A Critical Literature Review on Computer Vision Based Melanoma Detection and Identification
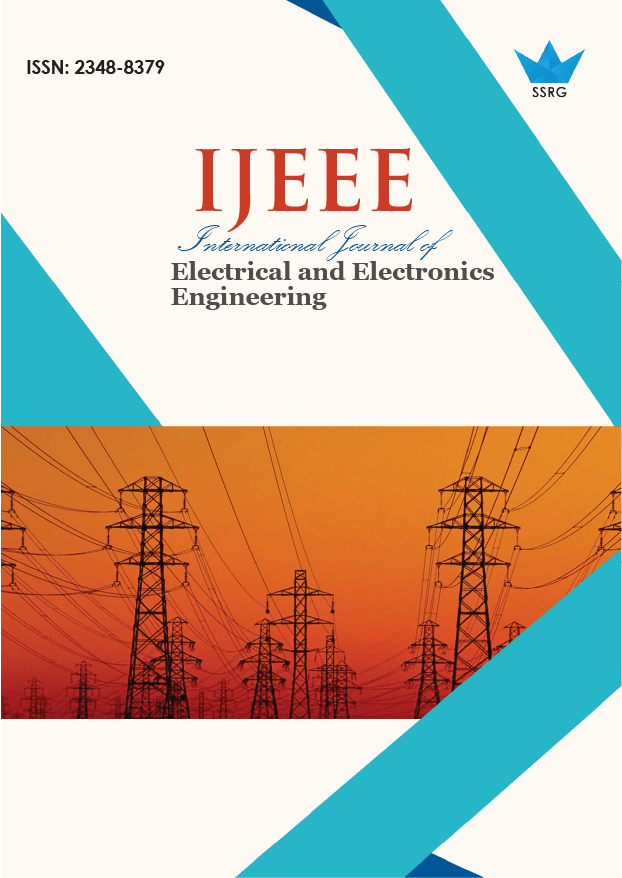
International Journal of Electrical and Electronics Engineering |
© 2022 by SSRG - IJEEE Journal |
Volume 9 Issue 12 |
Year of Publication : 2022 |
Authors : Soumya Gadag, P. Pradeepa |
How to Cite?
Soumya Gadag, P. Pradeepa, "A Critical Literature Review on Computer Vision Based Melanoma Detection and Identification," SSRG International Journal of Electrical and Electronics Engineering, vol. 9, no. 12, pp. 59-80, 2022. Crossref, https://doi.org/10.14445/23488379/IJEEE-V9I12P106
Abstract:
Melanoma is a skin tumor that initiates in the melanocyte cells that manage the pigmentation of the skin. Melanoma is still the most destructive form of skin cancer. In recent times, Convolutional neural network (CNN) centered classifiers have become quite popular for detecting melanoma. According to studies, CNN-based classifiers are equally accurate in classifying skin cancer scans as dermatologists. It has eased the process of Melanoma diagnoses. This work thoroughly assesses the most recent studies on the categorization of melanoma using deep learning and conventional machine learning procedures. The key purpose of this research is to compile cutting-edge research that identifies current research trends, problems, and prospects for melanoma diagnosis. It also looks into current approaches for applying deep learning to diagnose and identify melanoma. Finally, models, issues, and prospects have been provided to guide researchers working in the area of melanoma detection.
Keywords:
Melanoma, Skin cancer.
References:
[1] Niccolò Nami et al., “Teledermatology: State-of-the-Art and Future Perspectives,” Expert Review of Dermatology, vol. 7, no. 1, pp. 1-3, 2012. Crossref, https://doi.org/10.1586/edm.11.79
[2] "Cancer in Australia 2017," Cancer Series no. 101. Cat. no. CAN 100, Australian Institute of Health and Welfare, 2017.
[3] International Skin Imaging Collaboration, ISIC, 2019. [Online]. Available: https://challenge2019.Isic-Archive.com
[4] American Skin Cancer Organization, Skin Cancer Information, 2019. [Online]. Available: https://www.skincancer.org/skin-Cancer-Information/Skin-Cancer-Facts.
[5] FWHO, Ultraviolet Radiation, 2019. [Online]. Available: http://www.who.int/uv/faq/skincancer/en/index1.html
[6] Ashley Sample, and Yu-Ying He, "Mechanisms and Prevention of UV‐Induced Melanoma," Photodermatology, Photoimmunology & Photomedicine, vol. 34, no. 1, pp. 13-24, 2018. Crossref, https://doi.org/10.1111/phpp.12329
[7] Jean Cadet, and Thierry Douki, "Formation of UV-Induced DNA Damage Contributing to Skin Cancer Development," Photochemical & Photobiological Sciences, vol. 17, no. 12, pp. 1816-1841, 2018. Crossref, https://doi.org/10.1039/c7pp00395a
[8] Gerd P. Pfeifer et al., “Mechanisms of UV-Induced Mutations and Skin Cancer,” Genome Instability & Disease, vol. 1, no. 3, pp. 99- 113, 2020. Crossref, https://doi.org/10.1007%2Fs42764-020-00009-8
[9] Lei Bi et al., "Automatic Skin Lesion Analysis Using Large-Scale Dermoscopy Images and Deep Residual Networks," Computer Vision and Pattern Recognition, vol. 2, 2017. Crossref, https://doi.org/10.48550/arXiv.1703.04197
[10] Anthony F. Jerant et al., "Early Detection and Treatment of Skin Cancer," American Family Physician, vol. 62, no. 2, pp. 357-368, 2000.
[11] Dr. M. Emre Celebi et al., "Automatic Detection of Blue-White Veil and Related Structures in Dermoscopy Images," Computerized Medical Imaging and Graphics, vol. 32, no. 8, pp. 670–677, 2008. Crossref, https://doi.org/10.1016%2Fj.compmedimag.2008.08.003
[12] Qaisar Abbas et al., “Pattern Classification of Dermoscopy Images: A Perceptually Uniform Model,” Pattern Recognition, vol. 46, no. 1, pp. 86–97, 2013. Crossref, https://doi.org/10.1016/j.patcog.2012.07.027
[13] Adekanmi Adegun, and Serestina Viriri, "An Enhanced Deep Learning Framework for Skin Lesions Segmentation," International Conference on Computational Collective Intelligence, pp. 414–425, 2019. Crossref, http://dx.doi.org/10.1007/978-3-030-28377-3_34
[14] Muhammad Imran Qadir, "Skin Cancer: Etiology and Management," Pakistan Journal of Pharmaceutical Sciences, vol. 29, no. 3, pp. 999-1003, 2016.
[15] T. Tamilselvi et al., "Deep Derma Scan: A Proactive Diagnosis System for Predicting Malignant Skin Tumor with Deep Learning Mechanisms," International Journal of Engineering Trends and Technology, vol. 70, no. 8, pp. 310-317, 2022. Crossref, https://doi.org/10.14445/22315381/IJETT-V70I8P232
[16] T. Chase, K. E. Cham and B. E. Cham, "Curaderm, The Long-Awaited Breakthrough for Basal Cell Carcinoma," International Journal of Clinical Medicine, vol. 11, no. 10, pp. 579-604, 2020. Crossref, https://doi.org/10.4236/ijcm.2020.1110050
[17] Daniel E. Johnson et al., "Head and Neck Squamous Cell Carcinoma," Nature Reviews Disease Primers, vol. 6, pp. 1-22, 2020. Crossref, https://doi.org/10.1038/s41572-020-00224-3
[18] M. Raza Zaidi, David E. Fisher, and Helen Rizos, "Biology of Melanocytes and Primary Melanoma," Cutaneous Melanoma, pp. 3- 40, 2019. Crossref, https://doi.org/10.1007/978-3-319-46029-1_42-1
[19] Heinz Kutzner et al., “Overdiagnosis of Melanoma–Causes, Consequences and Solutions,” JDDG: Journal of the German Dermatological Society, vol. 18, no. 11, pp. 1236-1243, 2020. Crossref, https://doi.org/10.1111/ddg.14233
[20] Sharmin Majumder, and Muhammad Ahsan Ullah, "Feature Extraction from Dermoscopy Images for Melanoma Diagnosis," SN Applied Sciences, vol. 1, no. 7, pp. 1-11, 2019.
[21] Andre G.C.Pacheco, and Renato A. Krohling, "The Impact of Patient Clinical Information on Automated Skin Cancer Detection," Computers in Biology and Medicine, vol. 116, p. 103545, 2020. Crossref, https://doi.org/10.1016/j.compbiomed.2019.103545
[22] Eugenio Vocaturo, Ester Zumpano, and Pierangelo Veltri, "Image Pre-Processing in Computer Vision Systems for Melanoma Detection," In 2018 IEEE International Conference on Bioinformatics and Biomedicine (BIBM), pp. 2117-2124, 2018. Crossref, https://doi.org/10.1109/BIBM.2018.8621507
[23] Siegel R. L et al., "Cancer Statistics," CA: A Cancer Journal for Clinicians, vol. 72, no. 1, pp. 7-33, 2022. Crossref, https://doi.org/10.3322/caac.21708
[24] Akila Victor et al., "A Review on Skin Cancer Detection and Classification Using Infrared Images," International Journal of Engineering Trends and Technology, vol. 70, no. 4, pp. 403-417, 2022. Crossref, https://doi.org/10.14445/22315381/IJETT-V70I4P235
[25] Nayana Banjan, Prajkta Dalvi, and Neha Athavale, "Melanoma Skin Cancer Detection by Segmentation and Feature Extraction Using Combination of OTSU and STOLZ Algorithm Technique," SSRG International Journal of Electronics and Communication Engineering, vol. 4, no. 4, pp. 21-25, 2017. Crossref, https://doi.org/10.14445/23488549/IJECE-V4I4P105
[26] Mehwish Dildar et al., "Skin Cancer Detection: A Review Using Deep Learning Techniques," International Journal of Environmental Research and Public Health, vol. 18, no. 10, p. 5479, 2021. Crossref, https://doi.org/10.3390%2Fijerph18105479
[27] Nahata H, and Singh S. P, “Deep Learning Solutions for Skin Cancer Detection and Diagnosis," Machine Learning with Health Care Perspective, vol. 13, pp. 159-182, 2020. Crossref, https://doi.org/10.1007/978-3-030-40850-3_8
[28] Ali Bou Nassif et al., "Speech Recognition Using Deep Neural Networks: A Systematic Review," IEEE Access, vol. 7, pp. 19143- 19165, 2019. Crossref, https://doi.org/10.1109/ACCESS.2019.2896880
[29] Weibo Liu et al., "A Survey of Deep Neural Network Architectures and their Applications," Neurocomputing, vol. 234, pp. 11-26, 2017. Crossref, https://doi.org/10.1016/j.neucom.2016.12.038
[30] Seonwoo Min, Byunghan Lee, and Sungroh Yoon, "Deep Learning in Bioinformatics," Briefings in Bioinformatics, vol. 18, no. 5, pp. 851-869, 2017. Crossref, https://doi.org/10.1093/bib/bbw068
[31] Anusha Rao, and Kulkarni S. B, "A Hybrid Approach for Plant Leaf Disease Detection and Classification Using Digital Image Processing Methods," The International Journal of Electrical Engineering & Education, 2020. Crossref, https://doi.org/10.1177/0020720920953126
[32] Vipul Sharma, and Roohie Naaz Mir, "Saliency Guided Faster-RCNN (SGFr-RCNN) Model for Object Detection and Recognition," Journal of King Saud University-Computer and Information Science, vol. 34, no. 5, pp. 1687-1699. Crossref, https://doi.org/10.1016/j.jksuci.2019.09.012
[33] K. Siddesha, G. V. Jayaramaiah, and Chandrapal Singh, "A Novel Deep Reinforcement Learning Scheme for Task Scheduling in Cloud Computing," Cluster Computing, vol. 25, no. 6, pp. 1-18, 2022. Crossref, http://dx.doi.org/10.1007/s10586-022-03630-2
[34] Kaplan Kaplan et al., "Brain Tumor Classification Using Modified Local Binary Patterns (LBP) Feature Extraction Methods," Medical Hypotheses, vol. 139, p. 109696, 2020. Crossref, https://doi.org/10.1016/j.mehy.2020.109696
[35] Roberta B. Oliveira, Aledir S. Pereira, and João Manuel R. S. Tavares, "Computational Diagnosis of Skin Lesions from Dermoscopic Images Using Combined Features," Neural Computing and Applications, vol. 31, no. 10, pp. 6091-6111, 2019. Crossref, https://doi.org/10.1007/s00521-018-3439-8
[36] Farhat Afza et al., "Microscopic Skin Laceration Segmentation and Classification: A Framework of Statistical Normal Distribution and Optimal Feature Selection," Microscopy Research and Technique, vol. 82, no. 9, pp. 1471-1488, 2019. Crossref, http://dx.doi.org/10.1002/jemt.23301
[37] Muhammad Nasir et al., "An Improved Strategy for Skin Lesion Detection and Classification Using Uniform Segmentation and Feature Selection Based Approach," Microscopy Research and Technique, vol. 81, no. 6, pp. 528-543. Crossref, https://doi.org/10.1002/jemt.23009
[38] Akhator Terence Azeke, and Dele Eradebamwen Imasogie, "The Tale of Malignant Melanoma, a Tertiary Hospital Experience in South-South, Nigeria," SSRG International Journal of Medical Science, vol. 5, no. 6, pp. 9-14, 2018. Crossref, https://doi.org/10.14445/23939117/IJMS-V5I6P103
[39] Oukil S et al., "Automatic Segmentation and Melanoma Detection Based on Color and Texture Features in Dermoscopic Images," Skin Research and Technology, vol. 28, no. 2, pp. 203-2011, 2022. Crossref, https://doi.org/10.1111/srt.13111
[40] Mani Abedini et al., "Accurate and Scalable System for Automatic Detection of Malignant Melanoma," Dermoscopy Image Analysis, 2015. Crossref, http://dx.doi.org/10.1201/b19107-11
[41] Cudek P, and Hippe Z. S, "Melanocytic Skin Lesions: a New Approach to Color Assessment," 2015 8th International Conference on Human System Interaction (HIS), pp. 99-101, 2015. Crossref, https://doi.org/10.1109/HSI.2015.7170650
[42] Saptarshi Chatterjee, Debangshu Dey, and Sugata Munshi, “Optimal Selection of Features Using Wavelet Fractal Descriptors and Automatic Correlation Bias Reduction for Classifying Skin Lesions,” Biomedical Signal Processing and Control, vol. 40, pp. 252- 262, 2018. Crossref, https://doi.org/10.1016/j.bspc.2017.09.028
[43] Priyanti Paul Tumpa, and Md Ahasan Kabir, "An Artificial Neural Network Based Detection and Classification of Melanoma Skin Cancer Using Hybrid Texture Features," Sensors International, vol. 2, p. 100128, 2021. Crossref, https://doi.org/10.1016/j.sintl.2021.100128
[44] Doaa A. Shoieb, Sherin M. Youssef, and Walid M. Aly, "Computer-Aided Model for Skin Diagnosis Using Deep Learning," Journal of Image and Graphics, vol. 4, no. 2, pp. 122-129, 2016. Crossref, https://doi.org/10.18178/joig.4.2.122-129
[45] Luís Rosado et al., "From Dermoscopy to Mobile Teledermatology," Dermoscopy Image Analysis, 2015. Crossref, http://dx.doi.org/10.1201/B19107-13
[46] Ilona Kuzmina et al., "Towards Noncontact Skin Melanoma Selection by Multispectral Imaging Analysis," Journal of Biomedical Optics, vol. 16, no. 6, p. 060502, 2011. Crossref, http://dx.doi.org/10.1117/1.3584846
[47] Bernard Querleux et al., "Quantitative Magnetic Resonance Imaging of the Skin: in Vitro and in Vivo Applications," Imaging Technologies and Transdermal Delivery in Skin Disorders, pp. 341-369. Crossref, http://dx.doi.org/10.1002/9783527814633.Ch14
[48] Ali Rajabi-Estarabadi et al., "Optical Coherence Tomography Imaging of Melanoma Skin Cancer," Lasers in Medical Science, vol. 34, no. 2, pp. 411-420, 2019.
[49] Orlando Catalano et al., "Skin Cancer: Findings and Role of High-Resolution Ultrasound," Journal of Ultrasound, vol. 22, no. 4, pp. 423-431, 2019. Crossref, https://doi.org/10.1007/S40477-019-00379-0
[50] Mihaela Adriana Ilie et al., "Current and Future Applications of Confocal Laser Scanning Microscopy Imaging in Skin Oncology," Oncology Letters, vol. 17, no. 5, pp. 4102-4111, 2019. Crossref, https://doi.org/10.3892%2Fol.2019.10066
[51] Khalid M. Hosny, Mohamed A. Kassem, and Mohamed M. Fouad, "Classification of Skin Lesions into Seven Classes Using Transfer Learning with AlexNet," Journal of Digital Imaging, vol. 33, no. 5, pp. 1325-1334, 2020. Crossref, https://doi.org/10.1007/s10278- 020-00371-9
[52] Li Qiao et al., "Skin Cancer Diagnosis Based on a Hybrid Alexnet/Extreme Learning Machine Optimized by Fractional-Order Red Fox Optimization Algorithm," Proceedings of the Institution of Mechanical Engineers, Part H: Journal of Engineering in Medicine, 2022. Crossref, https://doi.org/10.1177/09544119221075941
[53] Ameri A, "A Deep Learning Approach to Skin Cancer Detection in Dermoscopy Images," Journal of Biomedical Physics & Engineering, vol. 10, no. 6, pp. 801-806, 2020. Crossref, https://doi.org/10.31661/jbpe.v0i0.2004-1107
[54] Ulzii-Orshikh Dorj et al., "The Skin Cancer Classification Using Deep Convolutional Neural Network," Multimedia Tools and Applications, vol. 77, no. 8, pp. 9909-9924, 2018. Crossref, https://doi.org/10.1007/s11042-018-5714-1
[55] Amirreza Mahbod et al., "Skin Lesion Classification Using Hybrid Deep Neural Networks," In ICASSP 2019-2019 IEEE International Conference on Acoustics, Speech and Signal Processing (ICASSP), IEEE, pp. 1229-1233, 2019. Crossref, https://doi.org/10.1109/ICASSP.2019.8683352
[56] Khalid M. Hosny, Mohamed A. Kassem, and Mohamed M. Foaud, "Classification of Skin Lesions Using Transfer Learning and Augmentation with Alex-Net," PloS One, vol. 14, no. 5, p. e0217293, 2019. Crossref, https://doi.org/10.1371/journal.pone.0217293
[57] Jason R Hagerty et al., "Deep Learning and Handcrafted Method Fusion: Higher Diagnostic Accuracy for Melanoma Dermoscopy Images," IEEE Journal of Biomedical and Health Informatics, vol. 23, no. 4, pp. 1385-1391, 2019. Crossref, https://doi.org/10.1109/JBHI.2019.2891049
[58] Said Yacine Boulahia, Mohamed Akram Benatia, and Abderrahmane Bouzar, "Att2ResNet: A Deep Attention‐Based Approach for Melanoma Skin Cancer Classification," International Journal of Imaging Systems and Technology, vol. 32, no. 2, pp. 476-489, 2022. Crossref, https://doi.org/10.1002/ima.22687
[59] Songtao Guo, and Zhouwang Yang, "Multi-Channel-ResNet: An Integration Framework towards Skin Lesion Analysis," Informatics in Medicine Unlocked, vol. 12, pp. 67-74, 2018. Crossref, https://doi.org/10.1016/j.imu.2018.06.006
[60] Muhammad Attique Khan et al., "Multi-Model Deep Neural Network Based Features Extraction and Optimal Selection Approach for Skin Lesion Classification," In 2019 International Conference on Computer and Information Sciences (ICCIS), IEEE, pp. 1-7, 2019. Crossref, https://doi.org/10.1109/ICCISci.2019.8716400
[61] E Yilmaz, and M. Trocan, "A Modified Version of Googlenet for Melanoma Diagnosis," Journal of Information and Telecommunication, vol. 5, no. 3, pp. 395-405, 2021. Crossref, https://doi.org/10.1080/24751839.2021.1893495
[62] Kalyanakumar Jayapriya, and Israel Jeena Jacob, "Hybrid Fully Convolutional Networks‐Based Skin Lesion Segmentation and Melanoma Detection Using Deep Feature," International Journal of Imaging Systems and Technology, vol. 30, no. 2, pp. 348-357, 2020. Crossref, https://doi.org/10.1002/ima.22377
[63] Tri-Cong Pham et al., "Deep CNN and Data Augmentation for Skin Lesion Classification," In Asian Conference on Intelligent Information and Database Systems, Springer, Cham, pp. 573-582, 2018. Crossref, https://doi.org/10.1007/978-3-319-75420-8_54
[64] Andre Esteva et al., "Dermatologist-Level Classification of Skin Cancer with Deep Neural Networks," Nature, vol. 542, no. 7639, pp. 115-118, 2017. Crossref, https://doi.org/10.1038/nature21056
[65] Lequan Yu et al., “Automated Melanoma Recognition in Dermoscopy Images via Very Deep Residual Networks,” IEEE Transactions on Medical Imaging, vol. 36, no. 4, pp. 994-1004, 2017. Crossref, https://doi.org/10.1109/TMI.2016.2642839
[66] Joanna Jaworek-Korjakowska, Pawel Kleczek, and Marek Gorgon, "Melanoma Thickness Prediction Based on Convolutional Neural Network with VGG-19 Model Transfer Learning," In Proceedings of the IEEE/CVF Conference on Computer Vision and Pattern Recognition Workshops, pp. 2748-2756, 2019. Crossref, https://doi.org/10.1109/CVPRW.2019.00333
[67] Ali Kaplan et al., "Prediction of Melanoma from Dermoscopic Images Using Deep Learning-Based Artificial Intelligence Techniques," In 2019 International Artificial Intelligence and Data Processing Symposium (IDAP), IEEE, pp. 1-5, 2019. Crossref, https://doi.org/10.1109/IDAP.2019.8875970
[68] Yiqi Yan, Jeremy Kawahara, and Ghassan Hamarneh, "Melanoma Recognition via Visual Attention," In International Conference on Information Processing in Medical Imaging, Springer, Cham, pp. 793-804, 2019. Crossref, https://doi.org/10.1007/978-3-030- 20351-1_62
[69] Tang Peng et al., "Efficient Skin Lesion Segmentation Using Separable-Unet with Stochastic Weight Averaging," Computer Methods and Programs in Biomedicine, vol. 178, pp. 289-301, 2019. Crossref, https://doi.org/10.1016/j.cmpb.2019.07.005
[70] Xiangwen Ding, and Shengsheng Wang, "Efficient Unet with Depth-Aware Gated Fusion for Automatic Skin Lesion Segmentation," Journal of Intelligent & Fuzzy Systems, vol. 40, no. 5, pp. 9963-9975, 2021. Crossref, https://doi.org/10.3233/JIFS-202566
[71] Sella Veluswami et al., "Melanoma Skin Cancer Recognition and Classification Using Deep Hybrid Learning," Journal of Medical Imaging and Health Informatics, vol. 11, no. 12, pp. 3110-3116, 2021. Crossref, https://doi.org/10.1166/jmihi.2021.3898
[72] Liang Gonog, and Yimin Zhou, "A Review: Generative Adversarial Networks," In 2019 14th IEEE Conference on Industrial Electronics and Applications (ICIEA), IEEE, pp. 505-510, 2019. Crossref, https://doi.org/10.1109/ICIEA.2019.8833686
[73] Ian Goodfellow, "NIPS 2016 Tutorial: Generative Adversarial Networks," arXiv preprint arXiv:1701.00160, 2016. Crossref, https://doi.org/10.48550/arXiv.1701.00160
[74] Haroon Rashid, M. Asjid Tanveer, and Hassan Aqeel Khan, "Skin Lesion Classification Using GAN Based Data Augmentation," In 2019 41st Annual International Conference of the IEEE Engineering in Medicine and Biology Society (EMBC), IEEE, pp. 916-919, 2019. Crossref, https://doi.org/10.1109/EMBC.2019.8857905
[75] Devansh Bisla et al., "Towards Automated Melanoma Detection with Deep Learning: Data Purification and Augmentation," In Proceedings of the IEEE/CVF Conference on Computer Vision and Pattern Recognition Workshops, pp. 2720-2728, 2019. Crossref, https://doi.org/10.1109/CVPRW.2019.00330
[76] Ibrahim Saad Ali, Mamdouh Farouk Mohamed, and Yousef Bassyouni Mahdy, "Data Augmentation for Skin Lesion using Self-Attention based Progressive Generative Adversarial Network," arXiv e-prints, arXiv-1910, 2019. Crossref, https://doi.org/10.48550/arXiv.1910.11960
[77] Arthur A. M. Teodoro et al., "A Skin Cancer Classification Approach using GAN and RoI-Based Attention Mechanism," Journal of Signal Processing Systems, 2022. Crossref, https://doi.org/10.1007/s11265-022-01757-4
[78] Adekanmi A. Adegun, and Serestina Viriri, "Deep Learning-Based System for Automatic Melanoma Detection," IEEE Access, vol. 8, pp. 7160-7172, 2019. Crossref, https://doi.org/110.1109/ACCESS.2019.2962812
[79] Mohammad Shorfuzzaman, "An Explainable Stacked Ensemble of Deep Learning Models for Improved Melanoma Skin Cancer Detection," Multimedia Systems, vol. 28, no. 4, pp. 1309-1323, 2022. Crossref, https://doi.org/10.1007/s00530-021-00787-5
[80] Samira Lafraxo, Mohamed El Ansari, and Said Charfi, “MelaNet: An Effective Deep Learning Framework for Melanoma Detection Using Dermoscopic Images,” Multimedia Tools and Applications, vol. 81, no. 11, pp. 16021-16045, 2022. Crossref, https://doi.org/10.1007/s11042-022-12521-y
[81] Usharani Bhimavarapu, and Gopi Battineni, "Skin Lesion Analysis for Melanoma Detection Using the Novel Deep Learning Model Fuzzy GC-SCNN," In Healthcare, vol. 10, no. 5, p. 962, 2022. Crossref, https://doi.org/10.3390/healthcare10050962
[82] Zhiying Xu et al., "Computer-Aided Diagnosis of Skin Cancer Based on Soft Computing Techniques," Open Medicine, vol. 15, no. 1, pp. 860-871, 2020. Crossref, https://doi.org/10.1515/med-2020-0131
[83] Ranpreet Kaur et al., “Melanoma Classification Using a Novel Deep Convolutional Neural Network with Dermoscopic Images,” Sensors, vol. 22, no. 3, p. 1134, 2022. Crossref, https://doi.org/10.3390/s22031134
[84] Philipp Tschandl, Cliff Rosendahl, and Harald Kittler, "The HAM10000 Dataset, a Large Collection of Multi-Source Dermatoscopic Images of Common Pigmented Skin Lesions," Scientific Data, vol. 5, no. 1, pp. 1-9, 2018. Crossref, https://doi.org/10.1038/sdata.2018.161
[85] Teresa Mendonça et al., "PH2 - A Dermoscopic Image Database for Research and Benchmarking," In 2013 35th Annual International Conference of the IEEE Engineering in Medicine and Biology Society (EMBC), IEEE, pp. 5437-5440, 2013. Crossref, https://doi.org/10.1109/EMBC.2013.6610779
[86] [Online]. Available: https://www.isicarchive.com/#!/topWithHeader/onlyHeaderTop/gallery?filter=%5B%5D
[87] Almut Boer, and KC Nischal, "A Growing Online Resource for Learning Dermatology and Dermatopathology," Indian Journal of Dermatology Venereology and Leprology, vol. 73, pp. 138-140, 2007. Crossref, https://doi.org/10.4103/0378-6323.31909
[88] DermIS. [Online]. Available: https://www.dermis.net/dermisroot/en/
[89] Giuseppe Argenziano et al., “Interactive atlas of Dermoscopy,” EDRA Medical Publishing and New Media, Milan, Italy, 2000.
[90] Dermnet. [Online]. Available: http://www.dermnet.com/dermatology-pictures-skin-disease-pictures/
[91] Nour Kibbi, Harriet Kluger, and Jennifer Nam Choi, "Melanoma: Clinical Presentations," Cancer Research and Treatment, pp.107- 129, 2016. Crossref, https://doi.org/10.1007/978-3-319-22539-5_4
[92] Bivas Biswas et al., "Not so 'rare'-An Example of Malignant Melanoma in India: Report from a Tertiary Cancer Centre," Ecancermedicalscience, vol. 15, p. 1335, 2021. Crossref, https://doi.org/10.3332/ecancer.2021.1335
[93] Catarina Campos et al., “High-Throughput Sequencing Identifies 3 Novel Susceptibility Genes for Hereditary Melanoma,” Genes, vol. 11, no. 4, p. 403, 2020. Crossref, https://doi.org/10.3390/genes11040403
[94] Imran Razzak et al., "Skin Lesion Analysis Toward Accurate Detection of Melanoma Using Multistage Fully Connected Residual Network," In 2020 International Joint Conference on Neural Networks (IJCNN), IEEE, pp. 1-8, 2020. Crossref, https://doi.org/10.1109/IJCNN48605.2020.9206881
[95] Vatsala Anand et al., "An Automated Deep Learning Models for Classification of Skin Disease Using Dermoscopy Images: A Comprehensive Study," Multimedia Tools and Applications, vol. 81, pp. 37379–37401, 2022. Crossref, https://doi.org/10.1007/s11042-021-11628-y
[96] Sameena Pathana K, Gopalakrishna Prabhu, and P.C.Siddalingaswamy, "Techniques and Algorithms for Computer Aided Diagnosis of Pigmented Skin Lesions—A Review," Biomedical Signal Processing and Control, vol. 39, pp. 237-262, 2018. Crossref, https://doi.org/10.1016/j.bspc.2017.07.010