Developing a Hybrid Faster Recurrent Convolutional Neural Network with an Improved Weighted Artificial Bee Optimization Method for Grape Disease Detection at an Early Stage
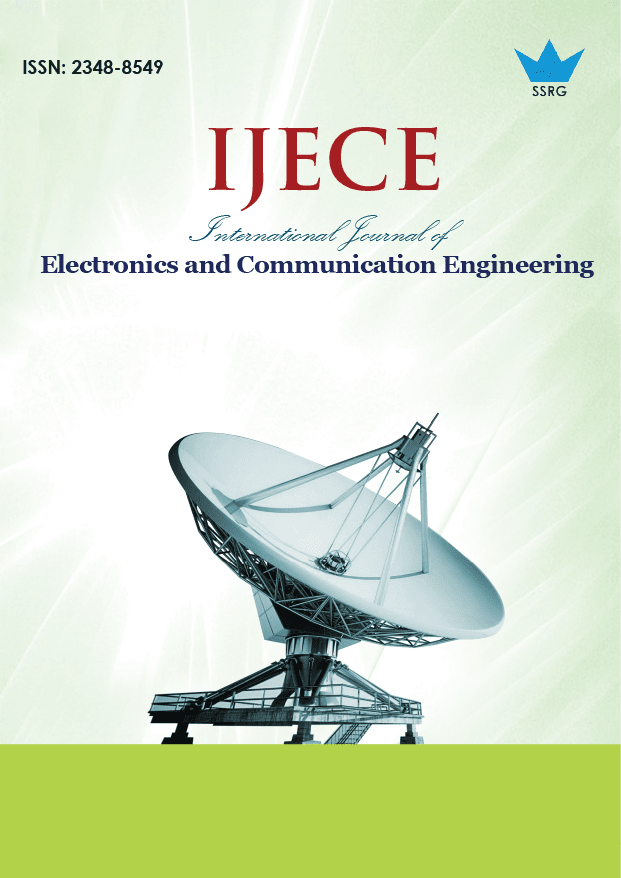
International Journal of Electronics and Communication Engineering |
© 2025 by SSRG - IJECE Journal |
Volume 12 Issue 1 |
Year of Publication : 2025 |
Authors : P. Jayapal, Pranesh Saminathan, Karthika, N. Nirmala Devi, K.R. Surendra |
How to Cite?
P. Jayapal, Pranesh Saminathan, Karthika, N. Nirmala Devi, K.R. Surendra, "Developing a Hybrid Faster Recurrent Convolutional Neural Network with an Improved Weighted Artificial Bee Optimization Method for Grape Disease Detection at an Early Stage," SSRG International Journal of Electronics and Communication Engineering, vol. 12, no. 1, pp. 161-173, 2025. Crossref, https://doi.org/10.14445/23488549/IJECE-V12I1P113
Abstract:
In India’s diverse range of crops, fruits play a crucial role in generating significant revenue for farmers. Among these fruits, grapes are extensively grown. However, grape plants are susceptible to various diseases affecting their fruits, stems, and leaves, ultimately impacting their yield. Grape disease detection at an early stage plays a crucial role in ensuring crop health and maximizing yield. To address this issue, early detection and effective treatment of these diseases are essential to ensure food safety. This study focuses on analyzing different methods for diagnosing and classifying diseases that affect grapevines, with particular emphasis on grape leaf diseases. Monitoring the condition of grape leaves provides valuable insights into the overall health of the grape plants. The research aims to provide a comprehensive overview of techniques used for identifying and categorizing these diseases. Automated disease detection algorithms are proposed to enhance diagnosis accuracy and enable timely control actions. Image processing, a widely used method, is endorsed for leaf disease identification and classification in plants. In this study, we propose a novel approach by integrating a hybrid Faster Recurrent Convolutional Neural Network (FRCNN) with an improved Weighted Artificial Bee Optimization (WABO) method for efficient grape disease detection. The FRCNN architecture combines the strengths of recurrent and convolutional neural networks to capture both spatial and temporal information from grape images. Additionally, the WABO method enhances the training process by optimizing the network’s weights to improve detection accuracy. Experiments conducted on a large-scale dataset show that the proposed approach is more effective than current approaches in terms of computational efficiency and detection accuracy when it comes to correctly diagnosing grape diseases early on. The proposed framework has a lot of potential for practical uses in vineyard management and precision agriculture.
Keywords:
Grape disease detection, Early stage detection, Hybrid Faster Recurrent Convolutional Neural Network, Weighted Artificial Bee Optimization, Precision agriculture, Vineyard management, Computational efficiency, Detection accuracy.
References:
[1] Chittabarni Sarkar et al., “Leaf Disease Detection Using Machine Learning and Deep Learning: Review and Challenges,” Applied Soft
Computing, vol. 145, 2023.
[CrossRef] [Google Scholar] [Publisher Link]
[2] Anuradha Chug et al., “A Novel Framework for Image-Based Plant Disease Detection Using Hybrid Deep Learning Approach,” Soft Computing, vol. 27, pp. 13613-13638, 2023.
[CrossRef] [Google Scholar] [Publisher Link]
[3] Imtiaz Ahmed, and Pramod Kumar Yadav, “Plant Disease Detection Using Machine Learning Approaches,” Expert Systems, vol. 40, no. 5, 2023.
[CrossRef] [Google Scholar] [Publisher Link]
[4] Emmanuel Moupojou et al., “FieldPlant: A Dataset of Field Plant Images for Plant Disease Detection and Classification with Deep Learning,” IEEE Access, vol. 11, pp. 35398-35410, 2023.
[CrossRef] [Google Scholar] [Publisher Link]
[5] Aanis Ahmad, Dharmendra Saraswat, and Aly El Gamal, “A Survey on Using Deep Learning Techniques for Plant Disease Diagnosis and Recommendations for Development of Appropriate Tools,” Smart Agricultural Technology, vol. 3, pp. 1-13, 2023.
[CrossRef] [Google Scholar] [Publisher Link]
[6] Aanis Ahmad, Aly El Gamal, and Dharmendra Saraswat, “Toward Generalization of Deep Learning-based Plant Disease Identification under Controlled and Field Conditions,” IEEE Access, vol. 11, pp. 9042-9057, 2023.
[CrossRef] [Google Scholar] [Publisher Link]
[7] Amirtha Haridasan, Jeena Thomas, and Ebin Deni Raj, “Deep Learning System for Paddy Plant Disease Detection and Classification,” Environmental Monitoring and Assessment, vol. 195, 2023.
[CrossRef] [Google Scholar] [Publisher Link]
[8] Adesh V. Panchal et al., “Image-based Plant Diseases Detection Using Deep Learning,” Materials Today: Proceedings, vol. 80, no. 3, pp. 3500-3506, 2023.
[CrossRef] [Google Scholar] [Publisher Link]
[9] Imtiaz Ahmed, and Pramod Kumar Yadav, “A Systematic Analysis of Machine Learning and Deep Learning Based Approaches for Identifying and Diagnosing Plant Diseases,” Sustainable Operations and Computers, vol. 4, pp. 96-104, 2023.
[CrossRef] [Google Scholar] [Publisher Link]
[10] Meenakshi Aggarwal et al., “Pre-Trained Deep Neural Network-Based Features Selection Supported Machine Learning for Rice Leaf Disease Classification,” Agriculture, vol. 13, no. 5, pp. 1-24, 2023.
[CrossRef] [Google Scholar] [Publisher Link]
[11] Tej Bahadur Shahi et al., “Recent Advances in Crop Disease Detection Using UAV and Deep Learning Techniques,” Remote Sensing, vol. 15, no. 9, pp. 1-29, 2023.
[CrossRef] [Google Scholar] [Publisher Link]
[12] Vikram Rajpoot, Akhilesh Tiwari, and Anand Singh Jalal, “Automatic Early Detection of Rice Leaf Diseases Using Hybrid Deep Learning and Machine Learning Methods,” Multimedia Tools and Applications, vol. 82, pp. 36091-36117, 2023.
[CrossRef] [Google Scholar] [Publisher Link]
[13] Rina Bora, Deepa Parasar, and Shrikant Charhate, “A Detection of Tomato Plant Diseases Using Deep Learning MNDLNN Classifier,” Signal, Image and Video Processing, vol. 17, pp. 3255-3263, 2023.
[CrossRef] [Google Scholar] [Publisher Link]
[14] Muhammad Shoaib et al., “An Advanced Deep Learning Models-Based Plant Disease Detection: A Review of Recent Research,” Frontiers in Plant Science, vol. 14, pp. 1-22, 2023.
[CrossRef] [Google Scholar] [Publisher Link]
[15] Jameer Kotwal, Ramgopal Kashyap, and Shafi Pathan, “Agricultural Plant Diseases Identification: from Traditional Approach to Deep Learning,” Materials Today: Proceedings, vol. 80, pp. 344-356, 2023.
[CrossRef] [Google Scholar] [Publisher Link]
[16] Rabbia Mahum et al., “A Novel Framework for Potato Leaf Disease Detection Using an Efficient Deep Learning Model,” Human and Ecological Risk Assessment: An International Journal, vol. 29, no. 2, pp. 303-326, 2023.
[CrossRef] [Google Scholar] [Publisher Link]
[17] Manowarul Islam et al., “DeepCrop: Deep Learning-Based Crop Disease Prediction with Web Application,” Journal of Agriculture and Food Research, vol. 14, pp. 1-11, 2023.
[CrossRef] [Google Scholar] [Publisher Link]
[18] Yousef Methkal Abd Algani et al., “Leaf Disease Identification and Classification Using Optimized Deep Learning,” Measurement: Sensors, vol. 25, pp. 1-6, 2023.
[CrossRef] [Google Scholar] [Publisher Link]
[19] Junde Chen et al., “Stacking Ensemble Model of Deep Learning for Plant Disease Recognition,” Journal of Ambient Intelligence and Humanized Computing, vol. 14, pp. 12359-12372, 2023.
[CrossRef] [Google Scholar] [Publisher Link]
[20] Vikki Binnar, and Sanjeev Sharma, “Plant Leaf Diseases Detection Using Deep Learning Algorithms,” Machine Learning, Image Processing, Network Security and Data Sciences: Select Proceedings of 3rd International Conference on MIND 2021, pp. 217-228, 2023.
[CrossRef] [Google Scholar] [Publisher Link]
[21] Tausif Mallick et al., “Deep Learning-Based Automated Disease Detection and Classification Model for Precision Agriculture,” Multimedia Tools and Applications, vol. 82, pp. 12017-12041, 2023.
[CrossRef] [Google Scholar] [Publisher Link]
[22] Laixiang Xu et al., “Wheat Leaf Disease Identification Based on Deep Learning Algorithms,” Physiological and Molecular Plant Pathology, vol. 123, 2023.
[CrossRef] [Google Scholar] [Publisher Link]
[23] Dians Susan Joseph, Pranav M. Pawar, and Rahul Pramanik, “Intelligent Plant Disease Diagnosis Using Convolutional Neural Network: A Review,” Multimedia Tools and Applications, vol. 82, pp. 21415-21481, 2023.
[CrossRef] [Google Scholar] [Publisher Link]
[24] Mike O. Ojo, and Azlan Zahid, “Improving Deep Learning Classifiers Performance Via Preprocessing and Class Imbalance Approaches in a Plant Disease Detection Pipeline,” Agronomy, vol. 13, no. 3, pp. 1-18, 2023.
[CrossRef] [Google Scholar] [Publisher Link]
[25] Sakib Hossain Shovon et al., “PlantDet: A Robust Multi-model Ensemble Method Based on Deep Learning for Plant Disease Detection,” IEEE Access, vol. 11, pp. 34846-34859, 2023.
[CrossRef] [Google Scholar] [Publisher Link]
[26] Seyed Mohamad Javidan et al., “Diagnosis of Grape Leaf Diseases Using Automatic K-Means Clustering and Machine Learning,” Smart Agricultural Technology, vol. 3, pp. 1-14, 2023.
[CrossRef] [Google Scholar] [Publisher Link]
[27] Olfa Mzoughi, and Itheri Yahiaoui, “Deep Learning-Based Segmentation for Disease Identification,” Ecological Informatics, vol. 75, 2023.
[CrossRef] [Google Scholar] [Publisher Link]
[28] Kemal Adem, Mehmet Metin Ozguven, and Ziya Altas, “A Sugar Beet Leaf Disease Classification Method based on Image Processing and Deep Learning,” Multimedia Tools and Applications, vol. 82, pp. 12577-12594, 2023.
[CrossRef] [Google Scholar] [Publisher Link]
[29] S. Sivasankaran, K. Jagan Mohan, and G. Mohammed Nazer, “Soil Moisture Quantity Prediction using Optimized Deep Learning Supported Model for Sustainable Cultivation of Groundnut Plant,” Natural Volatiles & Essential Oils Journal, vol. 8, no. 5, pp. 13184-13205, 2021.
[Google Scholar] [Publisher Link]