Effective Breast Cancer Prediction Based on Feature Extraction, Fusion And Selection using Hybrid Methodologies
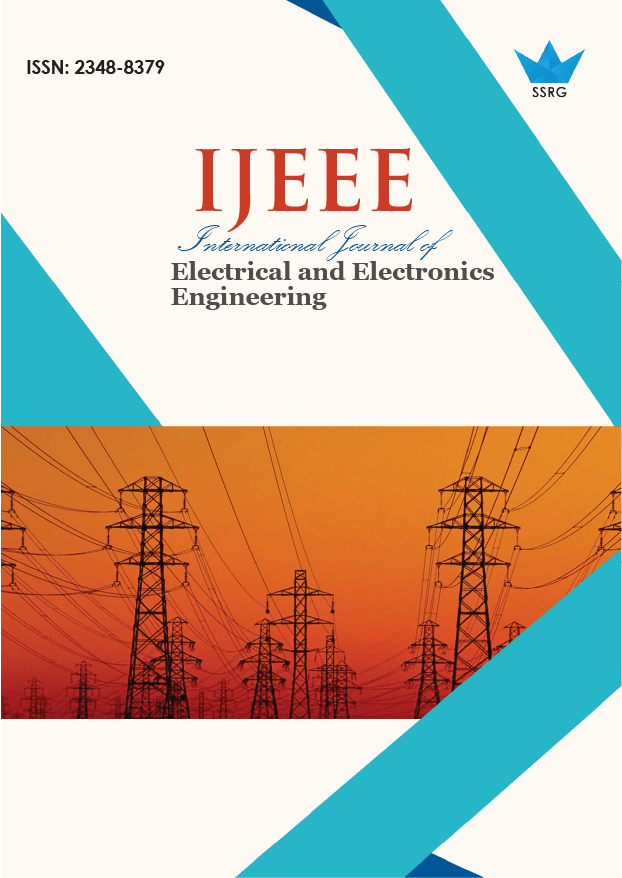
International Journal of Electrical and Electronics Engineering |
© 2023 by SSRG - IJEEE Journal |
Volume 10 Issue 5 |
Year of Publication : 2023 |
Authors : G. Rajasekaran, C. Sunitha Ram |
How to Cite?
G. Rajasekaran, C. Sunitha Ram, "Effective Breast Cancer Prediction Based on Feature Extraction, Fusion And Selection using Hybrid Methodologies," SSRG International Journal of Electrical and Electronics Engineering, vol. 10, no. 5, pp. 131-142, 2023. Crossref, https://doi.org/10.14445/23488379/IJEEE-V10I5P112
Abstract:
Women have been affected by many diseases for decades due to their low immune systems. Especially breast cancer is the second largest and most harmful disease for a woman that, leads to death. The earlier prediction of breast cancer can help for easy treatment and Save lives. Deep Learning methods are implemented in medical fields to attain an effective prediction and higher prediction accuracy. Therefore, in this work, the novel hybrid prediction topology is implemented two feature extractions, feature fusion, feature selection and classification with several algorithms such as Linear Discriminant Analysis (LDA), Canonical Correlation Analysis (CCA), Convolutional Neural Network (CNN) Long Short-Term Memory (LSTM), Transit Search Optimization (TSO), Support Vector Machine (SVM) respectively. The LDA and CNN-LSTM method is used to execute a two-feature extraction, and the CCA model is used to merge the two extracted features, a feature fusion task. Next, the TSO-based feature selection is implemented to minimize the redundant features. At last, the classification is carried out using an SVM method to acquire an efficient breast cancer prediction. The proposed hybrid method has achieved more accurate decision-making and efficient training than the conventional methods. The experimental result of the novel hybrid method acquired a superior performance in terms of precision, Recall, Accuracy, F1 Score, RMSE and MAE as 98.81%, 99.02%, 98.18%, 98.85%, 1.001 and 1.016, respectively.
Keywords:
Breast cancer prediction, Feature extraction, ML/DL methods, CCA based feature fusion, TSO-based selection, Classification, Performance accuracy.
References:
[1] Ahmedin Jema et al., “Cancer Statistics 2005,” CA: A Cancer Journal for Clinicians, vol. 55, no. 1, pp. 10-30, 2005.
[Google Scholar][Publisher Link]
[2] Kemal Polat, and Salih Güneş, “Breast Cancer Diagnosis using Least Square Support Vector Machine,” Digital Signal Processing, vol. 17, no. 4, pp. 694-701, 2007.
[CrossRef][Google Scholar][Publisher Link]
[3] Mehmet Fatih Akay, “Support Vector Machines Combined with Feature Selection for Breast Cancer Diagnosis,” Expert systems with Applications, vol. 36, no. 2, pp. 3240-3247, 2009.
[CrossRef][Google Scholar][Publisher Link]
[4] Wei-Chang Yeh, Wei-Wen Chang, and Yuk Ying Chung, “A New Hybrid Approach for Mining Breast Cancer Pattern using Discrete Particle Swarm Optimization and Statistical Method,” Expert Systems with Applications, vol. 36, no. 4, pp. 8204-8211, 2009.
[CrossRef][Google Scholar][Publisher Link]
[5] A. Marcano-Cedeño, J. Quintanilla-Domínguez, and D. Andina, “WBCD Breast Cancer Database Classification Applying Artificial Meta Plasticity Neural Network,” Expert Systems with Applications, vol. 38, no. 8, pp. 9573-9579, 2011.
[CrossRef][Google Scholar][Publisher Link]
[6] Yılmaz Kaya, and Murat Uyar, “A Hybrid Decision Support System Based on Rough Set and Extreme Learning Machine for Diagnosis of Hepatitis Disease,” Applied Soft Computing, vol. 13, no. 8, pp. 3429-3438.
[CrossRef][Google Scholar][Publisher Link]
[7] Kindie Biredagn Nahato, Khanna Nehemiah Harichandran, and Kannan Arputharaj, “Knowledge Mining from Clinical Datasets using Rough Sets And Back Propagation Neural Network,” Computational and Mathematical Methods in Medicine, vol. 2015, pp. 1-13, 2015.
[CrossRef][Google Scholar][Publisher Link]
[8] Lijuan Liu, and Mingrong Deng, “An Evolutionary Artificial Neural Network Approach for Breast Cancer Diagnosis,” In Third International Conference on Knowledge Discovery and Data Mining, pp. 593-596, 2010.
[CrossRef][Google Scholar][Publisher Link]
[9] Hui-Ling Chen et al., “A Support Vector Machine Classifier with Rough Set-Based Feature Selection for Breast Cancer Diagnosis,” Expert Systems with Applications, vol. 38, no. 7, pp. 9014-9022, 2011.
[CrossRef][Google Scholar][Publisher Link]
[10] Noreen Fatima et al., “Prediction of Breast Cancer, Comparative Review of Machine Learning Techniques, and Their Analysis,” IEEE Access, vol. 8, pp. 150360-150376, 2020.
[CrossRef][Google Scholar][Publisher Link]
[11] Min-Wei Huang et al., “SVM and SVM Ensembles in Breast Cancer Prediction,” PloS One, vol. 12, no. 1, pp. e0161501, 2017.
[CrossRef][Google Scholar][Publisher Link]
[12] Yixuan Li, and Zixuan Chen, “Performance Evaluation of Machine Learning Methods for Breast Cancer Prediction,” Applied and Computational Mathematics, vol. 7, no. 4, pp. 212-216, 2018.
[CrossRef][Google Scholar][Publisher Link]
[13] K. Sivakami, “Mining Big Data: Breast Cancer Prediction using DT-SVM Hybrid Model,” International Journal of Scientific Engineering and Applied Science, vol. 1, no. 5, pp. 418-429, 2015.
[Google Scholar][Publisher Link]
[14] Jayesh George Melekoodappattu, and Perumal Sankar Subbian, “Automated Breast Cancer Detection using Hybrid Extreme Learning Machine Classifier,” Journal of Ambient Intelligence and Humanized Computing, vol. 14, pp. 5489-5498, 2023.
[CrossRef][Google Scholar][Publisher Link]
[15] Ilangovan Sangaiah, and A. Vincent Antony Kumar, “Improving Medical Diagnosis Performance using Hybrid Feature Selection via Relief and Entropy-Based Genetic Search (RF-EGA) Approach: Application to Breast Cancer Prediction,” Cluster Computing, vol. 22, no. 3, pp. 6899-6906, 2019.
[CrossRef][Google Scholar][Publisher Link]
[16] Tze Sheng Lim et al., “Breast Cancer Diagnosis System using Hybrid Support Vector Machine-Artificial Neural Network,” International Journal of Electrical and Computer Engineering, vol. 11, no. 4, pp. 3059-3069, 2021.
[CrossRef][Google Scholar][Publisher Link]
[17] Fang Chen et al., “Immunohisto Chemical Index Prediction of Breast Cancer by using Hybrid Ultrasound Data," In IEEE Transactions on Biomedical Engineering, vol. 70, no. 4, pp. 1401-1412, 2023.
[CrossRef][Google Scholar][Publisher Link]
[18] R. Senkamalavalli, and T. Bhuvaneswari, “Improved Classification of Breast Cancer Data using Hybrid Techniques,” International Journal of Advanced Engineering Research and Science, vol. 5, no. 5, pp. 77-81, 2017.
[CrossRef][Google Scholar][Publisher Link]
[19] Shobha Gusain, “Modifiable Risk Factors for Breast and Cervical Cancer and their Association with Sample Characteristics,” SSRG International Journal of Nursing and Health Science, vol. 6, no. 1, pp. 10-16, 2020.
[CrossRef][Publisher Link]
[20] Shankar Thawkar et al., “Breast Cancer Prediction using A Hybrid Method Based on Butterfly Optimization Algorithm and Ant Lion Optimizer,” Computers in Biology and Medicine, vol. 139, pp. 104968, 2021.
[CrossRef][Google Scholar][Publisher Link]
[21] Jayesh George Melekoodappattu, Perumal Sankar Subbian, and M. P. Flower Queen, “Detection and Classification of Breast Cancer from Digital Mammograms using Hybrid Extreme Learning Machine Classifier,” International Journal of Imaging Systems and Technology, vol. 31, no. 2, pp. 909-920, 2021.
[CrossRef][Google Scholar][Publisher Link]
[22] Jong Pill Choi1, Tae Hwa Han, and Rae Woong Park, “A Hybrid Bayesian Network Model for Predicting Breast Cancer Prognosis,” Journal of Korean Society of Medical Informatics, vol. 15, no. 1, pp. 49-57, 2009.
[CrossRef][Google Scholar][Publisher Link]
[23] Mohammad R. Mohebian et al., “A Hybrid Computer-Aided-Diagnosis System for Prediction of Breast Cancer Recurrence (HPBCR) using Optimized Ensemble Learning,” Computational and Structural Biotechnology Journal, vol. 15, pp. 75-85, 2017.
[CrossRef][Google Scholar][Publisher Link]
[24] Youness Aliyari Ghassabeh, Frank Rudzicz, and Hamid Abrishami Moghaddam, “Fast Incremental LDA Feature Extraction,” Pattern Recognition, vol. 48, no. 6, pp. 1999-2012, 2015.
[CrossRef][Google Scholar][Publisher Link]
[25] Almas Begum et al., “A Combined Deep CNN: LSTM with a Random Forest Approach for Breast Cancer Diagnosis,” Complexity, vol. 2022, no. 1-9, 2022.
[CrossRef][Google Scholar][Publisher Link]
[26] Mariam Shadan et al., “Histological Categorization of Stromal Desmoplasia in Breast Cancer and Its Diagnostic and Prognostic Utility,” SSRG International Journal of Medical Science, vol. 4, no. 6, pp. 8-11, 2017.
[CrossRef][Google Scholar][Publisher Link]
[27] B. Johnson, P. Keerthi Vasan, and V. Thillaivendan, “Performance of Hyperthermia for Breast Cancer,” SSRG International Journal of Applied Physics, vol. 3, no. 2, pp. 1-5, 2016.
[CrossRef][Publisher Link]
[28] Masoomeh Mirrashid, and Hosein Naderpour, “Transit Search: An Optimization Algorithm Based on Exoplanet Exploration,” Results in Control and Optimization, vol. 7, 2022.
[CrossRef][Google Scholar][Publisher Link]
[29] G. Ravi Kumar, G. A. Ramachandra, and K. Nagamani, “An Efficient Prediction of Breast Cancer Data using Data Mining Techniques,” International Journal of Innovations in Engineering and Technology, vol. 2, no. 4, pp. 139-144, 2013.
[Google Scholar][Publisher Link]
[30] Brotobor Deliverance, Edeawe Isaac Osahogie, and Brotobor Onoriode, “Awareness and Practice of Student Nurses on Breast Self-Examination: A Risk Assessment Tool for Breast Cancer,” SSRG International Journal of Nursing and Health Science, vol. 6, no. 1, pp. 66-69, 2020.
[CrossRef][Publisher Link]
[31] Nidhi Mongoriya, and Vinod Patel, “Review the Breast Cancer Detection Technique using Hybrid Machine Learning,” SSRG International Journal of Computer Science and Engineering, vol. 8, no. 6, pp. 5-8, 2021.
[CrossRef][Publisher Link]
[32] M. Hossin, and M. N. Sulaiman, “A Review on Evaluation Metrics for Data Classification Evaluations,” International Journal of Data Mining and Knowledge Management Process, vol. 5, no. 2, pp. 1-11, 2015.
[CrossRef][Publisher Link]