Robust Adverse Drug Reaction Prediction and Classification by Employing Deer Hunting Optimization Driven Deep Learning Approach
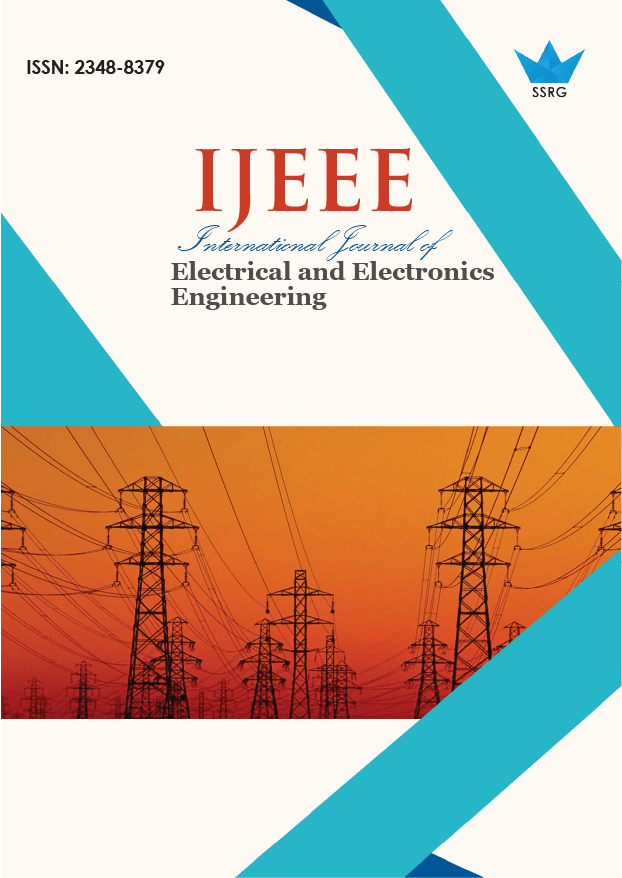
International Journal of Electrical and Electronics Engineering |
© 2023 by SSRG - IJEEE Journal |
Volume 10 Issue 5 |
Year of Publication : 2023 |
Authors : S. Nithinsha, S. Anusuya |
How to Cite?
S. Nithinsha, S. Anusuya, "Robust Adverse Drug Reaction Prediction and Classification by Employing Deer Hunting Optimization Driven Deep Learning Approach," SSRG International Journal of Electrical and Electronics Engineering, vol. 10, no. 5, pp. 48-59, 2023. Crossref, https://doi.org/10.14445/23488379/IJEEE-V10I5P105
Abstract:
Drugs for medical purpose aims to save a person’s life and improve the quality of life. They enhance one’s mental or physical fitness and treat disease. However, drugs might cause adverse reactions or even unexpected effects on patients, known as Adverse Drug Reactions (ADRs). For preventing ADRs, drug trials were carried out to evaluate and analyze the potential danger in the procedure of drug development. Deep learning (DL) is a type of Machine Learning (ML) in Artificial intelligence (AI) that has developed as a highly effective and promising method that can interrogate and combine diverse biological data kinds to produce new hypotheses. DL is extensively used in drug repurposing and discovery, but its application in ADR prediction employing gene expression data is limited. Therefore, this study introduces a Deer Hunting Optimization Driven Deep Learning Model for Robust Adverse Drug Reaction Recognition and Classification (DHODL-ADRRC) technique. The DHODL-ADRRC technique involves diverse phases of data preprocessing to normalize the data. In addition, the Binary Fire Hawks Optimization (BFHO) based feature selection (FS) method is used to elect an optimum set of features. Moreover, the Attention-based Convolutional Bidirectional Long Short-Term Memory (ACBLSTM) algorithm is utilized for ADR identification. Furthermore, the DHO model is exploited for the tuning process adjusting of the ACBLSTM model, advancing the classification outputs. The simulation output of the DHODL-ADRRC algorithm was investigated on the ADR dataset, and the extensive outputs highlighted the advanced achievement of the DHODL-ADRRC method over other current methods in terms of different measures.
Keywords:
Adversary drug reaction, Artificial intelligence, Feature selection, Deer hunting optimizer, Deep learning.
References:
[1] Zehong Zhang et al., “Graph Neural Network Approaches for Drug-Target Interactions,” Current Opinion in Structural Biology, vol. 73, pp. 102327, 2022.
[CrossRef] [Google Scholar] [Publisher Link]
[2] Chang Sun, “A Deep Neural Network-based Co-coding Method to Predict Drug-protein Interactions by Analyzing the Feature Consistency between Drugs and Proteins,” IEEE/ACM Transactions on Computational Biology and Bioinformatics, 2023.
[CrossRef] [Google Scholar] [Publisher Link]
[3] Hossein Sharifi-Noghabi et al., “MOLI: Multi-Omics Late Integration with Deep Neural Networks for Drug Response Prediction,” Bioinformatics, vol. 35, no. 14, pp. i501-i509, 2019.
[CrossRef] [Google Scholar] [Publisher Link]
[4] Natarajan Yuvaraj et al., “Analysis of Protein-Ligand Interactions of SARS-Cov-2 Against Selective Drug using Deep Neural Networks,” Big Data Mining and Analytics, vol. 4, no. 2, pp. 76-83, 2021.
[CrossRef] [Google Scholar] [Publisher Link]
[5] Yang Li et al., “Drug Target Interaction Predication via Multi-Channel Graph Neural Networks,” Briefings in Bioinformatics, vol. 23, no. 1, pp. bbab346, 2022. [CrossRef] [Google Scholar] [Publisher Link]
[6] X. Xiao et al., “MPCDDI: A Secure Multiparty Computation-Based Deep Learning Framework for Drug-Drug Interaction Predictions,” Bioinformatics Research and Applications, vol. 13760, 2022.
[CrossRef] [Google Scholar] [Publisher Link]
[7] Rabia Javed et al., “An Efficient Pattern Recognition Based Method for Drug-Drug Interaction Diagnosis,” 2021 1st International Conference on Artificial Intelligence and Data Analytics (CAIDA), Riyadh, Saudi Arabia, pp. 221-226, 2021.
[CrossRef] [Google Scholar] [Publisher Link]
[8] Shichao Liu et al., “Enhancing Drug-Drug Interaction Prediction using Deep Attention Neural Networks,” IEEE/ACM Transactions on Computational Biology and Bioinformatics, vol. 20, no. 2, pp. 976-985, 2023.
[CrossRef] [Google Scholar] [Publisher Link]
[9] E. G. Swetala, P. Sujatha, and P. Bharath, “Automatic Load Frequency Control for Wind-Thermal Micro Grid Based on Deep Reinforcement Learning,” SSRG International Journal of Electrical and Electronics Engineering, vol. 8, no. 8, pp. 1-8, 2021.
[CrossRef] [Publisher Link]
[10] G. P. Dimf, P. Kumar, and K. Paul Joshua, “CNN with BI-LSTM Electricity Theft Detection based on Modified Cheetah Optimization Algorithm in Deep Learning,” SSRG International Journal of Electrical and Electronics Engineering, vol. 10, no. 2, pp. 35-43, 2023.
[CrossRef] [Publisher Link]
[11] An Huang et al., “A Multimodal Data Fusion-Based Deep Learning Approach for Drug-Drug Interaction Prediction,” Bioinformatics Research and Applications: 18th International Symposium, pp. 275-285, 2023.
[CrossRef] [Google Scholar] [Publisher Link]
[12] Xiaorui Su et al., “Biomedical Knowledge Graph Embedding with Capsule Network for Multi-Label Drug-Drug Interaction Prediction,” IEEE Transactions on Knowledge and Data Engineering, vol. 35, no. 6, pp. 5640-5651, 2023.
[CrossRef] [Google Scholar] [Publisher Link]
[13] Jaesub Park et al., Large-Scale Prediction of Adverse Drug Reactions-Related Proteins with Network Embedding,” Bioinformatics, vol. 39, no. 1, pp. 1-9, 2023.
[CrossRef] [Google Scholar] [Publisher Link]
[14] Shanchen Pang et al., “AMDE: A Novel Attention-Mechanism-Based Multidimensional Feature Encoder for Drug–Drug Interaction Prediction,” Briefings in Bioinformatics, vol. 23, no. 1, pp. bbab545, 2022.
[CrossRef] [Google Scholar] [Publisher Link]
[15] Nair Bini Balakrishnan, P.S. Sreeja, and Jisha Jose Panackal, “Alzheimer’s Disease Diagnosis using Machine Learning: A Review,” International Journal of Engineering Trends and Technology, vol. 71, no. 3, pp. 120-129, 2023.
[CrossRef] [Publisher Link]
[16] Chi-Shiang Wang et al., “Detecting Potential Adverse Drug Reactions using A Deep Neural Network Model,” Journal of medical Internet Research, vol. 21, no. 2, 2019.
[CrossRef] [Google Scholar] [Publisher Link]
[17] Brandon Fan et al., “Adverse Drug Event Detection and Extraction from Open Data: A Deep Learning Approach,” Information Processing & Management, vol. 57, no. 1, 2020.
[CrossRef] [Google Scholar] [Publisher Link]
[18] Jiajing Zhu et al., “DAEM: Deep Attributed Embedding Based Multi-Task Learning for Predicting Adverse Drug–Drug Interaction,” Expert Systems with Applications, vol. 215, 2023.
[CrossRef] [Google Scholar] [Publisher Link]
[19] Arnold K. Nyamabo et al., “Drug–Drug Interaction Prediction with Learnable Size-Adaptive Molecular Substructures,” Briefings in Bioinformatics, vol. 23, no. 1, 2022.
[CrossRef] [Google Scholar] [Publisher Link]
[20] Christopher McMaster et al., “Developing a Deep Learning Natural Language Processing Algorithm for Automated Reporting of Adverse Drug Reactions,” Journal of Biomedical Informatics, vol. 137, 2023.
[CrossRef] [Google Scholar] [Publisher Link]
[21] Chun Yen Lee, and Yi-Ping Phoebe Chen, “Prediction of Drug Adverse Events Using Deep Learning in Pharmaceutical Discovery,” Briefings in Bioinformatics, vol. 22, no. 2, pp. 1884–1901, 2021.
[CrossRef] [Google Scholar] [Publisher Link]
[22] Fenia Christopoulou et al., “Adverse Drug Events and Medication Relation Extraction in Electronic Health Records with Ensemble Deep Learning Methods,” Journal of the American Medical Informatics Association, vol. 27, no. 1, pp. 39-46, 2020.
[CrossRef] [Google Scholar] [Publisher Link]
[23] Mohammed Alonazi, and Mrim M. Alnfiai, “Fire Hawk Optimizer with Deep Learning Enabled Human Activity Recognition,” Computer Systems Science and Engineering, vol. 45, no.3, pp. 3135–3150, 2023.
[CrossRef] [Publisher Link]
[24] Jianqing Wu et al., “Towards Attention-Based Convolutional Long Short-Term Memory for Travel Time Prediction of Bus Journeys,” Sensors, vol. 20, no. 12, 2020.
[CrossRef] [Google Scholar] [Publisher Link]
[25] S. R. Kanna, K. Sivakumar, and N. Lingaraj, “Development of Deer Hunting Linked Earthworm Optimization Algorithm for Solving Large Scale Traveling Salesman Problem,” Knowledge-Based Systems, vol. 227, 2021.
[CrossRef] [Google Scholar] [Publisher Link]
[26] S. Sandhiya, and U. Palani, “A Novel Hybrid PSBCO Algorithm for Feature Selection,” International Journal of Computer and Organization Trends, vol. 10, no. 3, pp. 21-26, 2020.
[Google Scholar] [Publisher Link]
[27] Jhih-Yuan Huang,Wei-Po Lee, and King-Der Lee, “Predicting Adverse Drug Reactions from Social Media Posts: Data Balance, Feature Selection and Deep Learning,” Healthcare, vol. 10, no. 4, pp. 618, 2022.
[CrossRef] [Google Scholar] [Publisher Link]
[28] Wongpanya S. Nuankaew et al., “Forecasting Graduation Schedule Model of Higher Education Learners using Feature Selection Techniques,” International Journal of Engineering Trends and Technology, vol. 71, no. 4, pp. 354-358, 2023.
[CrossRef] [Publisher Link]