Ensemble Neuro Evolution of Augmenting Topologies Using Fused Features for Alzheimer’s Disease Diagnosis System
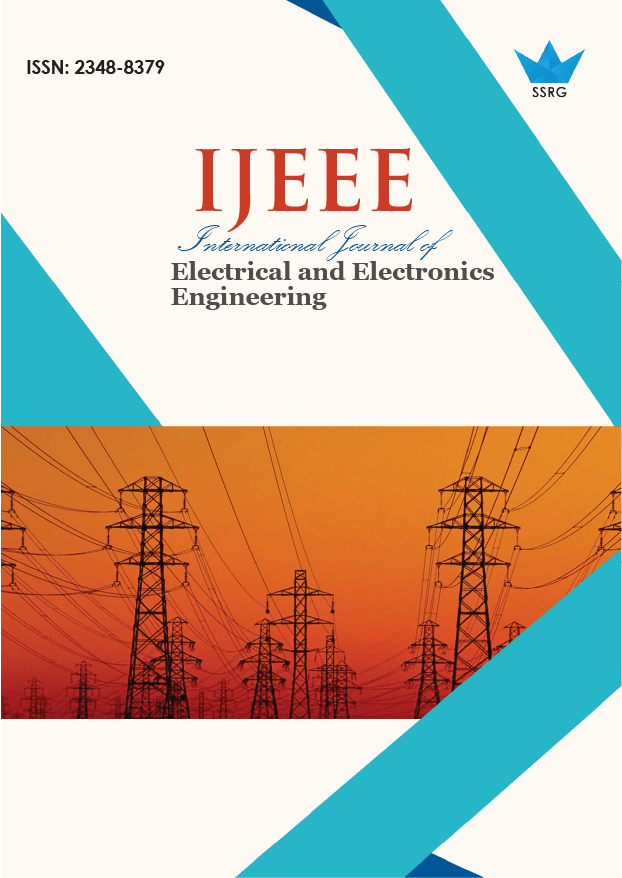
International Journal of Electrical and Electronics Engineering |
© 2023 by SSRG - IJEEE Journal |
Volume 10 Issue 10 |
Year of Publication : 2023 |
Authors : S.Chithra, R.Vijayabhanu |
How to Cite?
S.Chithra, R.Vijayabhanu, "Ensemble Neuro Evolution of Augmenting Topologies Using Fused Features for Alzheimer’s Disease Diagnosis System," SSRG International Journal of Electrical and Electronics Engineering, vol. 10, no. 10, pp. 20-31, 2023. Crossref, https://doi.org/10.14445/23488379/IJEEE-V10I10P103
Abstract:
Alzheimer’s Disease (AD) is a neurological condition that makes it difficult for a person to carry out the activities required of them daily. Because of the rapid advancement of Alzheimer’s patients and the lack of exact diagnostic techniques, early detection and classification of AD are essential research areas. One of the many researchers’ goals is to identify Alzheimer’s disease soon and correctly so it can be halted or delayed. Using a wide range of machine-learning algorithms, this study aims to compare the contemporary techniques for diagnosing and categorizing Alzheimer’s disease at the early stage. The proposed method effectively compares using the ADNI, which stands for the dataset, which is available to the public. Similarly, it reveals that the multi-feature combination methodology outperforms the single-feature extraction method. This paper proposes an AD diagnosis system that uses ML algorithms such as Support Vector Machine (SVM), Decision Tree (DT), K-Nearest Neighbour (KNN), Neuro Evolution of Augmenting Topologies (NEAT), and Bagging-NEAT (proposed) to diagnose AD in patients accurately. According to the study’s findings, the Bagging Neat can efficiently classify the stages of Alzheimer’s disease with an accuracy of 95.8% on the test dataset.
Keywords:
Alzheimer’s Disease, Features extraction, NEAT, Ensemble, Bagging, MR images.
References:
[1] Alzheimer’s Association, “2019 Alzheimer’s Disease Facts and Figures,” Alzheimer’s & Dementia, vol. 15, no. 3, pp. 321-387, 2019.
[CrossRef] [Google Scholar] [Publisher Link]
[2] Monika Sethi et al., “An Exploration: Alzheimer’s Disease Classification Based on Convolutional Neural Network,” BioMed Research International, vol. 2022, pp. 1-19, 2022.
[CrossRef] [Google Scholar] [Publisher Link]
[3] Monika Sethi et al., “A CAD System for Alzheimer’s Disease Classification Using Neuroimaging MRI 2D Slices,” Computational and Mathematical Methods in Medicine, vol. 2022, pp. 1- 11, 2022.
[CrossRef] [Google Scholar] [Publisher Link]
[4] Saidjalol Toshkhujaev et al., “Classification of Alzheimer’s Disease and Mild Cognitive Impairment Based on Cortical and Subcortical Features from MRI T1 Brain Images Utilizing Four Different Types of Datasets,” Journal of Healthcare Engineering, vol. 2020, pp. 1- 14, 2020.
[CrossRef] [Google Scholar] [Publisher Link]
[5] Jicha Gregory et al., “Screen and Intervene: The Importance of Early Detection and Treatment of Alzheimer’s Disease,” The Medical Roundtable General Medicine Edition, 2022.
[Google Scholar]
[6] M. Bachute et al., “Alzheimer’s Disease Detection and Classification Using Machine Learning Techniques,” 4th Smart Cities Symposium (SCS 2021), pp. 312-318, 2021.
[CrossRef] [Google Scholar] [Publisher Link]
[7] Kim Y.J. et al., “Predicting Alzheimer’s Disease Progression Using Machine Learning Methods,” Journal of Alzheimer’s Disease, vol. 74, no. 3, pp. 869-879, 2020.
[8] Subramanian N., Krishnamoorthy R., and Nagarajan V., “Early Detection of Alzheimer’s Disease: A Machine Learning Approach,” Journal of Medical Systems, vol. 46, no.2, pp. 1-9, 2022.
[9] Abdulhamit Subasi, “Use of Artificial Intelligence in Alzheimer’s Disease Detection,” Artificial Intelligence in Precision Health, pp. 257-278, 2020.
[CrossRef] [Google Scholar] [Publisher Link]
[10] Siddhartha Kumar Arjaria et al., “Performances of Machine Learning Models for Diagnosis of Alzheimer’s Disease,” Annals of Data Science, pp. 1-29, 2022.
[CrossRef] [Google Scholar] [Publisher Link]
[11] Khandaker Mohammad Mohi Uddin et al., “A Novel Approach Utilizing Machine Learning for the Early Diagnosis of Alzheimer’s Disease,” Biomedical Materials & Devices, pp. 1-17, 2023.
[CrossRef] [Google Scholar] [Publisher Link]
[12] Olfa Ben Ahmed et al., “Recognition of Alzheimer’s Disease and Mild Cognitive Impairment with Multimodal Image-Derived Biomarkers and Multiple Kernel Learning,” Neurocomputing, vol. 220, pp. 98-110, 2017.
[CrossRef] [Google Scholar] [Publisher Link]
[13] Heba Elshatoury et al., “Volumetric Histogram-Based Alzheimer’s Disease Detection Using Support Vector Machine,” Journal of Alzheimer’s Disease, vol. 72, no. 2, pp. 515-524, 2019.
[CrossRef] [Google Scholar] [Publisher Link]
[14] Uma Rani K. et al., “Binary Classification of Alzheimer’s Disease Using MRI Images and Support Vector Machine,” 2021 IEEE Mysore Sub Section International Conference (MysuruCon), India, pp. 423-426, 2021.
[CrossRef] [Google Scholar] [Publisher Link]
[15] Subramaniyan Mani et al., “An Analytical Framework for Screening Cardiogenic Brain Abscess in Patients with Tetralogy of Fallot,” International Journal of Engineering Trends and Technology, vol. 71, no. 2, pp. 78-88, 2023.
[CrossRef] [Publisher Link]
[16] Srinivasan Aruchamy, Veeramachaneni Mounya, and Ankit Verma, “Alzheimer’s Disease Classification in Brain MRI Using Modified KNN Algorithm,” 2020 IEEE International Symposium on Sustainable Energy, Signal Processing and Cyber Security (iSSSC), India, pp. 1-6, 2020.
[CrossRef] [Google Scholar] [Publisher Link]
[17] Anjani Yalamanchili, D. Venkatasekhar, and G. Vijay Kumar, “Attn_CNN_LSTM: IoT-Based Automated Alzheimer’s Disease Classification Using Deep Learning Approach,” International Journal of Engineering Trends and Technology, vol. 71, no. 7, pp. 132- 146, 2023.
[CrossRef] [Publisher Link]
[18] Payam Hosseinzadeh Kasani, “An Evaluation of Machine Learning Classifiers for Prediction of Alzheimer’s Disease, Mild Cognitive Impairment and Normal Cognition,” 2021 International Conference on Information and Communication Technology Convergence (ICTC), Jeju Island, Korea, pp. 362-367, 2021.
[CrossRef] [Google Scholar] [Publisher Link]
[19] Sebastian Lang et al., “Neuro Evolution of Augmenting Topologies for Solving A Two-Stage Hybrid Flow Shop Scheduling Problem: A Comparison of Different Solution Strategies,” Expert Systems with Applications, vol. 172, 2021.
[CrossRef] [Google Scholar] [Publisher Link]
[20] M.A. Ganaie, and M. Tanveer, “Ensemble Deep Random Vector Functional Link Network Using Privileged Information for Alzheimers Disease Diagnosis,” IEEE/ACM Transactions on Computational Biology and Bioinformatics, pp. 1-1, 2022.
[CrossRef] [Google Scholar] [Publisher Link]
[21] Donghwan Kim, and Jun-Geol Baek, “Bagging Ensemble-Based Novel Data Generation Method for Univariate Time Series Forecasting,” Expert Systems with Applications, vol. 203, 2022.
[CrossRef] [Google Scholar] [Publisher Link]
[22] A. Shah, A. Misra, and B.K. Panigrahi, “A Systematic Literature Review on Parameter Tuning of SVM Classifier,” Journal of King Saud University-Computer and Information Sciences, vol. 33, no. 2, pp. 134-150, 2021.
[23] R. Salehinejad et al., “Recent Advances in Decision Tree Algorithms,” IEEE Transactions on Knowledge and Data Engineering, vol. 33, no. 1, pp. 69-84, 2021.
[24] Gangsar Ali Daroni et al., “Therap Application to Evaluate Therapy Programs for Autistic Children,” SSRG International Journal of Medical Science, vol. 5, no. 7, pp. 18-21, 2018.
[CrossRef] [Google Scholar] [Publisher Link]
[25] N. Bruneel, A. Verstockt, and W. Philips, “An Empirical Comparison of Hyperparameter Tuning Techniques for k-Nearest Neighbour Classification,” Expert Systems with Applications, vol. 169, 2021.
[26] Y. Wang et al., “Improved Performance of NEAT-Based Neural Network in Solar Power Forecasting,” Renewable Energy, vol. 173, pp. 1048-1060, 2021.