WeaveSense: IoT Infrastructure for Rapier Loom Condition Monitoring and Analysis
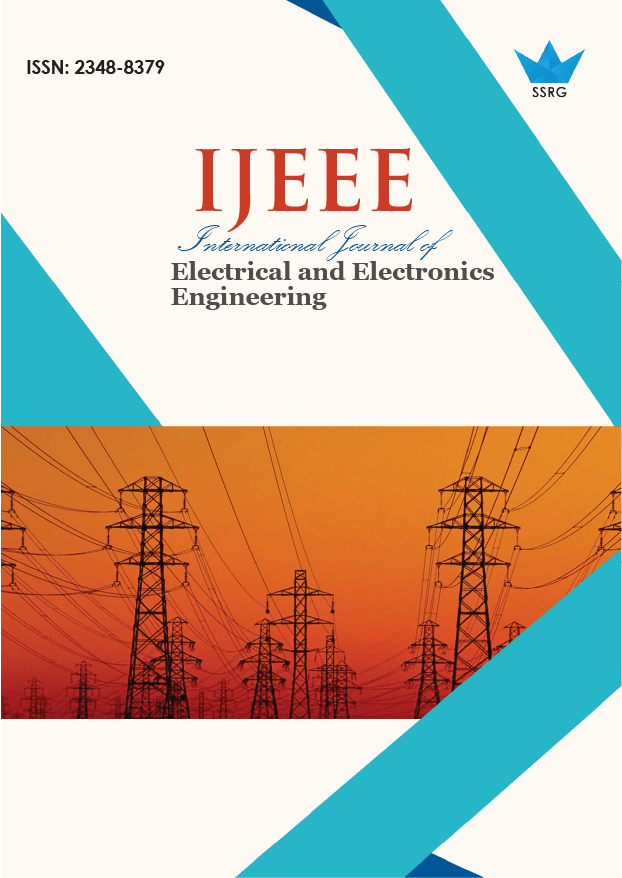
International Journal of Electrical and Electronics Engineering |
© 2023 by SSRG - IJEEE Journal |
Volume 10 Issue 12 |
Year of Publication : 2023 |
Authors : Ajit S. Gundale, Vaibhav M. Meshram |
How to Cite?
Ajit S. Gundale, Vaibhav M. Meshram, "WeaveSense: IoT Infrastructure for Rapier Loom Condition Monitoring and Analysis," SSRG International Journal of Electrical and Electronics Engineering, vol. 10, no. 12, pp. 27-36, 2023. Crossref, https://doi.org/10.14445/23488379/IJEEE-V10I12P104
Abstract:
Various machines and equipment are involved at different stages of the textile manufacturing process. The rapier looms are used to produce high-quality fabrics and other traditional textiles. The rapier looms are exposed to mechanical and frequently occurring electronic problems, which disrupts their operation and production efficiency. The condition monitoring of the rapier loom is helpful to ensure optimal performance. The IoT infrastructure for condition monitoring is designed and implemented at the industrial level, and ground truth data is captured. Weavesense targets temporal analysis of Service-Oriented Architecture (SOA) based on an IoT framework implemented for condition monitoringof rapier loom. The study of captured streaming data involves pre-processing, feature extraction and behaviour pattern recognition. The supervised machine learning approach permits correlating extracted features and captured data. The application scenario of the rapier loom and sequence of observations clearly show the consequences on the performance of the rapier loom.
Keywords:
Rapier loom, Augmented framework, Filtering, Feature extraction, Data validation, Supervised learning, Correlation.
References:
[1] Lilia Tightiz, and Hyosik Yang, “A Comprehensive Review on IoT Protocols’ Features in Smart Grid Communication,” Energies, vol. 13, no. 11, pp. 1-24, 2020.
[CrossRef] [Google Scholar] [Publisher Link]
[2] Rohan Pal et al., “A Comprehensive Review on IoTāBased Infrastructure for Smart Grid Applications,” IET Renewable Power Generation, vol. 15, no. 16, pp. 3761-3776, 2021.
[CrossRef] [Google Scholar] [Publisher Link]
[3] Rathin Chandra Shit et al., “Location of Things (LoT): A Review and Taxonomy of Sensors Localization in IoT Infrastructure,” IEEE Communications Surveys & Tutorials, vol. 20, no. 3, pp. 2028-2061, 2018.
[CrossRef] [Google Scholar] [Publisher Link]
[4] Shanzhi Chen et al., “A Vision of IoT: Applications, Challenges, and Opportunities with China Perspective,” IEEE Internet of Things Journal, vol. 1, no. 4, pp. 349-359, 2014.
[CrossRef] [Google Scholar] [Publisher Link]
[5] Tamas Ruppert, and Janos Abonyi, “Industrial Internet of Things Based Cycle Time Control of Assembly Lines,” 2018 IEEE International Conference on Future IoT Technologies (Future IoT), Eger, Hungary, pp. 1-4, 2018.
[CrossRef] [Google Scholar] [Publisher Link]
[6] Iqbal H. Sarker, “Machine Learning: Algorithms, Real-World Applications and Research Directions,” SN Computer Science, vol. 2, 2021.
[CrossRef] [Google Scholar] [Publisher Link]
[7] Osisanwo F.Y. et al., “Supervised Machine Learning Algorithms: Classification and Comparison,” International Journal of Computer Trends and Technology (IJCTT), vol. 48, no. 3, pp. 128-138, 2017.
[CrossRef] [Google Scholar] [Publisher Link]
[8] Deepanshu Jain, Sayam Kumar, and Yashika Goyal, “Fake Reviews Filtering System Using Supervised Machine Learning,” 2022 IEEE International Conference on Data Science and Information System (ICDSIS), Hassan, India, pp. 1-4, 2022.
[CrossRef] [Google Scholar] [Publisher Link]
[9] Gopal Chandra Jana, Anshuman Sabath, and Anupam Agrawal, “Performance Analysis of Supervised Machine Learning Algorithms for Epileptic Seizure Detection with High Variability EEG Datasets: A Comparative Study,” 2019 International Conference on Electrical, Electronics and Computer Engineering (UPCON), Aligarh, India, pp. 1-6, 2019.
[CrossRef] [Google Scholar] [Publisher Link]
[10] Somaieh Amraee, Maryam Chinipardaz, and Mohammadali Charoosaei, “Analytical Study of Two Feature Extraction Methods in Comparison with Deep Learning Methods for Classification of Small Metal Objects,” Visual Computing for Industry, Biomedicine, and Art, vol. 5, 2022.
[CrossRef] [Google Scholar] [Publisher Link]
[11] Erhui Xi, “Image Feature Extraction and Analysis Algorithm Based on Multi-Level Neural Network,” 2021 5th International Conference on Computing Methodologies and Communication (ICCMC), Erode, India, pp. 1062-1065, 2021.
[CrossRef] [Google Scholar] [Publisher Link]
[12] Yan Li et al., “A Comparative Study of Time Series Data Forecasting Using Machine Learning Based on Improved Grey Model,” 2021 IEEE 23rd International Conference on High Performance Computing & Communications; 7th International Conference on Data Science & Systems; 19th International Conference on Smart City; 7th International Conference on Dependability in Sensor, Cloud & Big Data Systems & Application (HPCC/DSS/SmartCity/DependSys), Haikou, Hainan, China, pp. 1643-1649, 2021.
[CrossRef] [Google Scholar] [Publisher Link]
[13] Ahmad Alqatawna et al., “Incorporating Time-Series Forecasting Techniques to Predict Logistics Companies’ Staffing Needs and Order Volume,” Computation, vol. 11, no. 7, pp. 1-17, 2023.
[CrossRef] [Google Scholar] [Publisher Link]
[14] Yusuke Takahashi, “Anomaly Detection Using Vibration Analysis with Machine Learning Technology for Industrial IoT System,” OKI Technical Review, vol. 84, no. 2, pp. 1-4, 2017.
[Google Scholar] [Publisher Link]
[15] Viju Chacko, and Vikram Bharati, “Data Validation and Sensor Life Prediction Layer on Cloud for IoT,” 2017 IEEE International Conference on Internet of Things (iThings) and IEEE Green Computing and Communications (GreenCom) and IEEE Cyber, Physical and Social Computing (CPSCom) and IEEE Smart Data (SmartData), Exeter, UK, pp. 906-909, 2017.
[CrossRef] [Google Scholar] [Publisher Link]
[16] Kukjin Choi et al., “Deep Learning for Anomaly Detection in Time-Series Data: Review, Analysis, and Guidelines,” IEEE Access, vol. 9, pp. 120043-120065, 2021.
[CrossRef] [Google Scholar] [Publisher Link]
[17] Manish Gupta et al., “Outlier Detection for Temporal Data: A Survey,” IEEE Transactions on Knowledge and Data Engineering, vol. 26, no. 9, pp. 2250-2267, 2014.
[CrossRef] [Google Scholar] [Publisher Link]
[18] Giuseppe Manco et al., “Fault Detection and Explanation through Big Data Analysis on Sensor Streams,” Expert Systems with Applications, vol. 87, pp. 141-156, 2017.
[CrossRef] [Google Scholar] [Publisher Link]
[19] Andrew A. Cook, Goksel Misirli, and Zhong Fan, “Anomaly Detection for IoT Timeseries Data: A Survey,” IEEE Internet of Things Journal, vol. 7, no. 7, pp. 6481-6494, 2020.
[CrossRef] [Google Scholar] [Publisher Link]
[20] Md Rafiqul Islam, Rafiqul Islam, and Abu Raihan M. Kamal, “Time Series Anomaly Detection in Online Social Network: Challenges & Solutions,” Proceedings of the 1st International Conference on Machine Learning and Data Engineering (iCMLDE2017), pp. 21-28, 2017.
[Google Scholar] [Publisher Link]
[21] Weiling Liu et al., “A Condition Monitoring Strategy of Looms Based on DSmT Theory and Genetic Multiobjective Optimization Improves the Rough Set Method,” IEEE Access, vol. 10, pp. 59723-59736, 2022.
[CrossRef] [Google Scholar] [Publisher Link]
[22] Reshma Ajith et al., “Low-Cost Vibration Sensor for Condition-Based Monitoring Manufactured from Polyurethane Foam,” IEEE Sensors Letters, vol. 1, no. 6, pp. 1-4, 2017.
[CrossRef] [Google Scholar] [Publisher Link]
[23] Alan Rezazadeh, “Wind Turbine Gearbox Condition Based Monitoring,” arXiv, pp. 1-14, 2021.
[CrossRef] [Google Scholar] [Publisher Link]