A Comparison of Methods for Spatial Interpolation across Different Spatial Scales
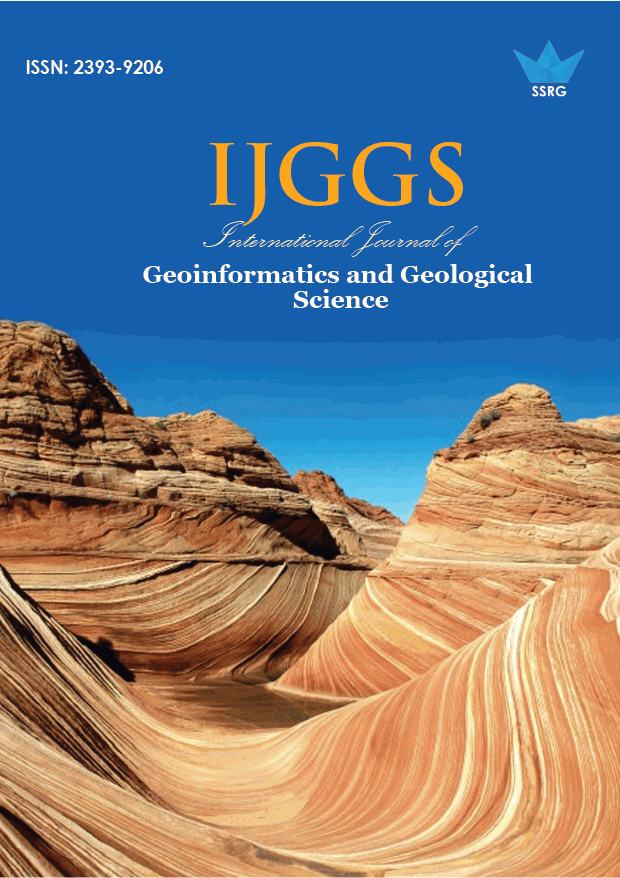
International Journal of Geoinformatics and Geological Science |
© 2017 by SSRG - IJGGS Journal |
Volume 4 Issue 2 |
Year of Publication : 2017 |
Authors : Idris Mohammed Jega, Alexis J. Comber, Nicholas J. Tate |
How to Cite?
Idris Mohammed Jega, Alexis J. Comber, Nicholas J. Tate, "A Comparison of Methods for Spatial Interpolation across Different Spatial Scales," SSRG International Journal of Geoinformatics and Geological Science, vol. 4, no. 2, pp. 12-22, 2017. Crossref, https://doi.org/10.14445/23939206/IJGGS-V4I3P102
Abstract:
Spatially distributed estimates of population provide commonly used demand surfaces in support of spatial planning. In many countries, spatially detailed population estimates in small areas are not available. For such cases a number of interpolation methods have been proposed to redistribute summary population totals over small areas to estimate locally nuanced demand surfaces. Population allocations to small areas are commonly validated by comparing the estimates with some known values for those areas. This paper explores different interpolation methods applied at different spatial scales in locations where the validation of estimated surfaces is possible in order to suggest appropriate interpolation parameters for locations where it is not. The results show binary dasymetric mapping applied at medium scales provide the best estimates of population, among the methods, areal weighting the worst at all scales and pycnophylactic interpolation shows significant improvement on areal weighting at all scales. This paper provides a comprehensive evaluation of these techniques, using different scales of input data and residual mappings to compare and evaluate the spatial distribution of errors in the estimated surfaces. The application of such methods for estimating spatially distributed demand population values in different types of spatial data analysis and in locations where validation data do not exist are discussed.
Keywords:
Areal interpolation, dasymetric mapping, areal weighting, pycnophylactic interpolation, population data.
References:
[1]. Langford, M. An Evaluation of Small Area Population Estimation Techniques Using Open Access Ancillary Data. Geographical Analysis 2013, 45, 324-344.
[2]. Harris, R. J.; Longley, P. A. Creating small area measures of urban deprivation. Environment and Planning A 2002, 34, 1073-1093.
[3]. Martin, D. From enumeration districts to output areas: Experiments in the automated creation of a census output geography. Population Trends 1997, 88, 36-42.
[4]. Martin, D. Optimizing census geography: The separation of collection and output geographies. International Journal of Geographical Information Science 1998, 12, 673-685.
[5]. Leyk, S.; Nagle, N. N.; Buttenfield, B. P. Maximum Entropy Dasymetric Modeling for Demographic Small Area Estimation. Geographical Analysis 2013, 45, 285-306.
[6]. Goodchild, M. F.; Lam, N. Areal interpolation: A variant of the traditional spatial problem. Geo-Processing 1980, 1, 297- 312.
[7]. Zandbergen, P. A.; Ignizio, D. A. Comparison of Dasymetric Mapping Techniques for Small-Area Population Estimates. Cartography and Geographic Information Science 2010, 37, 199-214.
[8]. Wu, S.; Qiu, X.; Wang, L. Population estimation methods in GIS and remote sensing: a review. Geographic Information Science and Remote Sensing 2005, 42, 80-96.
[9]. Lam, N. S. Spatial Interpolation Methods: A Review. The American Cartographer 1983, 10, 129-149.
[10]. Langford, M.; Higgs, G. Measuring Potential Access to Primary Healthcare Services: The Influence of Alternative Spatial Representations of Population. The Professional Geographer 2006, 58, 294-306.
[11]. Hewko, J.; Smoyer-Tomic, K. E.; Hodgson, M. J. Measuring neighbourhood spatial accessibility to urban amenities: does aggregation error matter? Environment and Planning A 2002, 34, 1185-1206.
[12]. Tobler, W. Smooth Pycnophylactic Interpolation for Geographical Regions. Journal of the American Statistical Association 1979, 74, 519-530.
[13]. Langford, M.; Maguire, D. J.; Unwin, D. J. The area transform problem: Estimating population using remote sensing in a GIS framework. In: Masser, I. and Blakemore, M. (eds) Handling Geographical Information: Methodology and Potential Applications. London: Longman 1991, 55-77.
[14]. Eicher, C.; Brewer, C. Dasymetric mapping and areal interpolation: Implementation and evaluation. Cartography and Geographic Information Science 2001, 28, 125-138.
[15]. Mennis, J. Generating surface models of population using dasymetric mapping. The Professional Geographer 2003, 55, 31-42.
[16]. Green, N. E. Aerial photographic analysis of residential neighbourhoods’: An evaluation of data accuracy. Social Forces 1956, 35, 142-147.
[17]. Lo, C. P. Population Estimation Using Geographically Weighted Regression. GIScience and Remote Sensing 2008, 45, 131-148.
[18]. Lillesand, T. M.; Kiefer, R. W., Eds.; In Remote sensing and image interpretation; John Wiley and Sons.: New York, 1987. [19]. Lo, C. P. Estimating Population and Census Data. American Society for Photogrammetry and Remote Sensing 2006, 337- 377.
[20]. Langford, M.; Unwin, D. Generating and mapping population density surfaces within a geographical information system. The Cartographic Journal 1994, 31, 21- 26.
[21]. Cromley, R. G.; Hanink, D. M.; Bentley, G. C. A Quantile Regression Approach to Areal Interpolation. Annals of the Association of American Geographers 2011, 102, 763-777.
[22]. Mennis, J. Dasymetric Mapping for Estimating Population in Small Areas. Geography Compass 2009, 3, 727-745.
[23]. Tapp, A. F. Areal Interpolation and Dasymetric Mapping Methods Using Local Ancillary Data Sources. Cartography and Geographic Information Science 2010, 37, 215-228.
[24]. Su, M.; Lin, M.; Hsieh, H.; Tsai, B.; Lin, C. Multi-Layer Multi-class Dasymetric Mapping to Estimate Population Distribution. Science of the Total Environment 2010, 408, 4807-4816.
[25]. Langford, M. Obtaining population estimates in non-census reporting zones: An evaluation of the 3-class dasymetric method. Computers, Environment and Urban Systems 2006, 30, 161-180.
[26]. Qiu, F.; Cromley, R. Areal Interpolation and Dasymetric Modeling. Geographical Analysis 2013, 45, 213-215.
[27]. Sridharan, H.; Qiu, F. A Spatially Disaggregated Areal Interpolation Model Using Light Detection and Ranging- Derived Building Volumes. Geographical Analysis 2013, 45, 238-258.
[28]. Qiu, F.; Zhang, C.; Zhou, Y. The Development of an Areal Interpolation ArcGIS Extension and a Comparative Study. GIScience and Remote Sensing 2012, 49, 644-663.
[29]. Mennis, J.; Hultgren, T. Intelligent dasymetric mapping and its application to areal interpolation. Cartography and Geographic Information Science 2006, 3 3, 179-194.
[30]. Lu, D.; Li, G.; Moran, E.; Freitas, C. C.; Dutra, L.; Anna, S. J. S. In In A Comparison of Maximum Likelihood Classifier and Object-Based Method Based on Multiple Sensor Datasets for Land-Use/Cover Classification in the Brazilian Amazon; Proceedings of the 4th GEOBIA; Rio de Janero, Brazil, 2012; pp 20.
[31]. Blaschke, T. Object based image analysis for remote sensing. ISPRS Journal of Photogrammetry and Remote Sensing 2010, 65, 2-16.
[32]. Zhou, W.; Huang, G.; Troy, A.; Cadenasso, M. L. Objectbased land cover classification of shaded areas in high spatial resolution imagery of urban areas: A comparison study. Remote Sensing of Environment 2009, 113, 1769-1777.
[33]. Lu, D.; Hetrick, S.; Moran, E. Land cover classification in a complex urban-rural landscape with QuickBird imagery. Photogrammetric Engineering and Remote Sensing 2010, 76, 1159-1168.
[34]. Comber, A.; Proctor, C.; Anthony, S. The Creation of a National Agricultural Land Use Dataset: Combining Pycnophylactic Interpolation with Dasymetric mapping technique. Transactions in GIS 2008, 12, 775-791.