Prediction of Crime Rate in Diverse Environs Using Hybrid Classifier
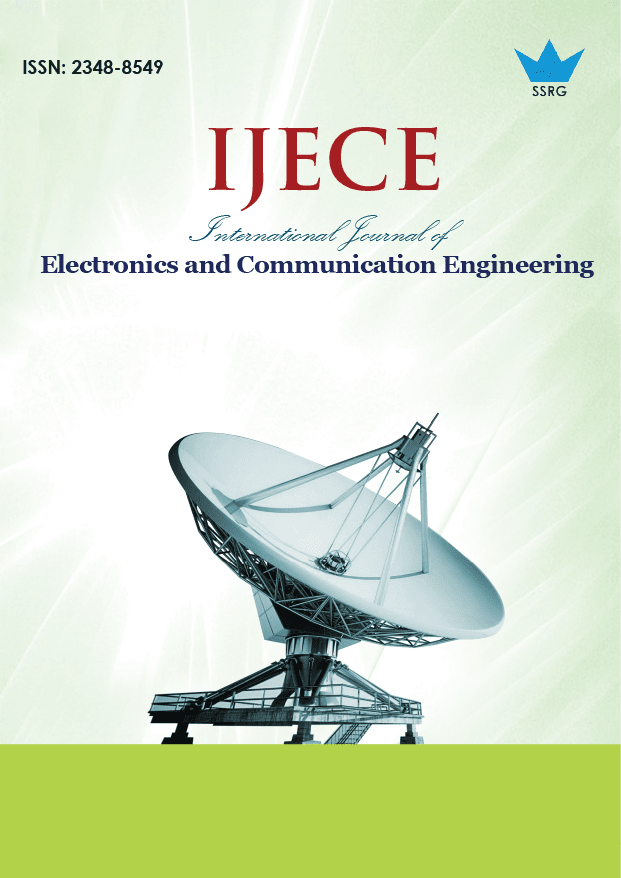
International Journal of Electronics and Communication Engineering |
© 2024 by SSRG - IJECE Journal |
Volume 11 Issue 2 |
Year of Publication : 2024 |
Authors : S. Santhosh, N. Sugitha |
How to Cite?
S. Santhosh, N. Sugitha, "Prediction of Crime Rate in Diverse Environs Using Hybrid Classifier," SSRG International Journal of Electronics and Communication Engineering, vol. 11, no. 2, pp. 80-91, 2024. Crossref, https://doi.org/10.14445/23488549/IJECE-V11I2P109
Abstract:
Crime is the fear and terror among the populace worldwide. Crime is an inherent component of the hazards we encounter daily. In recent times, the mass media has extensively covered numerous criminal incidents, including theft, rape and sexual offenses, robbery, murder, and kidnappings. Various works have been produced to understand the factors that lead to an individual committing a criminal act, the potential dangers involved, and strategies to prevent it. The crime computation technique aims to forecast crime rates, enabling police officers to avoid such incidents effectively. Based on this, a novel prediction approach utilizing a hybrid classifier is suggested. An evaluation of the proposed method was conducted using several criteria. The performance of this recently constructed hybrid prediction model is evaluated by comparing it with established models such as Genetic Algorithm, Particle Swarm Optimization, and Firefly Algorithm. Various performance measures, including error rate, sensitivity, specificity, precision, and execution time, are used for the comparison. Based on the results, this hybrid model is the most optimal crime prediction model compared to the other current models. The suggested approach is executed using the JAVA programming language.
Keywords:
Artificial Neural Network, Crime prediction, Hybrid classifier, Feature selection, Support Vector Machine,
Preprocessing, Prediction.
References:
[1] Anupam Mukherjee, and Anupam Ghosh, “Predictive Framework for Crime Data Analysis Using A Hybrid Logistic Regression-Support Vector Machine Based Ensemble Classifier Powered by CART (LR-SVM CART),” Multimedia Tools and Applications, vol. 82, pp. 35357-35377, 2023.
[CrossRef] [Google Scholar] [Publisher Link]
[2] Ruaa Mohammed Saeed, and Husam Ali Abdulmohsin, “A Study on Predicting Crime Rates through Machine Learning and Data Mining Using Text,” Journal of Intelligent Systems, vol. 32, no. 1, 2023.
[CrossRef] [Google Scholar] [Publisher Link]
[3] Angel Gonzalez-Prieto et al., “Hybrid Machine Learning Methods for Risk Assessment in Gender-Based Crime,” Knowledge-Based Systems, vol. 260, 2023.
[CrossRef] [Google Scholar] [Publisher Link]
[4] Vinothkumar K. et al., “Predicting High-Risk Areas for Crime Hotspot Using Hybrid KNN Machine Learning Framework,” 2023 5th International Conference on Inventive Research in Computing Applications (ICIRCA), Coimbatore, India, pp. 848-852, 2023.
[CrossRef] [Google Scholar] [Publisher Link]
[5] Ning Wang et al., “A Hybrid Ensemble Learning Method for the Identification of Gang-Related Arson Cases,” Knowledge-Based Systems, vol. 218, 2021.
[CrossRef] [Google Scholar] [Publisher Link]
[6] Bhavna Saini et al., “A Study of Lightweight Approaches to Analyze Crime Conditions in India,” Journal of Applied Security Research, vol. 18, no. 2, pp. 221-245, 2023.
[CrossRef] [Google Scholar] [Publisher Link]
[7] Wim Hardyns, and Robin Khalfa, “Predicting Crime across Cities and Regions: A Comparative Analysis of Predictive Modelling in Three Belgian Settings,” Applied Spatial Analysis and Policy, vol. 16, pp. 485-508, 2023.
[CrossRef] [Google Scholar] [Publisher Link]
[8] Rabia Musheer Aziz, Prajwal Sharma, and Aftab Hussain, “Machine Learning Algorithms for Crime Prediction under Indian Penal Code,” Annals of Data Science, pp. 1-32, 2022.
[CrossRef] [Google Scholar] [Publisher Link]
[9] Rabia Musheer Aziz, Aftab Hussain, and Prajwal Sharma, “Cognizable Crime Rate Prediction and Analysis under Indian Penal Code Using Deep Learning with Novel Optimization Approach,” Multimedia Tools and Applications, pp. 1-38, 2023.
[CrossRef] [Google Scholar] [Publisher Link]
[10] Pooja Mithoo, and Manoj Kumar, “Social Network Analysis for Crime Rate Detection Using Spizella Swarm Optimization Based BiLSTM Classifier,” Knowledge-Based Systems, vol. 269, 2023.
[CrossRef] [Google Scholar] [Publisher Link]
[11] Ali Raza et al., “Preventing Crimes through Gunshots Recognition Using Novel Feature Engineering and Meta-Learning Approach,” IEEE Access, vol. 11, pp. 103115-103131, 2023.
[CrossRef] [Google Scholar] [Publisher Link]
[12] Urvashi Gupta, and Rohit Sharma, “Analysis of Criminal Spatial Events in India Using Exploratory Data Analysis and Regression,” Computers and Electrical Engineering, vol. 109, Part A, 2023.
[CrossRef] [Google Scholar] [Publisher Link]
[13] S. Geetha, and Malathi K., “SVM vs Decision Tree Algorithm Cost Effective Comparison to Enhance Crime Detection and Prevention,” Journal of Survey in Fisheries Sciences, vol. 10, no. 1S, pp. 2814-2822, 2023.
[CrossRef] [Google Scholar] [Publisher Link]
[14] Monika, and Aruna Bhat, “DAC-BiNet: Twitter Crime Detection Using Deep Attention Convolutional Bi-Directional Aquila Optimal Network,” Multimedia Tools and Applications, 2023.
[CrossRef] [Google Scholar] [Publisher Link]
[15] Moruf Akin Adebowale, Khin T. Lwin, and M.A. Hossain, “Intelligent Phishing Detection Scheme Using Deep Learning Algorithms,” Journal of Enterprise Information Management, vol. 36, no. 3, pp. 747-766, 2023.
[CrossRef] [Google Scholar] [Publisher Link]
[16] Yifei Gong, Mengyan Dai, and Feng Gu, “CARESim: An Integrated Agent-Based Simulation Environment for Crime Analysis and Risk Evaluation (CARE),” Expert Systems with Applications, vol. 214, 2023.
[CrossRef] [Google Scholar] [Publisher Link]
[17] Anitha Thangasamy, Bose Sundan, and Logeswari Govindaraj, “A Novel Framework for DDoS Attacks Detection Using Hybrid LSTM Techniques,” Computer Systems Science & Engineering, vol. 45, no. 3, pp. 2553-2567, 2023.
[CrossRef] [Google Scholar] [Publisher Link]
[18] Balajee R.M., and Jayanthi Kannan M.K., “Intrusion Detection on AWS Cloud through Hybrid Deep Learning Algorithm,” Electronics, vol. 12, no. 6, pp. 1-21, 2023.
[CrossRef] [Google Scholar] [Publisher Link]