Innovative Toxicity Detection in Metal Oxide Nanoparticles Using 1-D CNN with Transformer Architecture
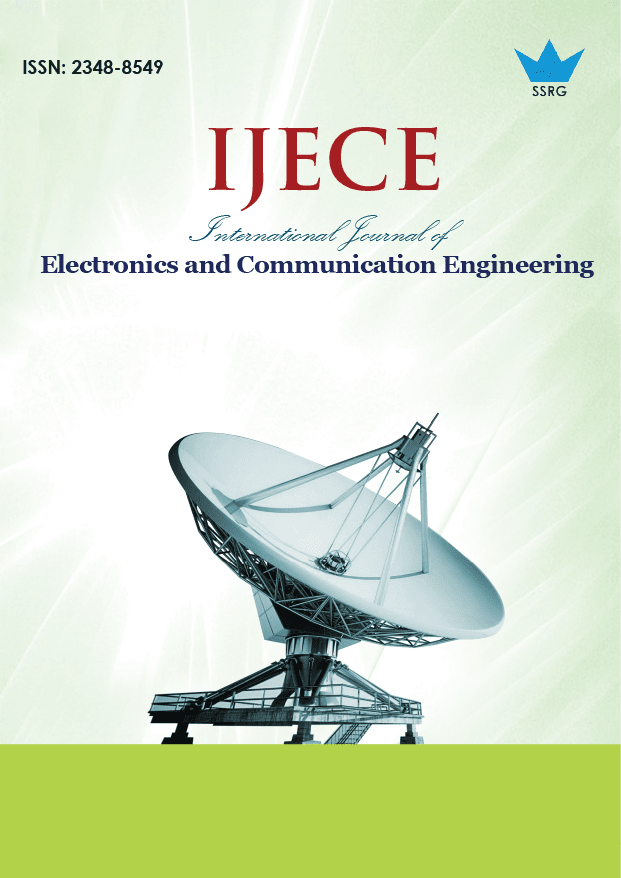
International Journal of Electronics and Communication Engineering |
© 2025 by SSRG - IJECE Journal |
Volume 12 Issue 1 |
Year of Publication : 2025 |
Authors : V.S. Nisha, R.S. Rimal Isaac |
How to Cite?
V.S. Nisha, R.S. Rimal Isaac, "Innovative Toxicity Detection in Metal Oxide Nanoparticles Using 1-D CNN with Transformer Architecture," SSRG International Journal of Electronics and Communication Engineering, vol. 12, no. 1, pp. 271-286, 2025. Crossref, https://doi.org/10.14445/23488549/IJECE-V12I1P121
Abstract:
Nanotechnology entails the manipulation of matter at the nanoscale to generate materials with distinctive features and is employed in several fields, such as medicine, electronics, and environmental science. Engineered nanoparticles at this scale provide improved functioning, although they also raise concerns about their potential toxicity to biological systems and ecosystems. Existing toxicity detection methods frequently depend on conventional experimental techniques or machine learning models that emphasize physicochemical properties, resulting in constraints such as limited sample sizes, overfitting, and insufficient interpretability. These approaches often inadequately represent the intricate interactions between nanoparticles and biological entities, leading to incorrect predictions. To tackle these problems, we propose an innovative method employing a 1-D Convolutional Neural Network (CNN) combined with transformers aimed at enhancing the predicted accuracy of Nanoparticle (NP) toxicity evaluations. The evaluation of the proposed design demonstrates highly excellent classification outcomes, with the model attaining an impressive accuracy of 96.61%. Every detected toxic case was, in fact toxic, as evidenced by the precision of 100% and the recall of 92.85% shows how sensitive the model is to real toxic events. The balanced performance is further demonstrated by an F1 score of 96.29%, highlighting the model's efficacy in handling imbalanced toxicity data. Comparisons with existing approaches highlight the superiority of the proposed method, providing essential insights for NP toxicity prediction and facilitating the production of safer nanomaterials for various applications.
Keywords:
Nanoparticle, Toxicity, Transformer, 1-D CNN, Metal oxide, Nanotechnology, Optics.
References:
[1] Muhammad Rafique et al., Chapter 1 - History and Fundamentals of Nanoscience and Nanotechnology, Nanotechnology and Photocatalysis for Environmental Applications: Micro and Nano Technologies, Elsevier, pp. 1-25, 2020.
[CrossRef] [Google Scholar] [Publisher Link]
[2] Sharon Mitchell et al., “Nanoscale Engineering of Catalytic Materials for Sustainable Technologies,” Nature Nanotechnology, vol. 16, no. 2, pp. 129-139, 2021.
[CrossRef] [Google Scholar] [Publisher Link]
[3] Safia Hameed et al., “Cannabis Sativa-Mediated Synthesis of Gold Nanoparticles and Their Biomedical Properties,” Bioinspired, Biomimetic and Nanobiomaterials, vol. 9, no. 2, pp. 95-102, 2020.
[CrossRef] [Google Scholar] [Publisher Link]
[4] Ibrahim Khan, Khalid Saeed, and Idrees Khan, “Nanoparticles: Properties, Applications and Toxicities,” Arabian Journal of Chemistry, vol. 12. no. 7, pp. 908-931, 2019.
[CrossRef] [Google Scholar] [Publisher Link]
[5] C.M. Vineeth Kumar et al., “The Impact of Engineered Nanomaterials on the Environment: Release Mechanism, Toxicity, Transformation, and Remediation,” Environmental Research, vol. 212, no. 2, 2022.
[CrossRef] [Google Scholar] [Publisher Link]
[6] Murthy S. Chavali, and Maria P. Nikolova, “Metal Oxide Nanoparticles and their Applications in Nanotechnology,” SN Applied Sciences, vol. 1, no. 6, pp. 1-30, 2019.
[CrossRef] [Google Scholar] [Publisher Link]
[7] Zhongjie Yu et al., “Reactive Oxygen Species-Related Nanoparticle Toxicity in the Biomedical Field,” Nanoscale Research Letters, vol. 15, no. 1, pp. 1-14, 2020.
[CrossRef] [Google Scholar] [Publisher Link]
[8] Furkan Eker et al., “A Comprehensive Review of Nanoparticles: from Classification to Application and Toxicity,” Molecules, vol. 29, no. 15, pp. 1-83, 2024.
[CrossRef] [Google Scholar] [Publisher Link]
[9] Stefania Gottardo et al., “Towards Safe and Sustainable Innovation in Nanotechnology: State-of-play for Smart Nanomaterials,” NanoImpact, vol. 21, pp. 1-10, 2021.
[CrossRef] [Google Scholar] [Publisher Link]
[10] Ajay Vikram Singh et al., “Artificial Intelligence and Machine Learning in Computational Nanotoxicology: Unlocking and Empowering Nanomedicine,” Advanced Healthcare Materials, vol. 9, no. 17, pp. 1-19, 2020.
[CrossRef] [Google Scholar] [Publisher Link]
[11] Iqra Yousaf, “AI and Machine Learning Approaches for Predicting Nanoparticles Toxicity the Critical Role of Physiochemical Properties,” arXiv, pp. 1-20, 2024.
[CrossRef] [Google Scholar] [Publisher Link]
[12] Yunchi Zhou et al., “Using Machine Learning to Predict Adverse Effects of Metallic Nanomaterials to Various Aquatic Organisms,” Environmental Science & Technology, vol. 57, no. 46, pp. 17786-17795, 2023.
[CrossRef] [Google Scholar] [Publisher Link]
[13] Yang Huang et al., “Use of Dissociation Degree in Lysosomes to Predict Metal Oxide Nanoparticle Toxicity in Immune Cells: Machine Learning Boosts Nano-Safety Assessment,” Environment International, vol. 164, pp. 1-12, 2022.
[CrossRef] [Google Scholar] [Publisher Link]
[14] Nilesh Anantha Subramanian, and Ashok Palaniappan, “NanoTox: Development of a Parsimonious in Silico Model for Toxicity Assessment of Metal-Oxide Nanoparticles Using Physicochemical Features,” ACS omega, vol. 6, no. 17, pp. 11729-11739, 2021.
[CrossRef] [Google Scholar] [Publisher Link]
[15] Farooq Ahmad, Asif Mahmood, and Tahir Muhmood, “Machine Learning-integrated Omics for the Risk and Safety Assessment of Nanomaterials,” Biomaterials science, vol. 9, no. 5, pp. 1598-1608, 2021.
[CrossRef] [Google Scholar] [Publisher Link]
[16] Hengjie Yu, Zhilin Zhao, and Fang Cheng, “Predicting and Investigating Cytotoxicity of Nanoparticles by Translucent Machine Learning,” Chemosphere, vol. 276, 2021.
[CrossRef] [Google Scholar] [Publisher Link]
[17] Jossana A. Damasco et al., “Understanding Nanoparticle Toxicity to Direct a Safe-by-Design Approach in Cancer Nanomedicine,” Nanomaterials, vol. 10, no. 11, pp. 1-41, 2020.
[CrossRef] [Google Scholar] [Publisher Link]
[18] Bhavna Saini, Sumit Srivastava, and A.K. Bajpai, “Deep CNN Model for Nanotoxicity Classification Using Microscopic Images,” International Journal of Advances in Soft Computing and its Applications, vol. 12, no. 2, pp. 1-22, 2020.
[Google Scholar] [Publisher Link]
[19] Furui Wang, Gangbing Song, and Yi-Lung Mo, “Shear Loading Detection of Through Bolts in Bridge Structures Using a Percussion-Based One-Dimensional Memory-Augmented Convolutional Neural Network,” ComputerāAided Civil and Infrastructure Engineering, vol. 36, no. 3, pp. 289-301, 2021.
[CrossRef] [Google Scholar] [Publisher Link]
[20] Ashish Vaswani, “Attention is all You Need,” 31st Conference on Neural Information Processing Systems, Long Beach, CA, USA, pp. 1-11, 2017.
[Google Scholar] [Publisher Link]
[21] Hans J.P. Marvin et al., “Application of Bayesian Networks for Hazard Ranking of Nanomaterials to Support Human Health Risk Assessment,” Nanotoxicology, vol. 11, no. 1, pp. 123-133, 2017.
[CrossRef] [Google Scholar] [Publisher Link]