Deep Learning Approach for the Classification of Caprine Parasites
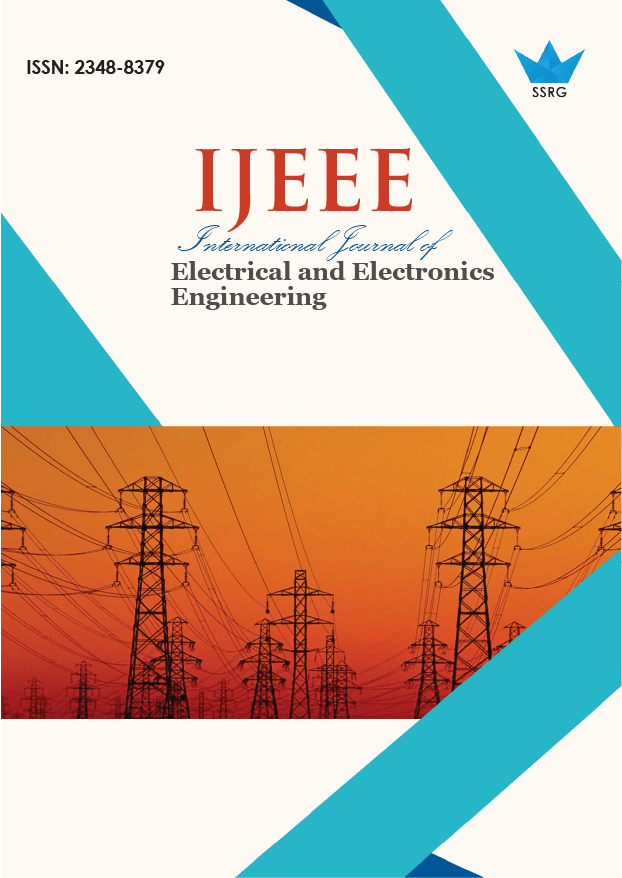
International Journal of Electrical and Electronics Engineering |
© 2023 by SSRG - IJEEE Journal |
Volume 10 Issue 9 |
Year of Publication : 2023 |
Authors : B. LakshmiNarayana Reddy, B. Keerthi Priya, K. Rajendra Prasad, A.Daisy Rani, D.V.Rama Koti Reddy |
How to Cite?
B. LakshmiNarayana Reddy, B. Keerthi Priya, K. Rajendra Prasad, A.Daisy Rani, D.V.Rama Koti Reddy, "Deep Learning Approach for the Classification of Caprine Parasites," SSRG International Journal of Electrical and Electronics Engineering, vol. 10, no. 9, pp. 190-205, 2023. Crossref, https://doi.org/10.14445/23488379/IJEEE-V10I9P118
Abstract:
A parasitic infection is, by far and away, the most common element in animal infectious diseases whose origins may be traced back to most places worldwide. The term "parasite" refers to any organism that lives within another living thing. Because of the near closeness of the people and the animals, there is a danger that the sanitary conditions of the animals will not be adequately treated, which has the potential to have severe ramifications for the health of the people. The surrounding environment has become contaminated due to the spread of parasites and bacteria that dwell in the digestive systems of a wide variety of different animals into the surrounding environment. This has resulted in the contamination of the ecosystem. The main objective of this paper is to employ numerous methods of deep learning to identify the types of parasites found in faeces samples taken from caprine animals. The caprine animals served as the source for the later analyzed samples. Images of parasites such as Amphistome, Ascaris, B-Coli, Moniezia, Schistosoma spindale, Strongyle, and Trichuris are included in the database. These images train and evaluate several models using deep learning techniques such as YOLOv4, YOLOv5, SSD and CNN. The classification results from the study indicate that the YOLOv4 technique can diagnose infections caused by caprine parasites more promptly. This was determined by looking at the results of the performed classification. The fact that the approach produced categorization results provides support for this hypothesis.
Keywords:
Parasitic worm, Feces, Deep learning, Amphistome, Strongyle, YOLOv5, CNN.
References:
[1] P.C. Sarmah et al., “Human Consumption of Rumen Flukes of Cattle in India,” Southeast Asian Journal of Tropical Medicine and Public Health, vol. 45, no. 1, pp. 26-30, 2014.
[Google Scholar] [Publisher Link]
[2] Johannes Charlier et al., “Chasing Helminths and their Economic Impact on Farmed Ruminants,” Trends in Parasitology, vol. 30, no. 7, pp. 361-367, 2014.
[CrossRef] [Google Scholar] [Publisher Link]
[3] Pinar Okyay et al., “Intestinal Parasites Prevalence and Related Factors in School Children, a Western City Sample - Turkey,” BMC Public Health, vol. 4, no. 64, pp. 1-6, 2004.
[CrossRef] [Google Scholar] [Publisher Link]
[4] Norman R. Stoll, “This Wormy World,” Journal of Parasitology, vol. 33, no. 1, pp. 1-18, 1947.
[Google Scholar] [Publisher Link]
[5] Peter J. Hotez et al., “Helminth Infections: The Great Neglected Tropical Diseases,” Journal of Clinical Investigation, vol. 118, no. 4, pp. 1311-1321, 2008.
[CrossRef] [Google Scholar] [Publisher Link]
[6] Pascal Del Giudice et al., “Autochthonous Cutaneous Larva Migrans in France and Europe,” Acta Dermato-Venereologica, vol. 99, no. 9, pp. 805-808, 2019.
[CrossRef] [Google Scholar] [Publisher Link]
[7] A. Jardim-Botelho et al., “Hookworm, Ascaris Lumbricoides Infection and Polyparasitism Associated with Poor Cognitive Performance in Brazilian Schoolchildren,” Tropical Medicine and International Health, vol. 13, no. 8, pp. 994-1004, 2008.
[CrossRef] [Google Scholar] [Publisher Link]
[8] Smita Gupta et al., “Balantidium Coli: Rare Urinary Pathogen or Fecal Contaminant in Urine? Case Study and Review,” IOSR Journal of Dental and Medical Sciences, vol. 16, no. 3, pp. 88-90, 2017.
[CrossRef] [Google Scholar] [Publisher Link]
[9] Fazly Ann Zainalabidin et al., “Monieziasis in Domestic Ruminants in Perak, Malaysia,” Songklanakarin Journal of Science and Technology, vol. 43, no. 1, pp. 218-221, 2021.
[CrossRef] [Google Scholar] [Publisher Link]
[10] Sara Lustigman et al., “A Research Agenda for Helminth Diseases of Humans: The Problem of Helminthiases,” Plos Neglected Tropical Diseases, vol. 6, no. 4, pp. 1-13, 2012.
[CrossRef] [Google Scholar] [Publisher Link]
[11] Martin K. Nielsen, Craig R. Reinemeyer, and Debra C. Sellon, Chapter 57 – Nematodes, Equine Infectious Diseases, Elsevier, pp. 475-489, 2014.
[CrossRef] [Google Scholar] [Publisher Link]
[12] A. Fenwick, “The Global Burden of Neglected Tropical Diseases,” Public Health, vol. 126, no. 3, pp. 233-236, 2012.
[CrossRef] [Google Scholar] [Publisher Link]
[13] K.M. Faizullah Fuhad et al., “Deep Learning Based Automatic Malaria Parasite Detection from Blood Smear and its Smartphone Based Application,” Diagnostics, vol. 10, no. 5, pp. 1-22, 2020.
[CrossRef] [Google Scholar] [Publisher Link]
[14] John A. Quinn et al., “Deep Convolutional Neural Networks for Microscopy-Based Point of Care Diagnostics,” Proceedings of the 1st Machine Learning for Healthcare Conference, vol. 56, pp. 271-281, 2016.
[Google Scholar] [Publisher Link]
[15] Anson Simon et al., “Shallow CNN with LSTM Layer for Tuberculosis Detection in Microscopic Image,” International Journal of Recent Technology and Engineering, vol. 7, no. 3, pp. 1-5, 2019.
[Google Scholar] [Publisher Link]
[16] Erdal Tasci, Caner Uluturk, and Aybars Ugur, “A Voting-Based Ensemble Deep Learning Method Focusing on Image Augmentation and Preprocessing Variations for Tuberculosis Detection,” Neural Computing and Applications, vol. 33, no. 22, pp. 15541-15555, 2021.
[CrossRef] [Google Scholar] [Publisher Link]
[17] Yaning Li et al., “A Low‐Cost, Automated Parasite Diagnostic System Via a Portable, Robotic Microscope and deep Learning,” Journal of Biophotonics, vol. 12, no. 9, 2019.
[CrossRef] [Google Scholar] [Publisher Link]
[18] Gargi Sharma, and Gourav Shrivastava, “Crop Disease Prediction Using Deep Learning Techniques - A Review,” SSRG International Journal of Computer Science and Engineering, vol. 9, no. 4, pp. 23-28, 2022.
[CrossRef] [Google Scholar] [Publisher Link]
[19] Carla Parra et al., “Automatic Identification of Intestinal Parasites in Reptiles Using Microscopic Stool Images and Convolutional Neural Networks,” Plos One, vol. 17, no. 8, pp. 1-24, 2022.
[CrossRef] [Google Scholar] [Publisher Link]
[20] Wei Liu et al., “SSD: Single Shot MultiBox Detector,” European Conference on Computer Vision, pp. 21-37, 2016.
[CrossRef] [Google Scholar] [Publisher Link]
[21] Joseph Redmon et al, “You Only Look Once: Unified, Real-Time Object Detection,” 2016 IEEE Conference on Computer Vision and Pattern Recognition (CVPR), pp. 779-788, 2016.
[CrossRef] [Google Scholar] [Publisher Link]
[22] Yanfen Li et al., “A deep Learning-Based Hybrid Framework for Object Detection and Recognition in Autonomous Driving,” IEEE Access, vol. 8, pp. 194228-194239, 2020.
[CrossRef] [Google Scholar] [Publisher Link]
[23] Alexey Bochkovskiy, Chien-Yao Wang, and Hong-Yuan Mark Liao, “YOLOv4: Optimal Speed and Accuracy of Object Detection,” arXiv, pp. 1-17, 2020.
[CrossRef] [Google Scholar] [Publisher Link]
[24] L. Kourkouta et al., “Treatment of Avian Influenza,” SSRG International Journal of Nursing and Health Science, vol. 5, no. 3, pp. 9-11, 2019.
[CrossRef] [Publisher Link]
[25] Ghaeth Safar, Mohamad Galiya, and Hassan Salman, “Gyrodactylus Worms of Monogenea Parasitizing on Common Carp Fish in Salah-ALdeen Dam Lake - Lattakia - Syria,” SSRG International Journal of Agriculture and Environmental Science, vol. 6, no. 5, pp. 41-43, 2019.
[CrossRef] [Google Scholar] [Publisher Link]