Slime Mould-Based Collaborative Deep Boltzmann Machine for Intrusion Detection Model in Mobile Ad Hoc Network
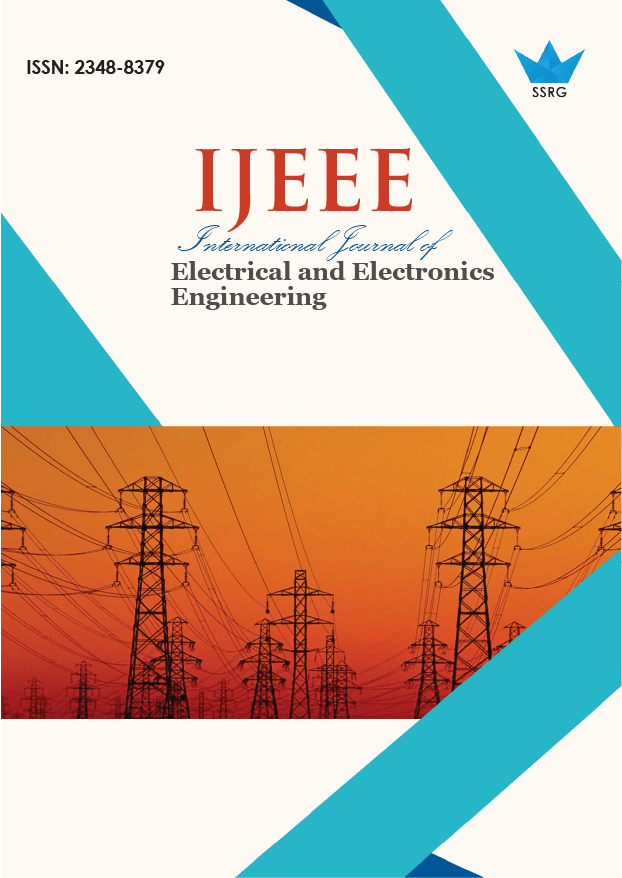
International Journal of Electrical and Electronics Engineering |
© 2023 by SSRG - IJEEE Journal |
Volume 10 Issue 11 |
Year of Publication : 2023 |
Authors : G. Parameshwar, N.V. Koteswara Rao, L. Nirmala Devi |
How to Cite?
G. Parameshwar, N.V. Koteswara Rao, L. Nirmala Devi, "Slime Mould-Based Collaborative Deep Boltzmann Machine for Intrusion Detection Model in Mobile Ad Hoc Network," SSRG International Journal of Electrical and Electronics Engineering, vol. 10, no. 11, pp. 31-38, 2023. Crossref, https://doi.org/10.14445/23488379/IJEEE-V10I11P103
Abstract:
Mobile Ad Hoc Network (MANET) is a dynamic wireless network developed by using wireless nodes without using any infrastructures. The significant features of MANET are low-cost infrastructure, self-organization, mobility and rapid deployment, which offer the opportunity to deploy it in various applications such as disaster relief, environmental monitoring and military communications. Based on the previous studies, improved Quality of Service (QoS) metrics with security problems during data communication are challenging with the increased wireless technology. By addressing these issues, here proposed a novel secured intrusion detection model in MANET. The cluster formation is effectuated with the Modified K-Harmonics Mean Clustering (MKHMC), and the cluster heads are selected with the proposed Chaotic Multi-verse Krill Herd Optimization (CMKHO) algorithm, which helps to provide energy efficiency, reduction in delay, and increased throughput. Meanwhile, this proposed blockchain-secured Slime Mould-based Collaborative Deep Boltzmann Machine (SM-CDBM) includes three stages, (i) learning the unimodal DBM models to identify the intrusion, (ii) learning the shared layer parameters utilizing a Collaborative Restricted Boltzmann Machine (CRBM), and (iii) fine-tuning the CDBM using the Slime Mould Optimization (SMO) algorithm. Simulations are effectuated in the NS2 tool and accomplish improved malicious node detection, end-to-end delay, energy efficiency, and overhead compared to other state-of-the-art approaches.
Keywords:
MANET, Modified K-Harmonics Mean Clustering, Chaotic Multi-verse Krill Herd Optimization, Collaborative DBM, Slime Mould Optimization algorithm.
References:
[1] Osamah Ibrahim Khalaf et al., “Efficient Dual-Cooperative Bait Detection Scheme for Collaborative Attackers on Mobile Ad-Hoc Networks,” IEEE Access, vol. 8, pp. 227962-227969, 2020.
[CrossRef] [Google Scholar] [Publisher Link]
[2] Vinicius Mariano Gonçalves, Ryan McLaughlin, and Guilherme A.S. Pereira, “Precise Landing of Autonomous Aerial Vehicles Using Vector Fields,” IEEE Robotics and Automation Letters, vol. 5, no. 3, pp. 4337-4344, 2020.
[CrossRef] [Google Scholar] [Publisher Link]
[3] Kamran Shaukat et al., “A Review on Security Challenges in Internet of Things (IoT),” 26th International Conference on Automation and Computing (ICAC), United Kingdom, pp. 1-6, 2021.
[CrossRef] [Google Scholar] [Publisher Link]
[4] Seichiroh Osaki, Takumi Ishihara, and Shinya Sugiura, “Eigenvalue-Decomposition-Precoded Ultra-Dense Non-Orthogonal Frequency-Division Multiplexing,” IEEE Transactions on Wireless Communications, vol. 19, no. 12, pp. 8165-8178, 2020.
[CrossRef] [Google Scholar] [Publisher Link]
[5] Erfan A. Shams, and Ahmet Rizaner, “A Novel Support Vector Machine Based Intrusion Detection System for Mobile Ad-Hoc Networks,” Wireless Networks, vol. 24, pp. 1821-1829, 2018.
[CrossRef] [Google Scholar] [Publisher Link]
[6] M. Islabudeen, and M.K. Kavitha Devi, “A Smart Approach for Intrusion Detection and Prevention System in Mobile Ad-Hoc Networks against Security Attacks,” Wireless Personal Communications, vol. 112, pp. 193-224, 2020.
[CrossRef] [Google Scholar] [Publisher Link]
[7] Zichen Zhang, Shifei Ding, and Yuting Sun, “A Support Vector Regression Model Hybridized with Chaotic Krill Herd Algorithm and Empirical Mode Decomposition for Regression Task,” Neurocomputing, vol. 410, pp. 185-201, 2020.
[CrossRef] [Google Scholar] [Publisher Link]
[8] Caciano Machado, and Carla Merkle Westphall, “Blockchain Incentivized Data Forwarding in MANETs: Strategies and Challenges,” Ad Hoc Networks, vol. 110, 2021.
[CrossRef] [Google Scholar] [Publisher Link]
[9] Mohammad Rafiqul Alam et al., “A Joint Deep Boltzmann Machine (jDBM) Model for Person Identification Using Mobile Phone Data,” IEEE Transactions on Multimedia, vol. 19, no. 2, pp. 317-326, 2017.
[CrossRef] [Google Scholar] [Publisher Link]
[10] Shimin Li et al., “Slime Mould Algorithm: A New Method for Stochastic Optimization,” Future Generation Computer Systems, vol. 111, pp. 300-323, 2020.
[CrossRef] [Google Scholar] [Publisher Link]
[11] K. Bala, S. Jothi, and A. Chandrasekar, “An Enhanced Intrusion Detection System for Mobile Ad-Hoc Network Based on Traffic Analysis,” Cluster Computing, vol. 22, pp. 15205-15212, 2019.
[CrossRef] [Google Scholar] [Publisher Link]
[12] R. Santhana Krishnan et al., “Modified Zone-Based Intrusion Detection System for Security Enhancement in Mobile Ad Hoc Networks,” Wireless Networks, vol. 26, pp. 1275-1289, 2020.
[CrossRef] [Google Scholar] [Publisher Link]
[13] R. Thiagarajan, M. Rajesh Babu, and M. Moorthi, “Quality of Service Based Ad Hoc On-Demand Multipath Distance Vector Routing Protocol in Mobile Ad Hoc Network,” Journal of Ambient Intelligence and Humanized Computing, vol. 12, pp. 4957-4965, 2021.
[CrossRef] [Google Scholar] [Publisher Link]
[14] Cong Pu, “Jamming-Resilient Multipath Routing Protocol for Flying Ad Hoc Networks,” IEEE Access, vol. 6, pp. 68472-68486, 2018.
[CrossRef] [Google Scholar] [Publisher Link]