Bin Ratio-Based Histogram Distances and their Application to Image Classification
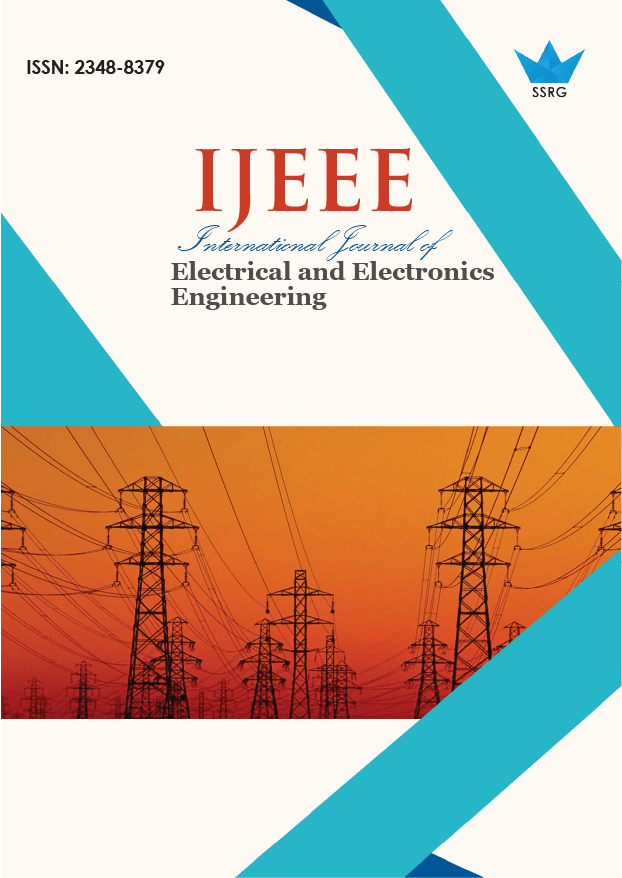
International Journal of Electrical and Electronics Engineering |
© 2017 by SSRG - IJEEE Journal |
Volume 4 Issue 8 |
Year of Publication : 2017 |
Authors : Sonam Agarwal, Prof. Ujwal. Harode |
How to Cite?
Sonam Agarwal, Prof. Ujwal. Harode, "Bin Ratio-Based Histogram Distances and their Application to Image Classification," SSRG International Journal of Electrical and Electronics Engineering, vol. 4, no. 8, pp. 29-32, 2017. Crossref, https://doi.org/10.14445/23488379/IJEEE-V4I8P105
Abstract:
Image representation in form of Histogram plays an important role in image classification, action and pattern recognition. Differences i.e. distances can be well identified and studied using a histogram plot. It is the representation in the graphical form of the plot of tonal distribution of digital image. It is the plot of pixels against tonal value. One can judge the tonal distribution in an image just by looking the Histogram plot. When it comes to the local Patches i.e. the distribution of local areas of image, Quantization of patches by sub-histogram is good approach. Histogram followed by sub-histogram and different distance classifier along with SVM gives good accuracy level. There are many modern technologies coming up like expert system classifier, ANN and DTC that maximize the accuracy level. The report will summarize the advance classification approaches that are used to improve accuracy levels.
Keywords:
SVM, DTC, Artificial Neural Network, Expert System Classifier.
References:
1. R. M. Haralick., “Digital step edges from zero crossing of the second directional derivatives,” IEEE Trans. Pattern Anal. Machine Intell., vol. PAMI-6, no . 1, pp. 58-68, Jan. 1984.
2. W. Härdle, R.A. Moro, D. Schäfer, March 2004, Rating Companies with Support Vector Machines, Discussion Paper Nr. 416, DIW Berlin. 3.
4. J. Zhang, M. Marszalek, S. Lazebnik and C. Schmid. Local features and kernels for classification of texture and object categories: A comprehensive study. IJCV, 2007
5. S. Mukherjee, E. Osuna, and F. Girosi. Nonlinear prediction of chaotic time series using a support vector machine. In J. Principe, L. Gile, N. Morgan, and E. Wilson, editors, Neural Networks for Signal Processing VII
6. E. Diday and J. V. Moreau, "Learning hierarchical clustering from examples -- Applications to the adaptive construction of dissimilarity indices," Pattern Recognition Lett.," 223-230 (1986)
7. R.O. Duda and P.E. Hart, Pattern classification and scene analysis, Wiley-Interscience, 1973.
8. P. Fletcher and M.j.D. Powell,"A rapid decent method for minimization," Computer Journal, Vol.6, ISS.2, 163-168 (1963).
9. PubPDF - Full Text Article Bin Ratio-Based Histogram Distances and Their Application to Image Classification. IEEE Transactions on Pattern Analysis and Machine Intelligence (Volume: 36, Issue: 12, Dec. 1 2014 )
10. A. Agarwal and B. Triggs,“Hyperfeatures -Multilevel Local Coding for Visual Recognition,”INRIA Research Report RR-5655, 2006.
11. A. C. Berg, T. L. Berg, and J. Malik,“Shape Matching and Object Recognition Using Low Distortion Correspondences,”in Proc. of ComputerVision and Pattern Recognition, vol.1, pp. 26-33, 2005.
12. D. Cai, X. He,and J. Han,“Efficient Partial Matching Analysis viaSpectral Regression,”In Proc. of IEEE International Conference on DataMining, pp. 427-432, Oct. 2007.