Residual U-Net Architecture for Retinal Layers in OCT Images with Choroidal Neovascularization
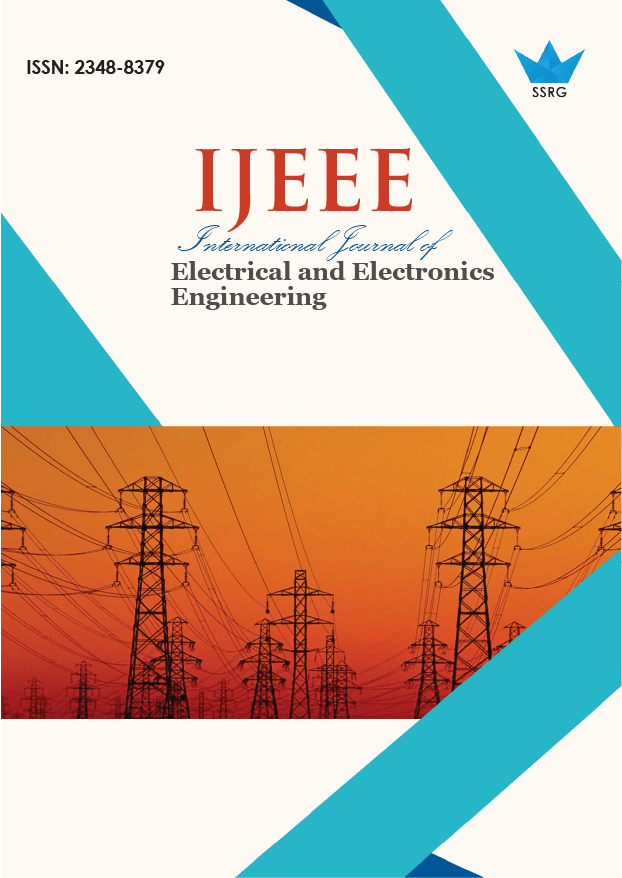
International Journal of Electrical and Electronics Engineering |
© 2023 by SSRG - IJEEE Journal |
Volume 10 Issue 5 |
Year of Publication : 2023 |
Authors : B. Miriam Zipporah, D. Francis Xavier Christopher |
How to Cite?
B. Miriam Zipporah, D. Francis Xavier Christopher, "Residual U-Net Architecture for Retinal Layers in OCT Images with Choroidal Neovascularization," SSRG International Journal of Electrical and Electronics Engineering, vol. 10, no. 5, pp. 205-212, 2023. Crossref, https://doi.org/10.14445/23488379/IJEEE-V10I5P119
Abstract:
U-Net is adequate for various biomedical image segmentation tasks. Using this in the Age-related Macular Degeneration (AMD) images would be better for segmenting only the affected region. The variant of U-Net uses residual connections. A residual link is a type of connection that allows the network to learn the residual mapping between the input and output rather than the complete mapping. This technique can help the network learn more efficiently and avoid the vanishing gradients problem. This research aims to develop a method for segmenting the retinal pigment epithelium, the Bruch’s membrane, and the Inner Limiting Membranes (ILM) in OCT scans of individuals in good health and with intermediate AMD.
Keywords:
Age-related macular degeneration, Inner limiting membrane, Optical coherence tomography, U-net.
References:
[1] Dr. Fabiola Esther Flores Arredondo, Macular Degeneration: Age 60 a Risk Factor, 2019. [Online]. Available: https://www.topdoctors.mx/doctor/fabiola-esther-flores-arredondo#
[2] Dr. Alfonso Dupinet Sánchez, Macular Degeneration, How does AMD Affect Your Sight?, 2021.
[3] Jefferson Alves Sous et al., “Automatic Segmentation of Retinal Layers in OCT Images with Intermediate Age-Related Macular Degeneration Using U-Net and Dexined,” PloS One, vol. 16, no. 5, pp.1-16, 2021.
[CrossRef] [Google Scholar] [Publisher Link]
[4] Jackson Scharf et al., “Optical Coherence Tomography Angiography of the Choriocapillaris in Age-Related Macular Degeneration,” Journal of Clinical Medicine, vol. 10, no. 4, pp. 1-16, 2021.
[CrossRef] [Google Scholar] [Publisher Link]
[5] X. Xu et al., “Automated Age-Related Macular Degeneration Detection and Lesion Segmentation in Optical Coherence Tomography Images Using Deep Learning,” Journal of Medical Systems, vol. 44, p. 104, 2020.
[6] Feng Liu et al., “Auxiliary Segmentation Method of Osteosarcoma MRI Image Based on Transformer and U-Net.” Computational Intelligence and Neuroscience, 2022.
[CrossRef] [Google Scholar] [Publisher Link]
[7] Nahian Siddique et al., “U-Net and its Variants for Medical Image Segmentation: Theory and Applications,” arXiv preprint arXiv: 2011.01118, 2020.
[CrossRef] [Google Scholar] [Publisher Link]
[8] H. Li et al., “ResUNet-aux: A Deep Learning Framework for Automatic Segmentation of Geographic Atrophy in Fundus Autofluorescence Images,” Medical Image Analysis, vol. 69, 2021.
[9] Ayoub Skouta et al., “Haemorrhage Semantic Segmentation in Fundus Images for the Diagnosis of Diabetic Retinopathy by Using a Convolutional Neural Network,” Journal of Big Data, vol. 9, no. 1, pp. 1-24, 2022.
[CrossRef] [Google Scholar] [Publisher Link]
[10] G Jeyalakshmi, and K.A Shahul Hameed, “Advanced Approaches to Brain Tumor Classification and Diagnosis,” SSRG International Journal of Electronics and Communication Engineering, vol. 9, no. 1, pp. 6-9, 2022.
[CrossRef] [Publisher Link]
[11] Olaf Ronneberger, Philipp Fischer, and Thomas Brox, “U-Net: Convolutional Networks for Biomedical Image Segmentation,” Medical Image Computing and Computer-Assisted Intervention–MICCAI 2015: 18th International Conference, Munich, Germany, Springer International Publishing, pp. 234-241, 2015.
[CrossRef] [Google Scholar] [Publisher Link]
[12] Malik A. Manan et al., “A Residual Encoder-Decoder Network for Segmentation of Retinal Image-Based Exudates in Diabetic Retinopathy Screening,” arXiv preprint arXiv:2201.05963, 2022.
[CrossRef] [Google Scholar] [Publisher Link]
[13] Ning Wang et al., “Improvement of Retinal Vessel Segmentation Method Based on U-Net,” Electronics, vol. 12, no. 2, p. 1-14, 2023.
[CrossRef] [Google Scholar] [Publisher Link]
[14] Y. Li et al., “Automatic Drusen and Geographic Atrophy Segmentation for Age-Related Macular Degeneration Using Multi-Scale Deep Residual Network,” IEEE Access, vol. 9, pp. 32146-32155, 2021.
[15] Introduction to Residual Networks – GeeksforGeeks, GeeksforGeeks. [Online]. Available. https://www.geeksforgeeks.org/introduction-to-residual-networks/ [16] Zhengxin Zhang, and Qingjie Liu, “Road Extraction by Deep Residual U-Net,” IEEE Geoscience and Remote Sensing Letters, vol. 15, no. 5, pp. 749-753, 2018.
[CrossRef] [Google Scholar] [Publisher Link]
[17] Lohitha Boyina et al., “Classification of Uncertain ImageNet Retinal Diseases using ResNet Model,” International Journal of Intelligent Systems and Applications in Engineering, vol. 10, no. 2s, pp. 35-42, 2022.
[CrossRef] [Publisher Link]
[18] Jeremy Zhang, UNet Line by Line Explanation, Medium, 2023. [Online]. Available: https://towardsdatascience.com/unet-line-by-line-explanation-9b191c76baf5 [19] Harshall Lamba, Understanding Semantic Segmentation with UNET, Medium, 2023. [Online]. Available: https://towardsdatascience.com/understading-semantic-segmentation-with-unet-6be4f42d4b47
[20] Nikhil Tomar, RESUNET Implementation inPyTorch - Idiot Developer, RESUNET Implementation in PyTorch - Idiot Developer, 2023. [Online]. Available: https://idiotdeveloper.com/resunet-implementation-in-pytorch/
[21] Gracelyn Shi, Implementing a ResNet Model from Scratch, Medium, 2023. [Online]. Available: https://towardsdatascience.com/implementing-a-resnet-model-from-scratch-971be7193718
[22] Y. Wei et al., “DRIS-Net: A Hybrid Deep Retinal Image Segmentation Network,” IEEE Transactions on Medical Imaging, vol. 39, no. 9, pp. 2795-2806, 2020.
[23] Keerti Maithil, and Tasneem Bano Rehman, "Urban Remote Sensing Image Segmentation using Dense U-Net+," SSRG International Journal of Computer Science and Engineering, vol. 9, no. 3, pp. 21-28, 2022.
[CrossRef] [Google Scholar] [Publisher Link]
[24] Y. Zhang et al., “Retinal U-Net: Embarrassingly Simple Exploitation of Segmentation Supervision for Medical Object Detection,” IEEE Transactions on Medical Imaging, vol. 39, no. 9, pp. 3108-3119, 2020.
[Google Scholar]
[25] Pallavi Hallappanavar Basavaraja, and Shanmugarathinam Ganesarathinam, "Weighted DenseNet-121 for Osteoporosis Disease Detection using X-ray Images," International Journal of Engineering Trends and Technology, vol. 70, no. 3, pp. 266-274, 2022.
[CrossRef] [Publisher Link]
[26] S. Parveen Banu, and M. Syed Mohamed, “Asial CNN: Assorted Scale Integrated Alternate Link Model Convolutional Neural Network for Lung Nodule Detection,” International Journal of Engineering Trends and Technology, vol. 70, no. 11, pp. 353-363, 2022.
[CrossRef] [Publisher Link]
[27] Doha Bouallal, Hassan Douzi, and Rachid Harba, “Diabetic Foot Thermal Image Segmentation using Double Encoder-ResUnet (DE-ResUnet),” Journal of Medical Engineering & Technology, vol. 46, no. 5, pp. 378-392, 2022.
[CrossRef] [Google Scholar] [Publisher Link]
[28] Kaiming He et al., “Deep Residual Learning for Image Recognition,” Proceedings of the IEEE Conference on Computer Vision and Pattern Recognition, pp. 770-778, 2016.
[Google Scholar] [Publisher Link]
[29] J. Zhang, L. Wang, and Y. Chen, “RA-U-Net: A Residual Attention U-Net Deep Convolutional Network for Retinal Vessel Segmentation,” Journal of Healthcare Engineering, 2020.