Obstructive Sleep Apnea Severity Prediction Model GUI using Anthropometrics
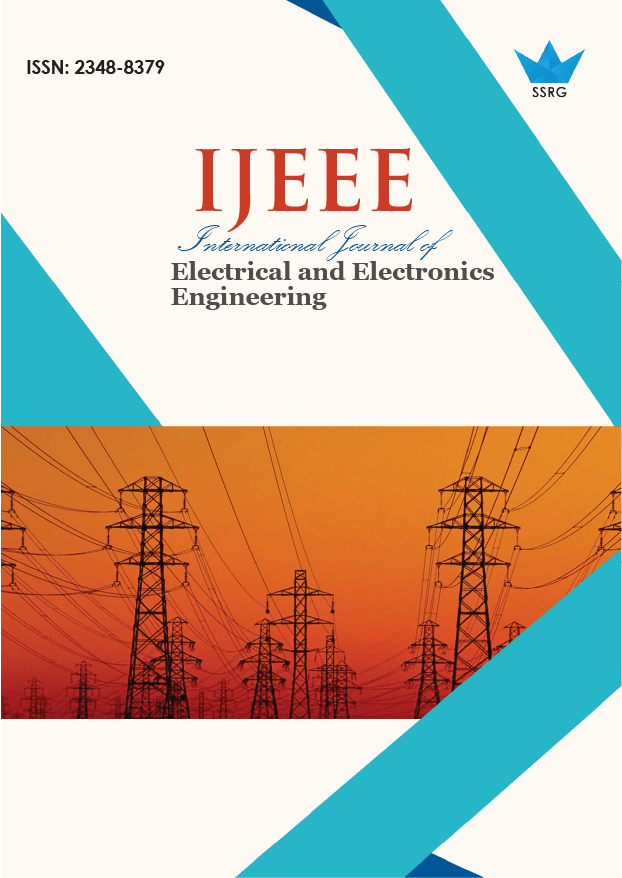
International Journal of Electrical and Electronics Engineering |
© 2022 by SSRG - IJEEE Journal |
Volume 9 Issue 12 |
Year of Publication : 2022 |
Authors : S. Aswath, S. Valarmathi |
How to Cite?
S. Aswath, S. Valarmathi, "Obstructive Sleep Apnea Severity Prediction Model GUI using Anthropometrics," SSRG International Journal of Electrical and Electronics Engineering, vol. 9, no. 12, pp. 134-144, 2022. Crossref, https://doi.org/10.14445/23488379/IJEEE-V9I12P112
Abstract:
It is still challenging to anticipate the austerity of obstructive sleep apnea (OSA) in clinical practice. Polysomnography (PSG) has been widely used for predicting OSA, but it is expensive. To tackle the challenge, in this work, a Machine Learning (ML) approach was used to create a predictive model for determining OSA severity level, and a web application was created based on the ML model, where anyone can check their level of OSA. Instead of PSG, anthropometrics such as weight, height, waist circumference, head, etc., can be used to predict OSA severity. Moreover, these parameters are very cheap and easy to measure. To validate this methodology, 5245 records from the TMUH (Taipei Medical University Hospital) sleep center dataset are employed, which has the physical body characteristics along with the age and gender of the patients. To evaluate the model, supervised machine learning classifiers were implemented to predict the OSA severity. Random Forest classifier performed well on processed data with an accuracy of 91%. With the help of a random forest model pickle file, a web application has been developed to classify the OSA severity based on anthropometrics.
Keywords:
Anthropometrics, Obstructive sleep apnea, Polysomnography, Supervised machine learning classifier, Web application.
References:
[1] Ayub S, and Won CH, “Obstructive Sleep Apnea in Women,” Journal of Sleep Medicine, vol. 16, no. 2, pp. 75-80, 2019. Crossref, http://doi.org/10.13078/jsm.190047
[2] Alison Wimms et al., “Obstructive Sleep Apnea in Women: Specific Issues and Interventions,” BioMed Research International, vol. 2016, p. 1764837, 2016. Crossref, http://doi.org/10.1155/2016/1764837
[3] Rundo JV, “Obstructive Sleep Apnea Basics,” Cleveland Clinic Journal of Medicine, vol. 86, no. 9-1, pp. 2-9, 2019. Crossref, http://doi.org/10.3949/ccjm.86.s1.02
[4] Patil SP et al., “Treatment of Adult Obstructive Sleep Apnea with Positive Airway Pressure: an American Academy of Sleep Medicine Clinical Practice Guideline,” Journal of Clinical Sleep Medicine, vol. 15, no. 2, pp. 335-43, 2019. Crossref, https://doi.org/10.5664/jcsm.7640
[5] Canessa. N et al., “Obstructive Sleep Apnea: Brain Structural Changes and Neurocognitive Function before and after Treatment,” American Journal of Respiratory and Critical Care Medicine, vol. 183, no. 10, pp. 1419-1426, 2011. Crossref, https://doi.org/10.1164/rccm.201005-0693oc
[6] Salman LA, Shulman R, and Cohen JB, “Obstructive Sleep Apnea, Hypertension, and Cardiovascular Risk: Epidemiology, Pathophysiology, and Management,” Current Cardiology Reports, vol. 22, no. 2, pp. 1-9, 2020. Crossref, https://doi.org/10.1007/s11886-020-1257-y
[7] Patrick. J et al., “Obstructive Sleep Apnea,” The New England Journal of Medicine, vol. 334, pp. 99-104, 1996. Crossref, https://doi.org/10.1056/NEJM199601113340207
[8] Mayo Clinic, Website. [Online]. Available: https://www.mayoclinic.org/diseases-conditions/obstructive-sleep-apnea/symptoms-causes/syc-20352090 [9] Bonsignore MR et al., “Obstructive Sleep Apnea and Comorbidities: a Dangerous Liaison,” Multidisciplinary Respiratory Medicine, vol. 14, no. 8, pp. 1-2, 2019. Crossref, https://doi.org/10.1186/s40248-019-0172-9
[10] Mohan Kumar, Sunil Kumar Khatri, and Masoud Mohammadian, “Feature Based Ensemble Learning Model for Breast Cancer Reoccurrence Retrieval,” International Journal of Engineering Trends and Technology, vol. 70, no. 10, pp. 210-220, 2022. Crossref, https://doi.org/10.14445/22315381/IJETT-V70I10P220
[11] Gottlieb DJ, Punjabi NM, “Diagnosis and Management of Obstructive Sleep Apnea: a Review,” JAMA network, vol. 323, no. 14, pp. 1389-1400, 2020. Crossref, https://doi.org/10.1001/jama.2020.3514
[12] Faal. M, and Almasganj. F, “Obstructive Sleep Apnea Screening from Unprocessed ECG Signals using Statistical Modeling,” Biomedical Signal Processing and Control, vol. 68, p. 102685, 2021. Crossref, https://doi.org/10.1016/j.bspc.2021.102685
[13] Ruobing Wei et al., “Body Fat Indices as Effective Predictors of Insulin Resistance in Obstructive Sleep Apnea: Evidence from a Cross-Sectional and Longitudinal Study,” Obesity Surgery, vol. 31, no. 5, pp. 2219-30, 2021. Crossref, https://doi.org/10.1007/s11695-021-05261-9
[14] Kamble P. G et al., “Sleep Apnea in Men is Associated with Altered Lipid Metabolism, Glucose Tolerance, Insulin Sensitivity, and Body Fat Percentage,” Endocrine, vol. 70, no. 1, pp. 48-57, 2020. Crossref, https://doi.org/10.1007/s12020-020-02369-3
[15] Piqueras. P et al., “Anthropometric Indicators as a Tool for Diagnosis of Obesity and Other Health Risk Factors: a Literature Review,” Frontiers in Psychology, vol. 12, 2021. Crossref, https://doi.org/10.3389%2Ffpsyg.2021.631179
[16] Casadei, Kyle, and John Kiel, “Anthropometric Measurement,” StatPearls Publishing, 2022.
[17] Wysocki. J et al., “Anthropometric and Physiologic Assessment in Sleep Apnoea Patients Regarding Body Fat Distribution,” Folia Morphologica, vol. 75, no. 3, pp. 393-399, 2016. Crossref, https://doi.org/10.5603/fm.a2015.0127
[18] Sherri Lynne Katz et al., “Does Neck-to-Waist Ratio Predict Obstructive Sleep Apnea in Children?,” Journal of Clinical Sleep Medicine, vol. 10, no. 12, pp. 1303-1308, 2014. Crossref, https://doi.org/10.5664%2Fjcsm.4284
[19] Wen-Te Liu et al., “Prediction of the Severity of Obstructive Sleep Apnea by Anthropometric Features via Support Vector Machine,” PloS ONE, vol. 12, no. 5, p. e0176991, 2017. Crossref, https://doi.org/10.1371/journal.pone.0176991
[20] Young Jae Kim et al., “Prediction Models for Obstructive Sleep Apnea in Korean Adults using Machine Learning Techniques,” Diagnostics, vol. 11, no. 4, p. 612, 2021. Crossref, https://doi.org/10.3390/diagnostics11040612
[21] Keshavarz, Zahra et al., “Obstructive Sleep Apnea: a Prediction Model using Supervised Machine Learning Method,” Studies in Health Technology and Informatics, vol. 272, pp. 387-390, 2020. Crossref, https://doi.org/10.3233/SHTI200576
[22] Corrado Mencar et al., “Application of Machine Learning to Predict Obstructive Sleep Apnea Syndrome Severity,” Health Informatics Journal, vol. 26, no. 1, pp. 298-317, 2020. Crossref, https://doi.org/10.1177/1460458218824725
[23] Steven J. Holfinger et al., “Diagnostic Performance of Machine Learning-Derived OSA Prediction Tools in Large Clinical and Community-Based Samples,” Chest, vol. 161, no. 3, pp. 807-17, 2022. Crossref, https://doi.org/10.1016/j.chest.2021.10.023
[24] Alaa Sheta et al., “Diagnosis of Obstructive Sleep Apnea from ECG Signals Using Machine Learning and Deep Learning Classifiers,” Applied Sciences, vol. 11, no. 14, p. 6622, 2021. Crossref, https://doi.org/10.3390/app11146622
[25] Gutiérrez‐Tobal GC et al., “Reliability of Machine Learning to Diagnose Pediatric Obstructive Sleep Apnea: Systematic Review and Meta‐Analysis,” Pediatric Pulmonology, vol. 57, no. 8, pp. 1931-1943, 2022. Crossref, https://doi.org/10.1002/ppul.25423
[26] Srimathi. S, Yamuna. G, and Nanmaran. R, “Neural Networks Based Cancer Classification Model Using CT-PET Fused Images,” Advances in Computing and Data Sciences, Communications in Computer and Information Science, vol. 1045, 2019. Crossref, https://doi.org/10.1007/978-981-13-9939-8_10
[27] Srimathi. S, Yamuna. G, and Nanmaran. R, “Threshold Based Stochastic Regression Model with Gabor Filter for Segmentation and Random Forest Classification of Lung Cancer,” Journal of Computational and Theoretical Nanoscience, vol. 16, no. 4, pp. 1666- 1673, 2019. Crossref, https://doi.org/10.1166/jctn.2019.8095
[28] Shanmugham Balasundaram et al., “Automated Detection and Classification of Breast Cancer Nuclei with Deep Convolutional Neural Network,” Journal of ICT Research & Applications, vol. 15, no. 2, 2021. Crossref, https://doi.org/10.5614/itbj.ict.res.appl.2021.15.2.3
[29] Wen-Te Liu et al., “Prediction of the Severity of Obstructive Sleep Apnea by Anthropometric Features via Support Vector Machine,” PloS One, vol. 12, no. 5, p. e0176991, 2017. Crossref, https://doi.org/10.1371/journal.pone.0176991
[30] Luiz Antônio Alves de Menezes Júnior et al., “Diagnostic Accuracy of the Berlin Questionnaire and the Nosas Score in Detecting Risk for Obstructive Sleep Apnea in Rotating Shift Workers,” Sleep and Breathing, vol. 26, no. 2, pp. 743-751, 2022. Crossref, https://doi.org/10.1007/s11325-021-02446-5
[31] Liang XW et al., “LR-SMOTE—an Improved Unbalanced Data set Oversampling Based on K-Means and SVM,” Knowledge-Based Systems, vol. 196, p. 105845, 2020. Crossref, https://doi.org/10.1016/j.knosys.2020.105845
[32] Nidhi Mongoriya, and Vinod Patel, “Review the Breast Cancer Detection Technique using Hybrid Machine Learning,” SSRG International Journal of Computer Science and Engineering, vol. 8, no. 6, pp. 5-8, 2021. Crossref, https://doi.org/10.14445/23488387/IJCSE-V8I6P102
[33] Fernández A et al., “SMOTE for Learning from Imbalanced Data: Progress and Challenges, Marking the 15-Year Anniversary,” Journal of Artificial Intelligence Research, vol. 61, pp. 863-905, 2018. Crossref, https://doi.org/10.1613/jair.1.11192
[34] Ishaq A et al., “Improving the Prediction of Heart Failure Patients’ Survival Using Smote and Effective Data Mining Techniques,” IEEE Access, vol. 9, pp. 39707-39716, 2021. Crossref, https://doi.org/10.1109/ACCESS.2021.3064084
[35] Thapa C et al., “Splitfed: When Federated Learning Meets Split Learning,” Proceedings of the AAAI Conference on Artificial Intelligence, vol. 36, no. 8, pp. 8485-8493, 2022. Crossref, https://doi.org/10.1609/aaai.v36i8.20825
[36] S. Aswath et al., “Enhancing the Performance of Classifiers in Detecting Abnormalities in Medical Data using Nature Inspired Optimization Techniques,” 2022 International Conference for Advancement in Technology, pp. 1-7, 2022. Crossref, https://doi.org/10.1109/ICONAT53423.2022.9726096
[37] Abdulrazaq. MB et al., “An Analytical Appraisal for Supervised Classifiers’ Performance on Facial Expression Recognition Based on Relief-F Feature Selection,” Journal of Physics: Conference Series, vol. 1804, no. 1, p. 012055, 2021. Crossref, https://doi.org/10.1088/1742-6596/1804/1/012055
[38] Shakti Chourasiya, and Suvrat Jain, “A Study Review on Supervised Machine Learning Algorithms,” SSRG International Journal of Computer Science and Engineering, vol. 6, no. 8, pp. 16-20, 2019. Crossref, https://doi.org/10.14445/23488387/IJCSE-V6I8P104
[39] N. Seliya, T. M. Khoshgoftaar, and J. Van Hulse, “A Study on the Relationships of Classifier Performance Metrics,” 2009 21st IEEE International Conference on Tools with Artificial Intelligence, pp. 59-66, 2009. Crossref, https://doi.org/10.1109/ICTAI.2009.25
[40] Krstinić D et al., “Multi-Label Classifier Performance Evaluation with Confusion,” Conference: International Conference on Soft Computing, Artificial Intelligence and Machine Learning, vol. 10, no. 8, pp. 1-4, 2020. Crossref, https://doi.org/10.5121/csit.2020.100801
[41] Vyshnavi. VR et al., “Efficient Way of Web Development using Python and Flask,” International Journal of Recent Research Aspects, vol. 6, no. 2, pp. 16-9, 2019.
[42] Akanksha Pandey, and L S Maurya, “Career Prediction Classifiers based on Academic Performance and Skills using Machine Learning,” SSRG International Journal of Computer Science and Engineering, vol. 9, no. 3, pp. 5-20, 2022. Crossref, https://doi.org/10.14445/23488387/IJCSE-V9I3P102
[43] Kanagathara N, and Nanmaran R, “Illustration of Potential Energy Surface from DFT Calculation Along with Fuzzy Logic Modelling for Optimization of N-Acetylglycine,” Computational and Theoretical Chemistry, vol. 1202, p. 113301, 2021. Crossref, https://doi.org/10.1016/j.comptc.2021.113301
[44] Srimathi. S, Yamuna. G, and Nanmaran. R, “An Efficient Cancer Classification Model for CT/MRI/PET Fused Images,” Current Medical Imaging, vol. 17, no. 3, pp. 319-330, 2021. Crossref, https://doi.org/10.2174/1573405616666200628134800
[45] Roebuck. A et al., “A Review of Signals Used in Sleep Analysis,” Physiological Measurement, vol. 35, 2014. Crossref, https://doi.org/10.1088/0967-3334/35/1/R1
[46] Nanmaran. R et al., “Investigating the Role of Image Fusion in Brain Tumor Classification Models Based on Machine Learning Algorithm for Personalized Medicine,” Computational and Mathematical Methods in Medicine, vol. 2022, 2022. Crossref, https://doi.org/10.1155/2022/7137524
[47] Rácz A, Bajusz D, and Héberger K, “Multi-Level Comparison of Machine Learning Classifiers and Their Performance Metrics,” Molecules, vol. 24, no. 15, p. 2811, 2019. Crossref, https://doi.org/10.3390/molecules24152811