Integrated Approach for Enhanced EEG-Based Emotion Recognition with Hybrid Deep Neural Network and Optimized Feature Selection
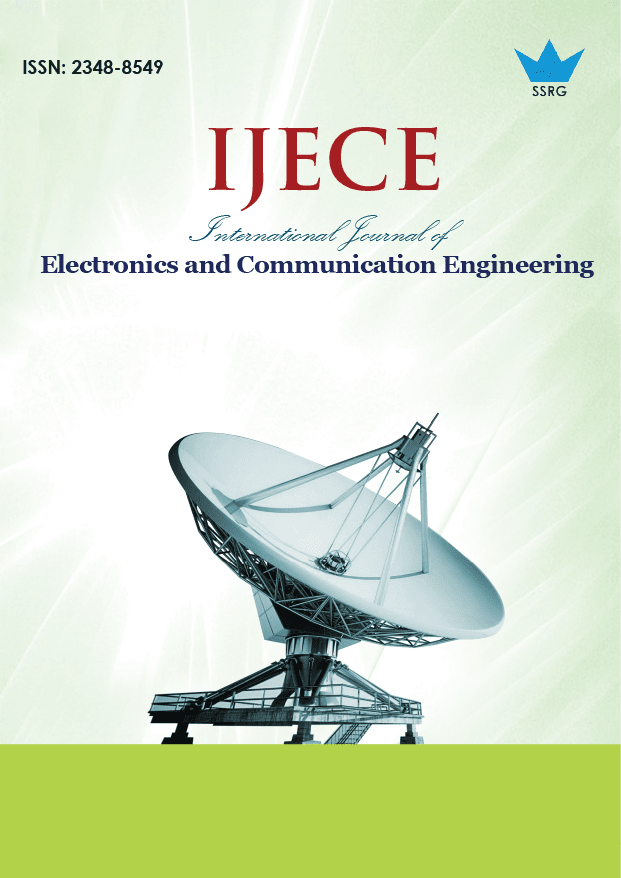
International Journal of Electronics and Communication Engineering |
© 2023 by SSRG - IJECE Journal |
Volume 10 Issue 11 |
Year of Publication : 2023 |
Authors : T. Manoj Prasath, R. Vasuki |
How to Cite?
T. Manoj Prasath, R. Vasuki, "Integrated Approach for Enhanced EEG-Based Emotion Recognition with Hybrid Deep Neural Network and Optimized Feature Selection," SSRG International Journal of Electronics and Communication Engineering, vol. 10, no. 11, pp. 55-68, 2023. Crossref, https://doi.org/10.14445/23488549/IJECE-V10I11P106
Abstract:
Emotion recognition through Electroencephalography (EEG) signals holds significant promise for a wide range of applications, from healthcare to human-computer interaction. This concept introduces a comprehensive approach to improve the accuracy and efficiency of EEG-based emotion recognition. It combines advanced signal processing techniques, hybrid feature selection methods and deep learning architectures, resulting in a robust and innovative framework. The proposed work begins with the preprocessing of EEG signals using an Orthogonal Wavelet Filter. This filter offers exceptional flexibility in balancing the preservation of relevant emotional information and reducing unwanted interference. This initial step is critical in ensuring the processed EEG signals are optimally prepared for subsequent analysis. To capture the time-frequency representations of EEG signals, the Empirical Mode Decomposition (EMD) technique is applied. EMD is known for extracting complex, non-stationary features from EEG data, which are often critical for understanding emotional states. A key innovation in this concept is the hybrid feature selection approach. It combines the Chaotic Squirrel Search Algorithm (CSSA), which leverages chaos theory for optimization, with the Whale Optimization Algorithm (WOA), a nature-inspired metaheuristic algorithm. This combination is designed to curate the feature set, retaining only the most discriminative elements for emotion classification. This process enhances the overall efficiency and accuracy of the classification task. For the final phase of emotion recognition, a novel hybrid deep learning architecture is employed. It combines an Attention-based Deep Convolutional Neural Network (DCNN) with Bidirectional Long Short-Term Memory (Bi-LSTM) networks. The CNN component is adept at automatically learning hierarchical features from EEG data, while the Bi-LSTM network captures temporal dependencies in the signals. Introducing attention mechanisms further refines the network’s ability to focus on salient features, improving the overall recognition performance. The resulting framework showcases a holistic approach to EEG-based emotion recognition, addressing challenges related to data preprocessing, feature selection, and deep learning model design.
Keywords:
EEG-based emotion recognition, Orthogonal Wavelet Filter, EMD, CSSA-WOA, and Attention-based DCNN-BiLSTM
References:
[1] Domencio De Berardis et al., “Emotional Dysregulation in Adolescents: Implications for the Development of Severe Psychiatric Disorders, Substance Abuse, and Suicidal Ideation and Behaviors,” Brain Sciences, vol. 10, no. 9, 2020.
[CrossRef] [Google Scholar] [Publisher Link]
[2] Yekta Said Can et al., “Personal Stress-Level Clustering and Decision-Level Smoothing to Enhance the Performance of Ambulatory Stress Detection with Smart Watches,” IEEE Access, vol. 8, pp. 38146-38163, 2020.
[CrossRef] [Google Scholar] [Publisher Link]
[3] Yuqi Wang et al., “EEG-Based Emotion Recognition Using a 2D CNN with Different Kernels,” Bioengineering, vol. 9, no. 6, pp. 231, 2022.
[CrossRef] [Google Scholar] [Publisher Link]
[4] Stanislaw Saganowski et al., “Emotion Recognition for Everyday Life Using Physiological Signals from Wearables: A Systematic Literature Review,” IEEE Transactions on Affective Computing, vol. 14, no. 3, pp. 1876-1897, 2023.
[CrossRef] [Google Scholar] [Publisher Link]
[5] Miguel Angel Sánchez-Cifo, Francisco Montero, and Maria Teresa Lopez, “A Methodology for Emotional Intelligence Testing in Elderly People with Low-Cost EEG and PPG Devices,” Journal of Ambient Intelligence and Humanized Computing, vol. 14, no. 3, pp. 2351-2367, 2023.
[CrossRef] [Google Scholar] [Publisher Link]
[6] Sandor Beniczky, and Donald L. Schomer, “Electroencephalography: Basic Biophysical and Technological Aspects Important for Clinical Applications,” Epileptic Disorders, vol. 22, no. 6, pp. 697-715, 2020.
[CrossRef] [Google Scholar] [Publisher Link]
[7] Md. Rabiul Islam et al., “EEG Channel Correlation Based Model for Emotion Recognition,” Computers in Biology and Medicine, vol. 136, 2021.
[CrossRef] [Google Scholar] [Publisher Link]
[8] Pragati Patel, R. Raghunandan, and Ramesh Naidu Annavarapu, “EEG-Based Human Emotion Recognition Using Entropy as a Feature Extraction Measure,” Brain Informatics, vol. 8, no. 1, pp. 1-13, 2021.
[CrossRef] [Google Scholar] [Publisher Link]
[9] Wei Li et al., “A Novel Spatio-Temporal Field for Emotion Recognition Based on EEG Signals,” IEEE Sensors Journal, vol. 21, no. 23, pp. 26941-26950, 2021.
[CrossRef] [Google Scholar] [Publisher Link]
[10] Tran-Dac-Thinh-Phanm et al., “EEG-Based Emotion Recognition by Convolutional Neural Network with Multi-Scale Kernels,” Sensors, vol. 21, no. 15, pp. 1-13, 2021.
[CrossRef] [Google Scholar] [Publisher Link]
[11] Ahmed S. Eltrass, and Noha H. Ghanem, “A New Automated Multi-Stage System of Non-Local Means and Multi-Kernel Adaptive Filtering Techniques for EEG Noise and Artifacts Suppression,” Journal of Neural Engineering, vol. 18, no. 3, 2021.
[CrossRef] [Google Scholar] [Publisher Link]
[12] P.V.V.S. Srinivas, and Pragnyaban Mishra, “A Novel Framework for Facial Emotion Recognition with Noisy and De Noisy Techniques Applied in Data Pre-Processing,” International Journal of System Assurance Engineering and Management, pp. 1-11, 2022.
[CrossRef] [Google Scholar] [Publisher Link]
[13] Haoshi Zhang et al., “A Robust Extraction Approach of Auditory Brainstem Response Using Adaptive Kalman Filtering Method,” IEEE Transactions on Biomedical Engineering, vol. 69, no. 12, pp. 3792-3802, 2022.
[CrossRef] [Google Scholar] [Publisher Link]
[14] Souvik Phadikar, Nidul Sinha, and Rajdeep Ghosh, “Automatic Eyeblink Artifact Removal from EEG Signal Using Wavelet Transform with Heuristically Optimized Threshold,” IEEE Journal of Biomedical and Health Informatics, vol. 25, no. 2, pp. 475-484, 2020.
[CrossRef] [Google Scholar] [Publisher Link]
[15] Md. Asadur Rahman et al., “Employing PCA and T-Statistical Approach for Feature Extraction and Classification of Emotion from Multichannel EEG Signal,” Egyptian Informatics Journal, vol. 21, no. 1, pp. 23-35, 2020.
[CrossRef] [Google Scholar] [Publisher Link]
[16] Qiang Gao et al., “EEG Based Emotion Recognition Using Fusion Feature Extraction Method,” Multimedia Tools and Applications, vol. 79, pp. 27057-27074, 2020.
[CrossRef] [Google Scholar] [Publisher Link]
[17] K. Saranya, and S. Jayanthy, “An Efficient AP-ANN-Based Multimethod Fusion Model to Detect Stress through EEG Signal Analysis,” Computational Intelligence and Neuroscience, vol. 2022, 2022.
[CrossRef] [Google Scholar] [Publisher Link]
[18] Md. Rabiul Islam et al., “Emotion Recognition from EEG Signal Focusing on Deep Learning and Shallow Learning Techniques,” IEEE Access, vol. 9, pp. 94601-94624, 2021.
[CrossRef] [Google Scholar] [Publisher Link]
[19] Patricia Becerra-Sánchez, Angelica Reyes-Munoz, and Antonio Guerrero-Ibañez, “Feature Selection Model Based on EEG Signals for Assessing the Cognitive Workload in Drivers,” Sensors, vol. 20, no. 20, pp. 1-25, 2020.
[CrossRef] [Google Scholar] [Publisher Link]
[20] Yong Jiao et al., “Multi-View Multi-Scale Optimization of Feature Representation for EEG Classification Improvement,” IEEE Transactions on Neural Systems and Rehabilitation Engineering, vol. 28, no. 12, pp. 2589-2597, 2020.
[CrossRef] [Google Scholar] [Publisher Link]
[21] Manosij Ghosh et al., “A Wrapper-Filter Feature Selection Technique Based on Ant Colony Optimization,” Neural Computing and Applications, vol. 32, pp. 7839-7857, 2020.
[CrossRef] [Google Scholar] [Publisher Link]
[22] Rosa A. García-Hernández et al., “Emotional State Detection Using Electroencephalogram Signals: A Genetic Algorithm Approach,” Applied Sciences, vol. 13, no. 11, pp. 1-15, 2023.
[CrossRef] [Google Scholar] [Publisher Link]
[23] Chunyuan Huang, “Recognition of Psychological Emotion by EEG Features,” Network Modeling Analysis in Health Informatics and Bioinformatics, vol. 10, pp. 1-11, 2021.
[CrossRef] [Google Scholar] [Publisher Link]
[24] Munaza Ramzan, and Suma Dawn, “Fused CNN-LSTM Deep Learning Emotion Recognition Model Using Electroencephalography Signals,” International Journal of Neuroscience, vol. 133, no. 6, pp. 587-597, 2023.
[CrossRef] [Google Scholar] [Publisher Link]
[25] Debashis Das Chakladar et al., “EEG-Based Mental Workload Estimation Using Deep BLSTM-LSTM Network and Evolutionary Algorithm,” Biomedical Signal Processing and Control, vol. 60, 2020.
[CrossRef] [Google Scholar] [Publisher Link]
[26] Rania Alhalaseh, and Suzan Alasasfeh, “Machine-Learning-Based Emotion Recognition System Using EEG Signals,” Computers, vol. 9, no. 4, pp. 1-15, 2020.
[CrossRef] [Google Scholar] [Publisher Link]
[27] Hao Chao, and Liang Dong, “Emotion Recognition Using Three-Dimensional Feature and Convolutional Neural Network from Multichannel EEG Signals,” IEEE Sensors Journal, vol. 21, no. 2, pp. 2024-2034, 2020.
[CrossRef] [Google Scholar] [Publisher Link]
[28] Aya Hassouneh, A.M. Mutawa, M. Murugappan, “Development of A Real-Time Emotion Recognition System Using Facial Expressions and EEG Based on Machine Learning and Deep Neural Network Methods,” Informatics in Medicine Unlocked, vol. 20, 2020.
[CrossRef] [Google Scholar] [Publisher Link]
[29] Bhuvaneshwari Chakravarthi et al., “EEG-Based Emotion Recognition Using Hybrid CNN and LSTM Classification,” Frontiers in Computational Neuroscience, vol. 16, 2022.
[CrossRef] [Google Scholar] [Publisher Link]