Improving Classification of Retinal Fundus Image Using Flow Dynamics Optimized Deep Learning Methods
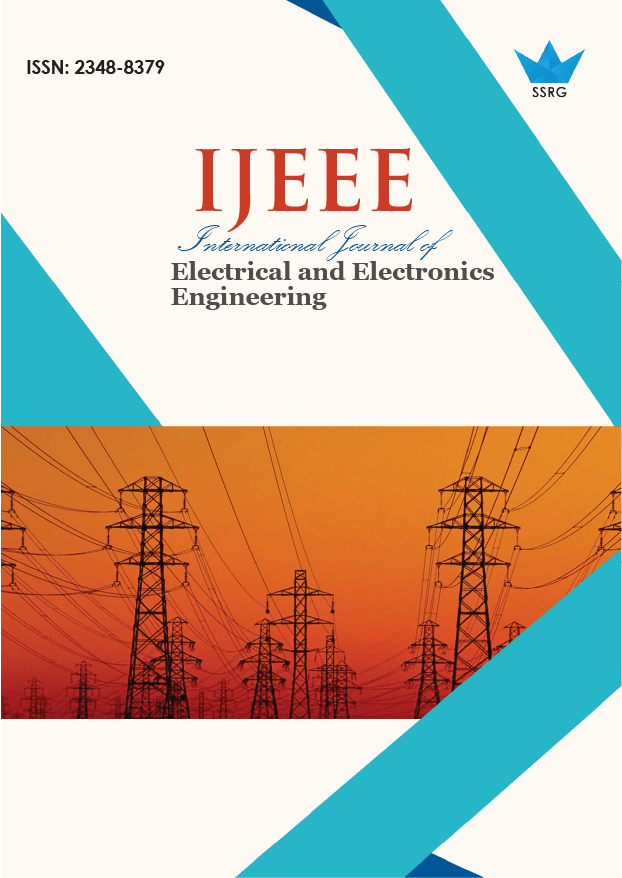
International Journal of Electrical and Electronics Engineering |
© 2022 by SSRG - IJEEE Journal |
Volume 9 Issue 12 |
Year of Publication : 2022 |
Authors : V. Banupriya, S. Anusuya |
How to Cite?
V. Banupriya, S. Anusuya, "Improving Classification of Retinal Fundus Image Using Flow Dynamics Optimized Deep Learning Methods," SSRG International Journal of Electrical and Electronics Engineering, vol. 9, no. 12, pp. 39-48, 2022. Crossref, https://doi.org/10.14445/23488379/IJEEE-V9I12P104
Abstract:
Diabetic Retinopathy (DR) refers to a barrier that takes place in diabetes mellitus damaging the blood vessel network present in the retina. This may endanger the subjects' vision if they have diabetes. It can take some time to perform a DR diagnosis using color fundus pictures because experienced clinicians are required to identify the tumors in the imagery used to identify the illness. Automated detection of the DR can be an extremely challenging task. Convolutional Neural Networks (CNN) are also highly effective at classifying images when applied in the present situation, particularly compared to the handmade and functionality methods employed. In order to guarantee high results, the researchers also suggested a cutting-edge CNN model that might determine the characteristics of the fundus images. The features of the CNN output were employed in various classifiers of machine learning for the proposed system. This model was later evaluated using different forms of deep learning methods and Visual Geometry Group (VGG) networks). It was done by employing the images from a generic KAGGLE dataset. Here, the River Formation Dynamics (RFD) algorithm proposed along with the FUNDNET to detect retinal fundus images has been employed. The investigation's findings demonstrated that the approach performed better than alternative approaches.
Keywords:
Diabetic Retinopathy (DR), Retinal Fundus Images, Deep Learning (DL), Visual Geometry Group (VGG) Network, Residual Networks (ResNet), and River Formation Dynamics (RFD).
References:
[1] Mohamed Shaban et al., “A Convolutional Neural Network for the Screening and Staging of Diabetic Retinopathy,” Plos One, vol. 15, p. E0233514, 2020. Crossref, https://doi.org/10.1371/journal.pone.0233514
[2] Amin Valizadeh et al., “Presentation of a Segmentation Method for a Diabetic Retinopathy Patient’s Fundus Region Detection Using a Convolutional Neural Network,” Computational Intelligence and Neuroscience, vol. 2021, pp. 1-14, 2021. Crossref, https://doi.org/10.1155/2021/7714351
[3] Davood Karimi, and Septimiu E, “Reducing the Hausdorff Distance in Medical Image Segmentation with Convolutional Neural Networks,” IEEE Transactions on Medical Imaging, vol. 39, no. 2, pp. 499-513, 2020. Crossref, https://doi.org/10.1109/TMI.2019.2930068
[4] Sehla Loussaief, and Afef Abdelkrim, “Convolutional Neural Network Hyper-Parameters Optimization Based on Genetic Algorithms,” International Journal of Advanced Computer Science and Applications, vol. 9, no. 10, pp. 252-266, 2018. Crossref, https://dx.doi.org/10.14569/IJACSA.2018.091031
[5] Jayanthi J et al., “An Intelligent Particle Swarm Optimization with Convolutional Neural Network for Diabetic Retinopathy Classification Model,” Journal of Medical Imaging and Health Informatics, vol. 11, no. 3, pp. 803-809, 2021. Crossref, https://doi.org/10.1166/jmihi.2021.3362
[6] Usharani Bhimavarapu, and Gopi Battineni, “Automatic Microaneurysms Detection for Early Diagnosis of Diabetic Retinopathy Using Improved Discrete Particle Swarm Optimization,” Journal of Personalized Medicine, vol. 12, no. 2, p. 317, 2022. Crossref, https://doi.org/10.3390/jpm12020317
[7] Roshini T. V et al., “Automatic Diagnosis of Diabetic Retinopathy with the Aid of Adaptive Average Filtering with Optimized Deep Convolutional Neural Network,” International Journal of Imaging Systems and Technology, vol. 30, no. 4, pp. 1173-1193, 2020. Crossref, https://doi.org/10.1002/ima.22419
[8] Paresh Chandra Sau, and Atul Bansal, “A Novel Diabetic Retinopathy Grading Using Modified Deep Neural Network with Segmentation of Blood Vessels and Retinal Abnormalities,” Multimedia Tools and Applications, vol. 81, pp. 39605–39633, 2022. Crossref, https://doi.org/10.1007/s11042-022-13056-y
[9] Manojkumar S B, and H S Sheshadri, “Analysis of Detection of Diabetic Retinopathy Using LPB and Deep Learning Techniques,” International Journal of Engineering Trends and Technology, vol. 68, no. 12, pp. 123-131, 2020. Crossref, https://doi.org/10.14445/22315381/IJETT-V68I12P221
[10] Ramya J, Rajakumar M. P, and Maheswari B. U, “Deep CNN with Hybrid Binary Local Search and Particle Swarm Optimizer for Exudates Classification from Fundus Images,” Journal of Digital Imaging, vol. 35, no. 1, pp. 56-67, 2022. Crossref, https://doi.org/10.1007/s10278-021-00534-2
[11] Anas Bilal et al., “Improved Grey Wolf Optimization-Based Feature Selection and Classification Using CNN for Diabetic Retinopathy Detection,” Evolutionary Computing and Mobile Sustainable Networks, vol. 116, pp. 1-4, 2022. Crossref, https://doi.org/10.1007/978-981-16-9605-3_1
[12] Raju Pugal Priya et al., “Deep Long and Short Term Memory Based Red Fox Optimization Algorithm for Diabetic Retinopathy Detection and Classification,” International Journal for Numerical Methods in Biomedical Engineering, vol. 38, no. 3, p. E3560, 2022. Crossref, https://doi.org/10.1002/cnm.3560
[13] Dr. Surendiran R et al., "Exploring the Cervical Cancer Prediction by Machine Learning and Deep Learning with Artificial Intelligence Approaches," International Journal of Engineering Trends and Technology, vol. 70, no. 7, pp. 94-107, 2022. Crossref, https://doi.org/10.14445/22315381/IJETT-V70I7P211
[14] Mark J. J. P. van Grinsven et al., “Fast Convolutional Neural Network Training Using Selective Data Sampling: Application to Hemorrhage Detection in Color Fundus Images,” IEEE Transactions on Medical Imaging, vol. 35, no. 5, pp. 1273-1284, 2016. Crossref, https://doi.org/10.1109/TMI.2016.2526689
[15] Darshit Doshi et al., “Diabetic Retinopathy Detection Using Deep Convolutional Neural Networks,” 2016 International Conference on Computing, Analytics and Security Trends (CAST), pp. 261-266, 2016. Crossref, https://doi.org/10.1109/CAST.2016.7914977
[16] Ms.S.Deepa, and Mr.S.Vijayprasath, "Certain Investigation of the Retinal Hemorrhage Detection in Fundus Images," SSRG International Journal of Electronics and Communication Engineering, vol. 2, no. 2, pp. 24-34, 2015. Crossref, https://doi.org/10.14445/23488549/IJECE-V2I2P106
[17] D. Dhinakaran et al., “Mining Privacy-Preserving Association Rules Based on Parallel Processing in Cloud Computing,” International Journal of Engineering Trends and Technology, vol. 70, no. 30, pp. 284-294, 2022. Crossref, https://doi.org/10.14445/22315381/IJETT-V70I3P232
[18] Kaushik R, and Kumar S, “Image Segmentation Using Convolutional Neural Network,” Internatıonal Journal of Scıentıfıc & Technology Research, vol. 8, no. 11, p. 667, 2019. Crossref, https://doi.org/10.1155/2021/7714351
[19] Chunyan Lian et al., “Deep Convolutional Neural Networks for Diabetic Retinopathy Classification,” Proceedings of the 2nd International Conference on Advances in Image Processing, pp. 68-72, 2018. Crossref, https://doi.org/10.1145/3239576.3239589
[20] Holly H. Vo, and Abhishek Verma, “New Deep Neural Nets for Fine-Grained Diabetic Retinopathy Recognition on Hybrid Color Space,” 2016 IEEE International Symposium on Multimedia (ISM), pp. 209-215, 2016. Crossref, https://doi.org/10.1109/ISM.2016.0049
[21] Tobias Hinz et al., “Speeding Up the Hyperparameter Optimization of Deep Convolutional Neural Networks,” International Journal of Computational Intelligence and Applications, vol. 17, no. 2, p. 1850008, 2018. Crossref, https://doi.org/10.1142/S1469026818500086
[22] D. Dhinakaran, et al., “Recommendation System for Research Studies Based on GCR,” 2022 International Mobile and Embedded Technology Conference (MECON), pp. 61-65, 2022. Crossref, https://doi.org/10.1109/MECON53876.2022.9751920
[23] Grzegorz Redlarski, Aleksander Pałkowski, and Mariusz Dąbkowski, “Using River Formation Dynamics Algorithm in Mobile Robot Navigation,” Solid State Phenomena, vol. 198, pp. 138-143, 2013. Crossref, https://doi.org/10.4028/www.scientific.net/SSP.198.138
[24] Grzegorz Redlarski, Mariusz Dabkowski, and Aleksander Palkowski, “Generating Optimal Paths in Dynamic Environments Using River Formation Dynamics Algorithm,” Journal of Computational Science, vol. 20, pp. 8-16, 2017. Crossref, https://doi.org/10.1016/j.jocs.2017.03.002
[25] S.M. Udhaya Sankar et al., "Safe Routing Approach by Identifying and Subsequently Eliminating the Attacks in Manet," International Journal of Engineering Trends and Technology, vol. 70, no. 11, pp. 219-231, 2022. Crossref, https://doi.org/10.14445/22315381/IJETT-V70I11P224
[26] Dhinakaran D et al., “Assistive System for the Blind with Voice Output Based on Optical Character Recognition,” International Conference on Innovative Computing and Communications, Lecture Notes in Networks and Systems, vol. 492, 2023. Crossref, https://doi.org/10.1007/978-981-19-3679-1_1
[27] Utkarsh Chouhan, and H N Verma, "Blood Vessel Segmentation for Iris in Unconstrained Environments Using Moment Method," SSRG International Journal of Computer Science and Engineering, vol. 5, no. 8, pp. 8-14, 2018. Crossref, https://doi.org/10.14445/23488387/IJCSE-V5I8P103
[28] Jena Catherine Bel D et al., "Trustworthy Cloud Storage Data Protection Based on Blockchain Technology," 2022 International Conference on Edge Computing and Applications (ICECAA), pp. 538-543, 2022. Crossref, https://doi.org/10.1109/ICECAA55415.2022.9936299
[29] Dr. Antarikhya Bordoloi, and Dr. Sayali Suhas Damle, "A Study of Severe Anemia in Elderly with Special Reference to Etiology," SSRG International Journal of Medical Science, vol. 7, no. 11, pp. 14-18, 2020. Crossref, https://doi.org/10.14445/23939117/IJMS-V7I11P103
[30] G. Gomathy et al., "Automatic Waste Management Based on IoT Using a Wireless Sensor Network," 2022 International Conference on Edge Computing and Applications (ICECAA), pp. 629-634, 2022. Crossref, https://doi.org/10.1109/ICECAA55415.2022.9936351
[31] A. Mary Dayana, and W. R. Sam Emmanuel, “An Enhanced Swarm Optimization-Based Deep Neural Network for Diabetic Retinopathy Classification in Fundus Images,” Multimedia Tools and Applications, vol. 81, pp. 20611-20642, 2022. Crossref, https://doi.org/10.1007/s11042-022-12492-0
[32] D. Dhinakaran, and P. M. Joe Prathap, “Preserving Data Confidentiality in Association Rule Mining Using Data Share Allocator Algorithm,” Intelligent Automation & Soft Computing, vol. 33, no. 3, pp. 1877–1892, 2022. Crossref, http://dx.doi.org/10.32604/iasc.2022.024509
[33] K. Sudharson et al., "Hybrid Deep Learning Neural System for Brain Tumor Detection," 2022 2nd International Conference on Intelligent Technologies (CONIT), pp. 1-6, 2022. Crossref, https://doi.org/10.1109/CONIT55038.2022.9847708
[34] D. Dhinakaran et al., "Secure Android Location Tracking Application with Privacy Enhanced Technique," 2022 Fifth International Conference on Computational Intelligence and Communication Technologies (CCICT), pp. 223-229, 2022. Crossref, https://doi.org/10.1109/CCiCT56684.2022.00050
[35] D. Dhinakaran, and P. M. Joe Prathap, "Protection of Data Privacy From Vulnerability Using Two-Fish Technique with Apriori Algorithm in Data Mining," The Journal of Supercomputing, vol. 78, no. 16, pp. 17559–17593, 2022. Crossref, https://doi.org/10.1007/S11227-022-04517-0