The Detection and Identification of Pest-FAW Infestation in Maize Crops Using Iot-Based Deep-Learning Algorithm
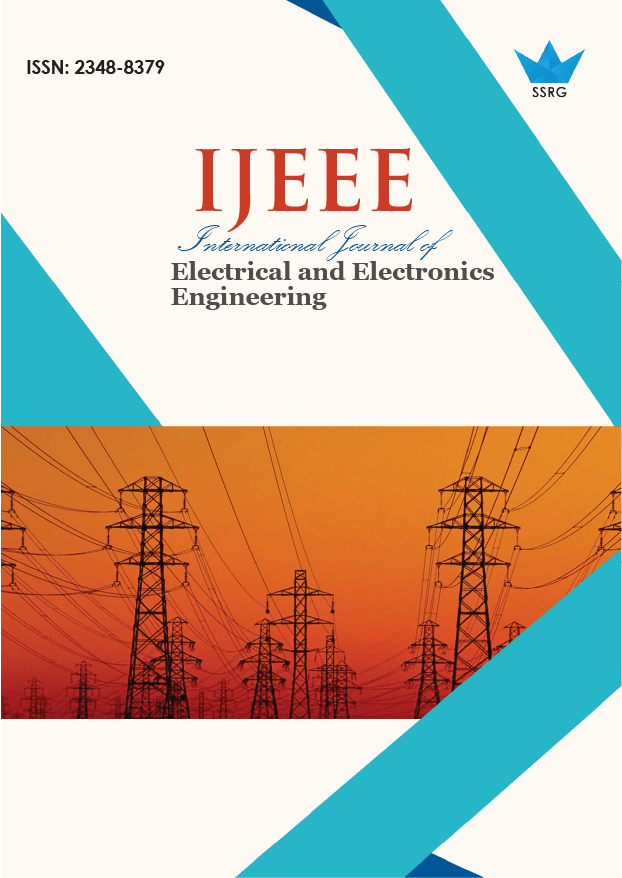
International Journal of Electrical and Electronics Engineering |
© 2022 by SSRG - IJEEE Journal |
Volume 9 Issue 12 |
Year of Publication : 2022 |
Authors : D. Sheema, K. Ramesh, V. K. Reshma, R. Surendiran |
How to Cite?
D. Sheema, K. Ramesh, V. K. Reshma, R. Surendiran, "The Detection and Identification of Pest-FAW Infestation in Maize Crops Using Iot-Based Deep-Learning Algorithm," SSRG International Journal of Electrical and Electronics Engineering, vol. 9, no. 12, pp. 180-188, 2022. Crossref, https://doi.org/10.14445/23488379/IJEEE-V9I12P116
Abstract:
In today's world, technology plays an integral role in addressing everyday challenges. Using technology in agriculture allows farmers to increase productivity while managing natural resources. Agriculture has always been plagued by pests, which destroy parts of the crops or even the whole field. Pest control at an early stage of crop development is a challenge for farmers. It eventually has a negative impact on the economy. By examining the odour substance of pests, a novel way to analyze pests is presented in this study. There is a distinct smell associated with every pest. Since odour can identify concealed pests, including deeply buried pests, swirled pests, etc., it is easier to detect than other detection methodologies. In light of this, based on odour as a key consideration, the proposed system evaluates five different odours, such as pungent, musty, misty, and sweet. In this case, gas sensors are analyzed, and then features are extracted using a Faster R-CNN algorithm. Additionally, it can be used to determine the density of infestations and counting. It is possible to develop process accuracy and timeliness using pseudocode. Comparatively, accuracy increased to 6% from Faster R CNN-based pest detection. Samples of the proposed development have been tested for performance metrics.
Keywords:
Pest detection, Odour detection, Deep learning detection, IoT.
References:
[1] Liu, Jun, and Xuewei Wang, "Plant Diseases and Pests Detection Based on Deep Learning: A Review,” Plant Methods, vol. 17, no. 1, pp. 1-18, 2021. Crossref, https://doi.org/10.1186/S13007-021-00722-9
[2] Fouqiya Badar, and Ayesha Naaz, "Detection of Fruit Diseases using Image Processing Techniques: A Review," SSRG International Journal of Electronics and Communication Engineering, vol. 9, no. 4, pp. 10-14, 2022. Crossref, https://doi.org/10.14445/23488549/IJECE-V9I4P102
[3] Mohamed Esmail Karar et al., “A New Mobile Application of Agricultural Pests Recognition Using Deep Learning in Cloud Computing System,” Alexandria Engineering Journal, vol. 60, no. 5, pp. 4423-4432, 2021. Crossref, https://doi.org/10.1016/j.aej.2021.03.009
[4] Deepali M Bongulwar, Vijay Prakash Singh, and S. N. Talbar, "Evaluation of CNN based on Hyperparameters to Detect the Quality of Apples," International Journal of Engineering Trends and Technology, vol. 70, no. 10, pp. 232-246, 2022. Crossref, https://doi.org/10.14445/22315381/IJETT-V70I10P222
[5] Naeem Ullah et al., “An Efficient Approach for Crops Pests Recognition and Classification Based on Novel DeeppestNet Deep Learning Model,” IEEE Access, vol. 10, pp. 73019-73032, 2022. Crossref, https://doi.org/10.1109/ACCESS.2022.3189676
[6] O. Nagaraja, and M. Krishnappa, "Leaf blight of Castor caused by Alternaria ricini: Detection and Pathogenicity in Castor (Ricinus Communis L) Seed," SSRG International Journal of Agriculture & Environmental Science, vol. 3, no. 4, pp. 6-10, 2016. Crossref, https://doi.org/10.14445/23942568/IJAES-V3I4P102
[7] Dan Jeric Arcega Rustia et al., “Automatic Greenhouse Insect Pest Detection and Recognition Based on a Cascaded Deep Learning Classification Method,” Journal of Applied Entomology, vol. 145, no. 3, pp. 206-222, 2021. Crossref, https://doi.org/10.1111/jen.12834
[8] D. Sheema et al., "An Algorithm for Detection and Identification of Infestation Density of Pest-Fall Armyworm in Maize Plants using Deep Learning based on IoT," International Journal of Engineering Trends and Technology, vol. 70, no. 9, pp. 240-251, 2022. Crossref, https://doi.org/10.14445/22315381/IJETT-V70I9P224
[9] Li, Wei, et al., “Recommending Advanced Deep Learning Models for Efficient Insect Pest Detection,” Agriculture, vol. 12, no. 7, pp. 1065, 2022. Crossref, https://doi.org/10.3390/agriculture12071065
[10] T.Nagarathinam, and Dr. K. Rameshkumar, "A Survey on Cluster Analysis Techniques for Plant Disease Diagnosis," SSRG International Journal of Computer Science and Engineering, vol. 3, no. 6, pp. 11-17, 2016. Crossref, https://doi.org/10.14445/23488387/IJCSE-V3I6P103
[11] Juan Guo, “ODRP: A Deep Learning Framework for Odour Descriptor Rating Prediction Using Electronic Nose,” IEEE Sensors Journal, vol. 21, no. 13, pp. 15012-15021, 2021. Crossref, https://doi.org/10.1109/JSEN.2021.3074173
[12] S. Bhuvaneswari et al., "Disease Detection in Plant Leaf using LNet Based on Deep Learning," International Journal of Engineering Trends and Technology, vol. 70, no. 9, pp. 64-75, 2022. Crossref, https://doi.org/10.14445/22315381/IJETT-V70I9P207
[13] Wang, Zhenyu, Duokun Yin, and Senrong Ji, “Real-Time Factory Smoke Detection Based on Two-Stage Relation-Guided Algorithm,” Scientific Reports, vol. 12, no. 1, pp. 1-15, 2022. Crossref, https://doi.org/10.1038/s41598-022-05523-1
[14] Nitin Kumar Vishwakarma, Dr.Ragini Shukla, and Dr. Ravi Mishra, "A Review of Different Methods for Implementing Smart Agriculture on an IoT Platform," SSRG International Journal of Computer Science and Engineering, vol. 7, no. 12, pp. 5-8, 2020. Crossref, https://doi.org/10.14445/23488387/IJCSE-V7I12P102
[15] Susovan Jana, Ranjan Parekh, and Bijan Sarkar, “Detection of Rotten Fruits and Vegetables Using Deep Learning,” Computer Vision and Machine Learning in Agriculture, pp. 31-49, 2022.
[16] P. Sindhu, and G. Indirani, "Equilibrium Optimizer with Deep Convolutional Neural Network-based SqueezeNet Model for Grape Leaf Disease Classification in IoT Environment," International Journal of Engineering Trends and Technology, vol. 70, no. 5, pp. 94-102, 2022. Crossref, https://doi.org/10.14445/22315381/IJETT-V70I5P212
[17] Yizhou Xiong et al., “An Odour Recognition Algorithm of Electronic Noses Based on Convolutional Spiking Neural Network for Spoiled Food Identification,” Journal of the Electrochemical Society, vol. 168, no. 7, p. 077519, 2021. Crossref, https://doi.org/10.1149/1945-7111/ac1699
[18] Ashay Rokade, and Manwinder Singh, “Analysis of Precise Green House Management System Using Machine Learning Based Internet of Things (IoT) for Smart Farming,” 2021 2nd International Conference on Smart Electronics and Communication (ICOSEC), IEEE, 2021. Crossref, https://doi.org/10.1109/ICOSEC51865.2021.9591962
[19] Jun LI, and Shang XIANG, "Intelligent Control of Urban Fresh Agricultural Products Supply Chain using Big Data and Internet of Things," SSRG International Journal of Computer Science and Engineering, vol. 7, no. 9, pp. 1-6, 2020. Crossref, https://doi.org/10.14445/23488387/IJCSE-V7I9P101
[20] Sharma, Anjuet al., “Smiles to Smell: Decoding the Structure–Odor Relationship of Chemical Compounds Using the Deep Neural Network Approach,” Journal of Chemical Information and Modeling, vol. 61, no. 2, pp. 676-688, 2021. Crossref, https://doi.org/10.1021/acs.jcim.0c01288
[21] Nitin Kumar Vishwakarma, Dr.Ragini Shukla, and Dr. Ravi Mishra, "A Review of Different Methods for Implementing Smart Agriculture on an IoT Platform," SSRG International Journal of Computer Science and Engineering, vol. 7, no. 12, pp. 5-8, 2020. Crossref, https://doi.org/10.14445/23488387/IJCSE-V7I12P102
[22] Sheema, D et al., “Fall Armyworm Detection on Maize Plants Using Gas Sensors, Image Classification, and Neural Network Based on IoT,” International Journal of Intelligent Systems and Applications in Engineering, vol. 10, no. 2s, pp. 165-173, 2022.
[23] M. H. Nayeem et al., "Application of Image Processing for Plant Disease Identification Based on SVM Technique," International Journal of Engineering Trends and Technology, vol. 68, no. 1, pp. 83-90, 2020. Crossref, https://doi.org/10.14445/22315381/IJETT-V68I1P213
[24] Jhatial, Muhammad Juman et al., “Deep Learning-Based Rice Leaf Diseases Detection Using Yolov5,” Sukkur IBA Journal of Computing and Mathematical Sciences, vol. 6, no.1, pp. 49-61, 2022. Crossref, http://dx.doi.org/10.30537/sjcms.v6i1.1009
[25] Rajendra Akhil Kuma et al., "Crop Monitoring using Visual Sensors and IoT," SSRG International Journal of Electronics and Communication Engineering, vol. 4, no. 4, pp. 42-47, 2017. Crossref, https://doi.org/10.14445/23488549/IJECE-V4I4P109
[26] Johannes Full et al., “Systematic Derivation of New Fields of Application for Innovative Bio-Based Odour Sensors with Transfected Cells and Analysis of Economic Potentials,” In Proceedings of the 1st International Electronic Conference on Biosensors, MDPI, 2020. Crossref, https://doi.org/10.3390/IECB2020-07029
[27] Geetha D et al., "Human and Animal Movement Detection in Agricultural Fields," SSRG International Journal of Computer Science and Engineering, vol. 6, no. 1, pp. 15-18, 2019. Crossref, https://doi.org/10.14445/23488387/IJCSE-V6I1P103
[28] Rinu Chacko et al., “Data Based Predictive Models for Odour Perception,” Scientific Reports, vol. 10, no. 1, pp. 1-13, 2020. Crossref, https://doi.org/10.1038/s41598-020-73978-1
[29] Rina Mahakud, Binod Kumar Pattanayak, and Bibudhendu Pati, "A Hybrid Multi-class Classification Model for the Detection of Leaf Disease using XGBoost and SVM," International Journal of Engineering Trends and Technology, vol. 70, no. 10, pp. 298-306, 2022. Crossref, https://doi.org/10.14445/22315381/IJETT-V70I10P229
[30] Rana Muhammad Saleem et al., “Iot-Based Cotton Whitefly Prediction Using Deep Learning,” Scientific Programming, vol. 2021, 2021. Crossref, https://doi.org/10.1155/2021/8824601
[31] Aniket Ravindra Jambukar, and Prof. N A Dawande, "IoT based Smart Monitoring and Controlling System for Sericulture," SSRG International Journal of Electronics and Communication Engineering, vol. 7, no. 8, pp. 1-4, 2020. Crossref, https://doi.org/10.14445/23488549/IJECE-V7I8P101
[32] DingJu Zhu et al., “Knowledge Graph and Deep Learning Based Pest Detection and Identification System for Fruit Quality,” Internet of Things, p. 100649, 2020. Crossref, https://doi.org/10.1016/j.iot.2022.100649
[33] Geetha Lekshmy. V, P. A. Vishnu, and P. S. Harikrishnan, “Adaptive IoT System for Precision Agriculture,” Inventive Computation and Information Technologies, pp. 39-49, 2022. Crossref, http://dx.doi.org/10.1007/978-981-16-6723-7_4
[34] G. Murugesan, and B. Radha, "Crop Rotation based Crop Recommendation System with Soil Deficiency Analysis through Extreme Learning Machine," International Journal of Engineering Trends and Technology, vol. 70, no. 4, pp. 122-134, 2022. Crossref, https://doi.org/10.14445/22315381/IJETT-V70I4P210
[35] JiachenYang et al., “Data Quality Assessment and Analysis for Pest Identification in Smart Agriculture,” Computers and Electrical Engineering, vol. 103, p. 108322, 2022. Crossref, https://doi.org/10.1016/j.compeleceng.2022.108322