5751, 2023.
[CrossRef] [Google Scholar] [Publisher Link]
[14] Samed Şatir et al., “A Novel Approach to Radiographic Detection of Growth Development Period with Hand-Wrist Radiographs: A Preliminary Study with ImageJ Imaging Software,” Orthodontics and Craniofacial Research, vol. 26, no. 1, pp. 100-106, 2023.
[CrossRef] [Google Scholar] [Publisher Link]
[15] Mansi Mahendru, and Sanjay Kumar Dubey, “Real Time Object Detection with Audio Feedback Using Yolo Vs. Yolo_V3,” 2021 11th International Conference on Cloud Computing, Data Science and Engineering (Confluence), pp. 734-740, 2021.
[CrossRef] [Google Scholar] [Publisher Link]
[16] Muhammad Gufran Khan et al., “Development of ANPR Framework for Pakistani Vehicle Number Plates Using Object Detection and OCR,” Complexity, pp. 1-14, 2021.
[CrossRef] [Google Scholar] [Publisher Link]
[17] D.R. Niranjan, B.C. VinayKarthik, and Mohana, “Deep Learning-Based Object Detection Model for Autonomous Driving Research Using Carla Simulator,” 2021 2nd International Conference on Smart Electronics and Communication (ICOSEC), pp. 1251-1258, 2021.
[CrossRef] [Google Scholar] [Publisher Link]
[18] Shanglin Zhou et al., “An End-to-End Multi-Task Object Detection Using Embedded GPU in Autonomous Driving,” 2021 11th International Conference on Cloud Computing, Data Science and Engineering (Confluence), pp. 122-128, 2021.
[CrossRef] [Google Scholar] [Publisher Link]
[19] Zengyi Qin, Jinglu Wang, and Yan Lu, “Monogrnet: A General Framework for Monocular 3D Object Detection,” IEEE Transactions on Pattern Analysis and Machine Intelligence, vol. 44, no. 9, pp. 5170-5184, 2022.
[CrossRef] [Google Scholar] [Publisher Link]
[20] Barret Zoph et al., “Learning Data Augmentation Strategies for Object Detection,” European Conference on Computer Vision, pp. 566- 583, 2020.
[CrossRef] [Google Scholar] [Publisher Link]
[21] Silvia Rostianingsih, Alexander Setiawan, and Christopher Imantaka Halim, “COCO (Creating Common Object in Context) Dataset for Chemistry Apparatus,” Procedia Computer Science, vol. 171, pp. 2445-2452, 2020.
[CrossRef] [Google Scholar] [Publisher Link]
[22] V.R.S. Mani, A. Saravanaselvan, and N. Arumugam, “Performance Comparison of CNN, QNN and BNN Deep Neural Networks for Real-Time Object Detection Using ZYNQ FPGA Node,” Microelectronics Journal, vol. 119, 2022.
[CrossRef] [Google Scholar] [Publisher Link]
[23] Rafael Padilla, Sergio L. Netto, and Eduardo A.B. da Silva, “A Survey on Performance Metrics for Object-Detection Algorithms,” 2020 International Conference on Systems, Signals and Image Processing (IWSSIP), pp. 237-242, 2020.
[CrossRef] [Google Scholar] [Publisher Link]
[24] Zicong Jiang et al., “Real-Time Object Detection Method Based on Improved Yolov4-Tiny,” arXiv, pp. 1-11, 2020.
[CrossRef] [Google Scholar] [Publisher Link]
[25] Tsung-Yi Lin et al., “Microsoft Coco: Common Objects in Context,” European Conference on Computer Vision, vol. 8693, pp. 740-755, 2014.
[CrossRef] [Google Scholar] [Publisher Link]
[26] Ayushi Sharma et al., “Object Detection Using OpenCV and Python,” 2021 3rd International Conference on Advances in Computing, Communication Control and Networking (ICAC3N), pp. 501-505, 2021.
[CrossRef] [Google Scholar] [Publisher Link]