Performance of Controllers for Non Linear Process
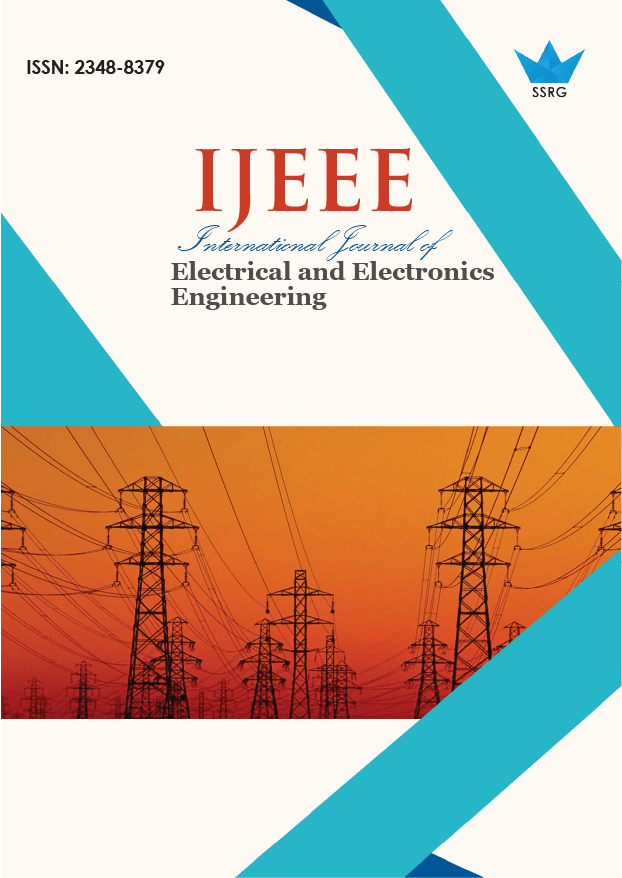
International Journal of Electrical and Electronics Engineering |
© 2019 by SSRG - IJEEE Journal |
Volume 6 Issue 11 |
Year of Publication : 2019 |
Authors : Mrs. A. Durgadevi, Dr. K.Dhayalini, Mr. R.Saran Raj |
How to Cite?
Mrs. A. Durgadevi, Dr. K.Dhayalini, Mr. R.Saran Raj, "Performance of Controllers for Non Linear Process," SSRG International Journal of Electrical and Electronics Engineering, vol. 6, no. 11, pp. 1-6, 2019. Crossref, https://doi.org/10.14445/23488379/IJEEE-V6I11P101
Abstract:
Non-linear process control is a complex task in process industries. Due to the persistently changing cross section and non-linearity of the tank a spherical tank provides a demanding problem for the level control. In this paper the model of a spherical tank system is derived as First Order Plus Dead Time (FOPDT) from the open loop response of real time setup of the system using Lab VIEW software. The intention of this project is to preserve the level inside the process tank at preferred value. The Proportional- Integral (PI) controller is designed to direct the level of the water in spherical tank. But for a non-linear system the same PI controller will give different responses at different operating regions. Hence there is a need for non-linear controller (Model Predictive Controller- MPC) to work in this non-linear region. But the complex control problem has led to use Neural Network (NN) in MPC. The advantage of Neural Network Predictive Controller (NNPC) is that an precise depiction of the process can be obtained by training the NN. The controllers are designed and the performances of these controllers (PI controller, MPC and NNPC) are compared for set point tracking and disturbance rejection using MATLAB. From the results it is inferred that NNPC gives minimum error and better tracking performance
Keywords:
Model Predictive Controller, Neural Network Predictive Controller, Proportional – Integral controller, Spherical tank.
References:
[1] Reshma.K. V and Dr. S. Sumathi, “Control of Non Linear Spherical Tank Level Process”, International Journal for Research in Applied Science & Engineering Technology, Vol. 3, pp. 448-453, 2015.
[2] M. Kalyan Chakravarthi, Nithya Venkatesan, “Labview Based Tuning Of PI Controllers For A Real Time Non Linear Process”, Journal of Theoretical and Applied Information Technology, vol. 68, pp. 579-585, 2014.
[3] Mrs. A. Asuntha and Dr Andy Srinivasan, “Tuning Of Controllers for Non Linear Process Using Intelligent Techniques”, International Journal of Innovative Science Engineering & Technology, vol. 1, PP. 180-183, 2014.
[4] Aidan O'Dwyer, “Handbook of PI and PID Controller Tuning Rules”, Imperial College Press, 2006.
[5] M. Vijayakarthick and P. K. Bhaba, “Optimized Tuning of PI Controller for a Spherical Tank Level System Using New Modified Repetitive Control Strategy”, International Journal of Engineering Research and Development, vol. 3, pp. 74-82, 2012.
[6] D. Pradeepkannan, Dr. S. Sathiyamoorthy, “Control of a Nonlinear Spherical Tank Process Using GA Tuned PID Controller”, International Journal of Innovative Research in Science, Engineering and Technology, vol. 3, pp. 580-586, 2014.
[7] Bequette B. W, “Process control Modeling, Design and Simulation”, Prentice Hall of India, 2004.
[8] Omkar P. Dahale and Sharad P. Jadhav, “Design and Implementation of Controller using MPC Toolbox”, International Journal of Applied Information Systems, vol. 5, pp. 2249-0868, 2014.
[9] Tobias Raff, “Nonlinear Model Predictive Control of a Four Tank System- an Experimental Stability Study”, Proceedings of the IEEE International Conference, vol. 2, pp. 237-242, 2006.
[10] M.Bharathi and C.Selvakumar, “Model based controller design for a spherical tank”, International Journal of Computer Trends and Technology, vol. 3, pp.19-35, 2012.
[11] Neha Sharma and Kailash Singh, “Model predictive control and Neural Network predictive control of TAME reactive distillation column”, Journal of chemical engineering and processing, vol. 59, pp. 9-21, 2012.
[12] K. Hari Krishnaa, J. Satheesh Kumarb and Mahaboob Shaik, “Real Time Implementation of Model based Controller for a Spherical Tank”, International Journal of Current Engineering and Technology, vol. 3, pp. 587-590, 2013.
[13] Keerthi Chacko, Lakshmi Ponnusamy and Sangapillai Sutha “Model Predictive Controllers for Non minimum-Phase Quadruple-Tank Process”, Springer Publication, vol. 324, pp. 853-860, 2014.
[14] P. Liu, V. Kadirkamanathan and S. A. Billinks, “Predictive control for nonlinear systems using neural networks”, International Journal of Control, vol. 71, pp. 1119-1132, 1998.
[15] S.S.Ge, “Nonlinear adaptive control using neural networks and its application to CSTR systems”, Journal of Process Control, vol. 10,
pp. 313-323, 1998.
[16] M. Akesson, H. T.Toivonen, J. B. Waller and R. H. Nystrom, “Neural network approximation of a nonlinear model predictive controller applied to a pH neutralization process”, Journal of Computers and Chemical Engineering, vol. 29, pp. 323-335, 2005.
[17] Piyush shrivastava and A.Trivedi, “Control of nonlinear process using neural network based model predictive control”, International Journal
of Engineering Science and Technology, vol. 8, pp. 2573-2581, 2011
[18] T.Yuvapriya and P. Lakshmi, “Neuro based controller for a Spherical tank process”, International Conference on Advances in engineering, science and management, pp.106-110, 2012.
[19] Ramdane Hedjar,“Adaptive Neural Network Model Predictive Control”, International Journal of Innovative Computing, Information and Control, vol. 9, pp. 1245-1257, 2013.
[20] Ballekallu Chinna Eeranna, Subba Rao and G. Prabhaker Reddy, “Artificial Neural Network Based Modeling and Control of Bioreactor”, International Journal of Engineering Research & Technology, vol. 3, 2014.