Performance Analysis of SVD-DSK-MIMO-OFDM System over Time Frequency Selective Fading Channels
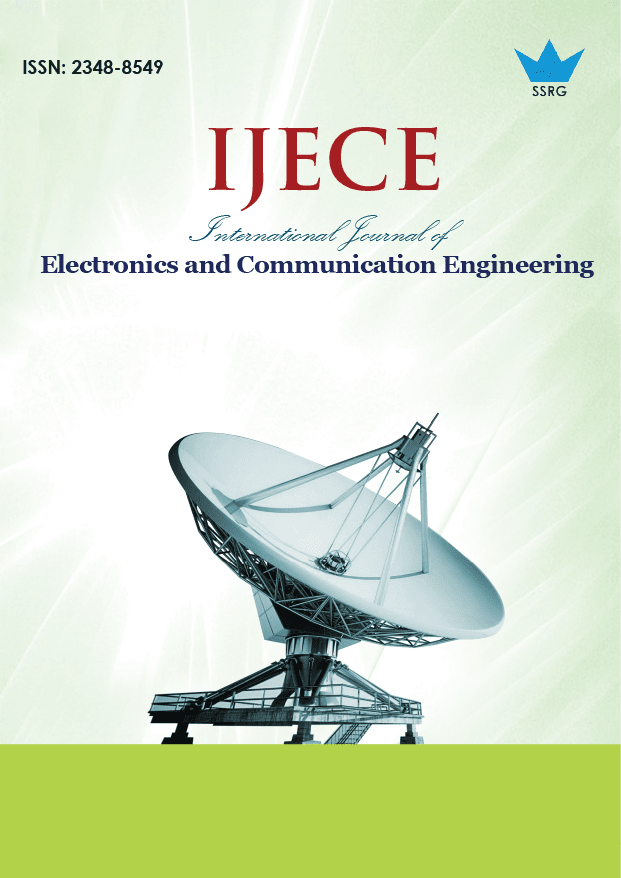
International Journal of Electronics and Communication Engineering |
© 2025 by SSRG - IJECE Journal |
Volume 12 Issue 4 |
Year of Publication : 2025 |
Authors : Kasetty Lakshminarasimha, M. Srilatha, Majeti Venkata Sireesha, A Sravanthi Peddinti, R. Anil Kumar, Kapula Kalyani |
How to Cite?
Kasetty Lakshminarasimha, M. Srilatha, Majeti Venkata Sireesha, A Sravanthi Peddinti, R. Anil Kumar, Kapula Kalyani, "Performance Analysis of SVD-DSK-MIMO-OFDM System over Time Frequency Selective Fading Channels," SSRG International Journal of Electronics and Communication Engineering, vol. 12, no. 4, pp. 167-173, 2025. Crossref, https://doi.org/10.14445/23488549/IJECE-V12I4P116
Abstract:
The paper presents a unique approach to improving the dependability of OFDM-DSK systems for communication over doubly selective fading channels. The proposed solution uses Singular Value Decomposition (SVD) to divide the channel into distinct sub-channels, each with its own transmission characteristics, using available Channel State Information (CSI). It improves performance by multiplying the symbol matrix by the appropriate singular vectors at the transmitter. The Signal-to-Noise Ratio (SNR) of the received symbols can be increased using this configuration to allocate the reference chaotic sequence’s strongest sub-channel. It is linked to the highest singular value. Consequently, the system's Bit Error Rate (BER) is reduced, increasing its dependability. Here, strategically rearrange symbols according to their weights, which ideally distributes symbols to sub-channels according to their strengths. It helps to further reduce the BER. The singular-vector pre-coded OFDM-DSK (SVP-OFDM-DSK) combined with the Multiple-Input Multiple-Output (MIMO) and symbol reallocation technique. The system’s reliability is greatly increased by this approach, which helps to increase SNR in doubly selective fading channels. Compared to traditional techniques, simulation results are validated on MATLAB and SVP-OFDM-DSK yields reduced BER with and without symbol permutation.
Keywords:
Bit Error Rate, Channel state information, Multiple-input multiple-output, Signal-to-Noise Ratio, Singular value decomposition.
References:
[1] Zhaofeng Liu et al., “A Reliable Singular-Vector Pre-Coded OFDM-DCSK System Over Doubly Selective Fading Channels,” IEEE Wireless Communications Letters, vol. 13, no. 5, pp. 1453-1457, 2024.
[CrossRef] [Google Scholar] [Publisher Link]
[2] Tianyu Zhao, and Feng Li, “Variational-Autoencoder Signal Detection for MIMO-OFDM-IM,” Digital Signal Processing, vol. 118, 2021.
[CrossRef] [Google Scholar] [Publisher Link]
[3] Xihong Chen, Qiang Liu, and Maokai Hu, “Performance Analysis of MIMO-OFDM Systems on Nakagami-m Fading Channels,” 2010 2nd International Conference on Future Computer and Communication, Wuhan, China, vol. 3, pp. V3-396-V3-400, 2010.
[CrossRef] [Google Scholar] [Publisher Link]
[4] R. Anil Kumar et al., “Symbol Interference Cancellation in MIMO-GFDM Using Singular Value Decomposition Technique for 5G,” International Journal of Electrical and Electronics Research, vol. 12, no. 4, pp. 1324-1331, 2024.
[CrossRef] [Google Scholar] [Publisher Link]
[5] Pasupuleti Sai Deepthi et al., “Review of 5G Communications Over OFDM and GFDM,” Proceedings of the 3rd International Conference on Communications and Cyber Physical Engineering, pp. 861-869, 2020.
[CrossRef] [Google Scholar] [Publisher Link]
[6] R. Anil Kumar et al., “Performance of MIMO-GFDM Scheme for Future Wireless Communications,” 2024 International Conference on Knowledge Engineering and Communication Systems, Chikkaballapur, India, pp. 1-6, 2024.
[CrossRef] [Google Scholar] [Publisher Link]
[7] Kun Chen-Hu, Yong Liu, and Ana García Armada, “Non-Coherent Massive MIMO-OFDM Down-Link Based on Differential Modulation,” IEEE Transactions on Vehicular Technology, vol. 69, no. 10, pp. 11281-11294, 2020.
[CrossRef] [Google Scholar] [Publisher Link]
[8] Tomohisa Wada et al., “A Denoising Autoencoder Based Wireless Channel Transfer Function Estimator for OFDM Communication System,” 2019 International Conference on Artificial Intelligence in Information and Communication, Okinawa, Japan, pp. 530-533, 2019.
[CrossRef] [Google Scholar] [Publisher Link]
[9] Ezmin Abdullah et al., “Deep Learning Based Asymmetrical Autoencoder for PAPR Reduction of CP-OFDM Systems,” Engineering Science and Technology, an International Journal, vol. 50, pp. 1-15, 2024.
[CrossRef] [Google Scholar] [Publisher Link]
[10] K. Mohammed Asif, and Aditya Trivedi, “OFDM Ensemble Autoencoder Using CNN and SPSA for End-to-End Learning Communication Systems,” 2020 IEEE 4th Conference on Information & Communication Technology, Chennai, India, pp. 1-6, 2020.
[CrossRef] [Google Scholar] [Publisher Link]
[11] Saeid K. Dehkordi et al., “Variational Autoencoder-Based Parameter Estimation in Beam-Space OFDM Integrated Sensing and Communication,” GLOBECOM 2023 - 2023 IEEE Global Communications Conference, Kuala Lumpur, Malaysia, pp. 3904-3909, 2023.
[CrossRef] [Google Scholar] [Publisher Link]
[12] Ying Han et al., “A Novel Multi-Impairment Compensation Scheme Based on Deep Autoencoder for CO-OFDM System,” 2020 Opto-Electronics and Communications Conference, Taipei, Taiwan, pp. 1-3, 2020.
[CrossRef] [Google Scholar] [Publisher Link]
[13] Alexander Felix et al., “OFDM-Autoencoder for End-to-End Learning of Communications Systems,” 2018 IEEE 19th International Workshop on Signal Processing Advances in Wireless Communications, Kalamata, Greece, pp. 1-5, 2018.
[CrossRef] [Google Scholar] [Publisher Link]
[14] Lina Shi et al., “PAPR Reduction Based on Deep Autoencoder for VLC DCO-OFDM System,” 2019 IEEE International Symposium on Broadband Multimedia Systems and Broadcasting, Jeju, Korea (South), pp. 1-4, 2019.
[CrossRef] [Google Scholar] [Publisher Link]
[15] Priti G. Pachpande et al., “Autoencoder Model for OFDM-Based Optical Wireless Communication,” Signal Processing in Photonic Communications, pp. 1-2, 2019.
[CrossRef] [Google Scholar] [Publisher Link]
[16] Monette H. Khadr, Anya E. Ross, and Hany Elgala, “Autoencoder Model for OFDM-Based Optical Wireless Communications Systems Employing Augmented Communications,” Signal Processing in Photonic Communications, 2021.
[CrossRef] [Google Scholar] [Publisher Link]
[17] Dongbo Li et al., “Autoencoder-Based OFDM for Agricultural Image Transmission,” 2022 Tenth International Conference on Advanced Cloud and Big Data, Guilin, China, pp. 157-162, 2022.
[CrossRef] [Google Scholar] [Publisher Link]
[18] Chiharu Nishide, and Masaya Ohta, “Complexity Suppression of AutoEncoder for PAPR Reduction of OFDM Signals,” 2022 IEEE 11th Global Conference on Consumer Electronics, Osaka, Japan, pp. 194-195, 2022.
[CrossRef] [Google Scholar] [Publisher Link]
[19] Seizan Tsugawa et al., “Scalability in Autoencoder-Based OFDM Communication System,” 2023 IEEE International Conference on Computer Vision and Machine Intelligence, Gwalior, India, pp. 1-5, 2023.
[CrossRef] [Google Scholar] [Publisher Link]
[20] Wenshan Jiang et al., “End-to-End Learning Based Bit-Wise Autoencoder for Optical OFDM Communication System,” 2021 Asia Communications and Photonics Conference, Shanghai, China, pp. 1-3, 2021.
[Google Scholar] [Publisher Link]
[21] Song Miao et al., “An Autoencoder-OFDM Power Line Carrier Communication System Based on Deep Learning,” 2021 IEEE 5th Conference on Energy Internet and Energy System Integration, Taiyuan, China, pp. 508-513, 2021.
[CrossRef] [Google Scholar] [Publisher Link]