Conditional Super Resolution Generative Adversarial Network for Cervical Cell Image Enhancement
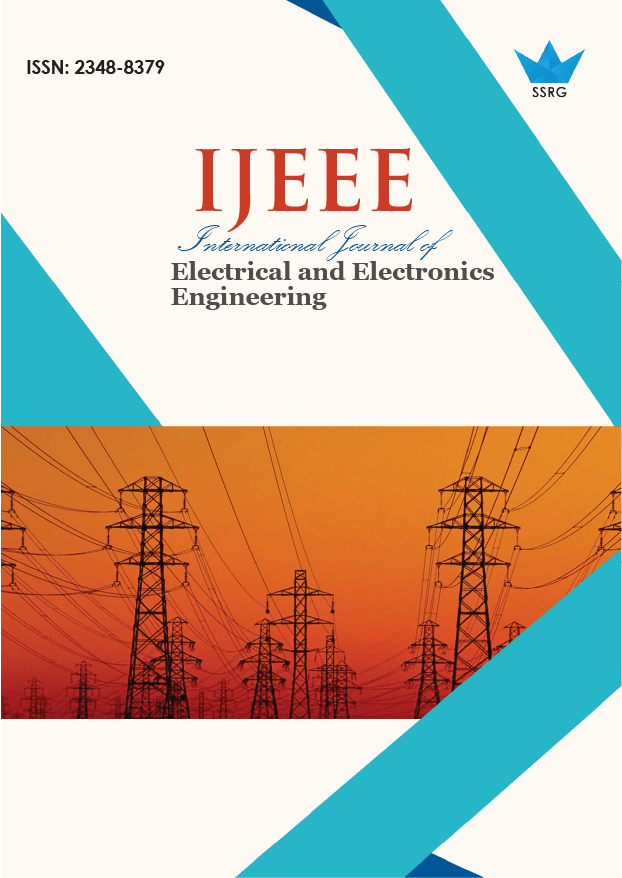
International Journal of Electrical and Electronics Engineering |
© 2023 by SSRG - IJEEE Journal |
Volume 10 Issue 4 |
Year of Publication : 2023 |
Authors : Janani S, D F X Christopher |
How to Cite?
Janani S, D F X Christopher, "Conditional Super Resolution Generative Adversarial Network for Cervical Cell Image Enhancement," SSRG International Journal of Electrical and Electronics Engineering, vol. 10, no. 4, pp. 70-76, 2023. Crossref, https://doi.org/10.14445/23488379/IJEEE-V10I4P107
Abstract:
Abnormal cellular development in the cervix causes cervical cancer. Undergoing Pap smear screening & HPV tests at regular intervals possibly helps identify the disease at an early stage, aiding diagnosis and treatment. Pathologists are involved in the screening to detect abnormal cells in the slide. Removing a portion of the slide with poor quality could improve nuclei detection by enabling better visualization of some important information. In this study, a Conditional Generative Adversarial Network (CGAN) with a Super Resolution technique is applied for cervical cell images to generate photo-realistic images. Poor-quality images are not removed; rather, they are converted into good-quality images. It ensures equal distribution of image generation in terms of classes in a given dataset. The performance of the recommended technique is measured using PSNR (Peak Signal-to-Noise Ratio), SSIM (Structural Similarity)and Inception Score(IS). The proposed methodology outperforms other augmentation techniques and Variational Autoencoders (VAE) based algorithms.
Keywords:
Augmentation, Cervical cells, CGAN, CNN, Super resolution.
References:
[1] World Health Organization Website, Cervical Cancer, 2022. [Online]. Available: https://www.who.int/news-room/fact-sheets/detail/human-papillomavirus-(hpv)-and-cervical-cancer
[2] J.C. Davies-Oliveira et al., “Eliminating Cervical Cancer: Progress and Challenges for High-income Countries,” Clinical Oncology, vol. 33, no. 9, pp. 550-559, 2021.
[CrossRef] [Google Scholar] [Publisher link]
[3] Amreen Abbas et al., “Tomato Plant Disease Detection using Transfer Learning with C-GAN Synthetic Images,” Computers and Electronics in Agriculture, vol. 187, p. 706279, 2021.
[CrossRef] [Google Scholar] [Publisher link]
[4] Syeda Sara Samreen, and Hakeem Aejaz Aslam, “Hyperspectral Image Classification using Deep Learning Techniques: A Review,” SSRG International Journal of Electronics and Communication Engineering, vol. 9, no. 6, pp. 1-4, 2022.
[CrossRef] [Publisher link]
[5] Christian Ledig et al., “Photo-realistic Single Image Super-resolution Using a Generative Adversarial Network,” IEEE Conference on Computer Vision and Pattern Recognition, pp. 105-114, 2017.
[CrossRef] [Google Scholar] [Publisher link]
[6] Alec Radford, Luke Metz, and Soumith Chintala, “Unsupervised Representation Learning with Deep Convolutional Generative Adversarial Networks,” arXiv preprint arXiv:1511.06434, 2015.
[CrossRef] [Google Scholar] [Publisher link]
[7] Lin Zhu et al., “Generative Adversarial Networks for Hyperspectral Image Classification,” IEEE Transactions on Geoscience and Remote Sensing, vol. 56, no. 9, pp. 5046-5063, 2018.
[CrossRef] [Google Scholar] [Publisher link]
[8] Hamid Eghbal-zadeh, and Gerhard Widmer, “Likelihood Estimation for Generative Adversarial Networks,” arXiv preprint arXiv:1707.07530, 2017.
[Publisher link]
[9] Qiang Yang, and Xiaokun Li, “BiGAN: LncRNA-Disease Association Prediction Based on Bidirectional Generative Adversarial Network,” BMC Bioinformatics, vol. 22, no. 1, 2021.
[CrossRef] [Google Scholar] [Publisher link]
[10] Shing Chan, and Ahmed H. Elsheikh, “Parametrization and Generation of Geological Models with Generative Adversarial Networks,” arXiv preprint arXiv:1708.01810, 2017.
[CrossRef] [Google Scholar] [Publisher link]
[11] Shabir Shah, and Humaira Tanvir, “Squamous Cell Carcinoma Associated With Chronic Use of Three Different Smokeless Tobacco,” SSRG International Journal of Medical Science, vol. 7, no. 8, pp. 1-3, 2020.
[CrossRef] [Publisher link]
[12] Poonam Chaudari, Himanshu Agrawal, and Ketan Kotecha, “Data Augmentation using MC-GAN for Improved Cancer Classification on Gene Expression Data,” Soft Computing, vol. 24, pp. 11381-11391, 2020.
[CrossRef] [Google Scholar] [Publisher link]
[13] Pin Wang et al., “Automatic Cell Nuclei Segmentation and Classification of Cervical Pap Smear Images,” Biomedical Signal Processing and Control, vol. 48, pp. 93–103, 2019.
[CrossRef] [Google Scholar] [Publisher link]
[14] Sookham R.P. Singh, Gurjit S. Randhawa, and Rajinder Singh Virk, “Efficacy of Genetic Algorithms for Staging Cervical Cancer,” International Journal of Cancer Research, vol.47, no. 2, p. 1164, 2013.
[Google Scholar]
[15] Jihe Zhu, Blagica Arsovska, and Kristina Kozovska, “Cervical Cancer – statistical Analysis Data in a 5year Period in Strumica and Republic of North Macedonia,” SSRG International Journal of Medical Science, vol. 7, no. 3, pp. 1-6, 2020.
[CrossRef] [Google Scholar] [Publisher link]
[16] Guo Chen, and Wenjian Zhang, “Application of Big Data Information System in Early Diagnosis, Treatment, and Nursing of Cervical Cancer Infected by Human Papillomavirus,” Expert Systems, vol. 40, no. 4, p. e 12791, 2021.
[CrossRef] [Google Scholar] [Publisher link]
[17] Siyu Chen et al., “Cervical Cancer Single Cell Image Data Augmentation Using Residual Condition Generative Adversarial Networks,” 3rd International Conference on Artificial Intelligence and Big Data (ICAIBD), 2020.
[CrossRef] [Google Scholar] [Publisher link]
[18] Ahmed Ghoneim, Ghulam Muhammad, and M. Shamim Hossain, “Cervical Cancer Classification using Convolutional Neural Networks and Extreme Learning Machines,” Future Generation Computer Systems, vol. 102, pp. 643-649, 2020.
[CrossRef] [Google Scholar] [Publisher link]
[19] Ruchika, Madhu Parmar, and Vinod Kumar Gupta, “Immune System Regulation with Cancer Vaccines Based on Dendritic Cells,” International Journal of Biotech Trends and Technology, vol. 9, no. 4, pp. 59-67, 2019.
[CrossRef] [Publisher link]
[20] Aditya Khamparia et al., “DCAVN: Cervical Cancer Prediction and Classification Using Deep Convolutional and Variational Autoencoder Network,” Multimedia Tools and Applications, vol. 80, pp. 30399-30415, 2021.
[CrossRef] [Google Scholar] [Publisher link]
[21] Joan Bruna, Pablo Sprechmann, and Yann LeCun, “Super-resolution with Deep Convolutional Sufficient Statistics,” arXiv preprint arXiv:1511.05666, 2015.
[CrossRef] [Google Scholar] [Publisher link]
[22] Asli Kale, and Selim Aksoy, “Segmentation of Cervical Cell Images,” 2010 20th International Conference on Pattern Recognition, 2010.
[CrossRef] [Google Scholar] [Publisher link]
[23] R. Surendiran et al., “Exploring the Cervical Cancer Prediction by Machine Learning and Deep Learning with Artificial Intelligence Approaches,” International Journal of Engineering Trends and Technology, vol. 70, no. 7, pp. 94-107, 2022.
[CrossRef] [Publisher link]
[24] R. Vidya, and G.M. Nasira, “Prediction of Cervical Cancer Using Hybrid Induction Technique: A Solution for Human Hereditary Disease Patterns,” Indian Journal of Science and Technology, vol. 9, no. 30, 2016.
[CrossRef] [Google Scholar]
[25] Kaggle, 2021. [Online]. Available: https://www.kaggle.com/datasets/prahladmehandiratta/cervical-cancer-largest-dataset-sipakmed
[26] Kaikai Liu et al., “Cells Image Generation Method based on VAE-SGAN,” Procedia Computer Science, vol. 183, pp. 589-595, 2021.
[CrossRef] [Google Scholar] [Publisher link]
[27] Mehdi Mirza, and Simon Osindero, “Conditional Generative Adversarial Nets,” arXiv preprint arXiv:1411.1784, 2014.
[CrossRef] [Google Scholar] [Publisher link]
[28] Qiufeng Wu, Yiping Chen, and Jun Meng, “DCGAN-based Data augmentation for Tomato Leaf Disease Identification,” IEEE Access, vol. 8, pp. 98716-98728, 2020.[CrossRef] [Google Scholar] [Publisher link]