Monitoring and Prediction of Boiler Refractory Failure by Remote Refractory Failure Detection and Temperature Pressure Monitoring System an Artificial Intelligence based System Using Machine Learning
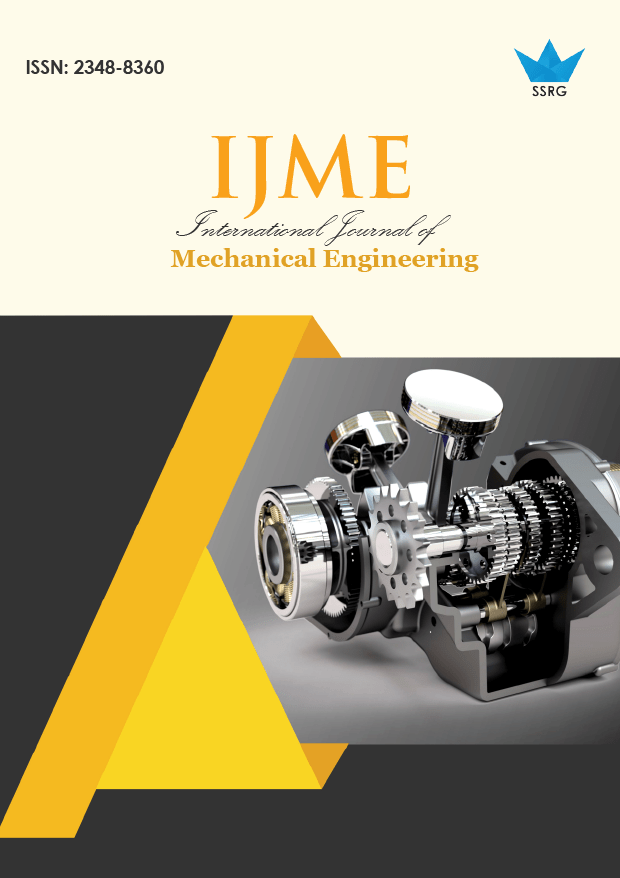
International Journal of Mechanical Engineering |
© 2025 by SSRG - IJME Journal |
Volume 12 Issue 4 |
Year of Publication : 2025 |
Authors : Meeta P Chauhan, Jeetendra A Vadher |
How to Cite?
Meeta P Chauhan, Jeetendra A Vadher, "Monitoring and Prediction of Boiler Refractory Failure by Remote Refractory Failure Detection and Temperature Pressure Monitoring System an Artificial Intelligence based System Using Machine Learning," SSRG International Journal of Mechanical Engineering, vol. 12, no. 4, pp. 61-69, 2025. Crossref, https://doi.org/10.14445/23488360/IJME-V12I4P106
Abstract:
Energy management is a crucial issue nowadays because the global energy demand is increasing day by day, and there are limited resources of energy available worldwide. The study utilized Internet of Things (IoT) devices to investigate cracks and hot spots of failed structures to understand and address the problem. Refractory materials constitute an integral element in industries involving high-temperature applications, such as furnaces, kilns, and reactors. Mechanical failure of these refractories in the said applications could result in large-scale disasters, including personnel hazards, costly downtime, and economic inefficiency in operations. This work constitutes research into the integration of various techniques in IoT applied to the early detection of refractory material failure. We have made the Smart system, which helps small-scale industries to identify energy loss and early detection of any fault in refractory. The system is based on the concept of artificial intelligence machine learning. It detects any fault in the boiler furnace, and we can also identify any significant heat/energy loss in the furnace. We used an artificial intelligence-based supervised machine learning algorithm and Python program to detect defects in refractory materials. The supervised data was collected over 21 months from the Thermax boiler furnace. This expert system will also reduce the inspection of periodic and frequent boiler plant shutdowns due to periodic checking and inspections. This smart system also avoids major boiler-exploding incidents and accidents in small-scale industrial plants.
Keywords:
Industry Innovation & Infrastructure, Energy loss, Refractory failure, Artificial intelligence based supervised Machine Learning, Energy management using the Internet of Things.
References:
[1] Refractories Handbook, The Technical Association of Refractories, Tokyo, Japan, 1998.
[Google Scholar]
[2] David O. Patrick et al., “Investigation of the Physical Properties of Tiles Produced with Otukpo Clay,” Leonardo Electronic Journal of Practices and Technologies, vol. 14, no. 27, pp. 162-176, 2015.
[Google Scholar] [Publisher Link]
[3] R.A. Heindl, and L. E. Mong, “Young's Modulus of Elasticity, Strength, and Extensibility of Refractories in Tension,” National Bureau of Standards, Report, vol. 17, pp. 463-482, 1936.
[Google Scholar] [Publisher Link]
[4] Eso Oluwasegun Falodun et al., “Investigating the Effects of High Alumina Cement and Silica Sand on the Suitability of Ikere Ekiti Clay for Refractory Applications,” American Journal of Engineering Research, vol. 6, no. 6, pp. 24-28, 2017.
[Google Scholar] [Publisher Link]
[5] Pritibhushan Sinha, and Subhash Saha Chandra, “An Optimum Design of the Lining of a Medium Frequency Induction Melting Furnace,” International Transactions in Operational Research, vol. 5, no. 4, pp. 255-259, 1998.
[CrossRef] [Google Scholar] [Publisher Link]
[6] Charles Schacht, Refractory Linings: ThermoMechanical Design and Applications, 1st ed., CRC Press, pp. 1-504, 1995.
[CrossRef] [Google Scholar] [Publisher Link]
[7] Fabien Simonin et al., “Thermomechanical Behavior of High-Alumina Refractory Castables with Synthetic Spinel Additions,” Journal of the American Ceramic Society, vol. 83, no. 10, pp. 2481-2490, 2000.
[CrossRef] [Google Scholar] [Publisher Link]
[8] E. Blond et al., “Modelling of High Temperature Asymmetric Creep Behavior of Ceramics,” Journal of the European Ceramic Society, vol. 25, no. 11, pp. 1819-1827, 2005.
[CrossRef] [Google Scholar] [Publisher Link]
[9] F. Damhof, W.A.M. Brekelmans, and M.G.D. Geers, “Experimental Analysis of the Evolution of Thermal Shock Damage Using Transit Time Measurement of Ultrasonic Waves,” Journal of the European Ceramic Society, vol. 29, no. 8, pp. 1309-1322, 2009.
[CrossRef] [Google Scholar] [Publisher Link]
[10] F. Damhof, W.A.M. Brekelmans, and M.G.D. Geers, “Non-Local Modeling of Thermal Shock Damage in Refractory Materials,” Engineering Fracture Mechanics, vol. 75, no. 16, pp. 4706-4720, 2008.
[CrossRef] [Google Scholar] [Publisher Link]
[11] Meeta P Chauhan et al., “Micro Analysis On Energy Management In Context of SME Industries: A Review of Present Practices, Gaps and Needs And Scope of Implementation of RRFDTPM System” Science, Technology and Development, vol. XI, no. VII, pp. 394 398, 2022.
[Google Scholar] [Publisher Link]
[12] Syafiqah Jamil et al., “Machine Learning Price Prediction on Green Building Prices,” 2020 IEEE Symposium on Industrial Electronics & Applications, TBD, Malaysia, pp. 1-6, 2020.
[CrossRef] [Google Scholar] [Publisher Link]
[13] Meeta P. Chauhan, and Jeetendra A. Vadher, “A Step Towards a New Horizon of Industry 4.0 with an Artificial Intelligent Refractory Failure Detection System Using IoT Technology,” Journal of Emerging Technologies and Innovative Research, vol. 9, no. 8, pp. e304 e307, 2022.
[Publisher Link]
[14] Nor Hamizah Zulkifley et al., “House Price Prediction Using a Machine Learning Model: A Survey of Literature,” International Journal of Modern Education and Computer Science, vol. 12, no. 6, pp. 46-54, 2020.
[CrossRef] [Google Scholar] [Publisher Link]
[15] Nehal N Ghosalkar, and Sudhir N Dhage, “Real Estate Value Prediction Using Linear Regression,” Fourth International Conference on Computing Communication Control and Automation, Pune, India, pp. 1-5, 2018.
[CrossRef] [Google Scholar] [Publisher Link]
[16] K.C. Bala, “Design Analysis of an Electric Induction Furnace for Melting Aluminum Scrap,” AU Journal of Technology, vol. 9, no. 2, pp. 83-88, 2005.
[Google Scholar]
[17] Bianca Schroeder, and G. A. Gibson, “The Computer Failure Data Repository (CFDR): Collecting, Sharing and Analyzing Failure Data,” Proceedings of the 2006 ACM/IEEE conference on Supercomputing, Tampa Florida, 2006.
[CrossRef] [Google Scholar] [Publisher Link]
[18] Angela Iagăr, Gabriel Nicolae Popa, and Ioan Šora, “Analysis of Electromagnetic Pollution Produced by Line Frequency Coreless Induction Furnaces,” Wseas Transactions on Systems, vol. 8, no. 1, pp. 1-11, 2009.
[Google Scholar] [Publisher Link]
[19] Nihar Bhagat, Ankit Mohorkar, and Shreyas Mane, “House Price Forecasting Using Data Mining,” International Journal of Computer Applications, vol. 152, no. 2, pp. 23-26, 2016.
[CrossRef] [Google Scholar] [Publisher Link]
[20] Bianca Schroeder, and Garth A. Gibson, “A Large-Scale Study of Failures in High-Performance Computing Systems,” IEEE Transactions on Dependable and Secure Computing, vol. 7, no. 4, pp. 337-350, 2010.
[CrossRef] [Google Scholar] [Publisher Link]
[21] V.N. Vapnik, “An Overview of Statistical Learning Theory,” IEEE Transactions on Neural Networks, vol. 10, no. 5, pp. 988-999, 1999.
[CrossRef] [Google Scholar] [Publisher Link]
[22] Marcelo C Medeiros, Alvaro Veiga, and Mauricio G.C. Resende, “A Combinatorial Approach to Piecewise Linear Time Series Analysis,” Journal of Computational and Graphical Statistics, vol. 11, no. 1, pp. 236-258, 2002.
[CrossRef] [Google Scholar] [Publisher Link]
[23] S. Avdakovic, A. Ademovic, and A. Nuhanovic, “Correlation between Air Temperature and Electricity Demand by Linear Regression and Wavelet Coherence Approach: UK, Slovakia and Bosnia and Herzegovina Case Study,” Archives of Electrical Engineering, vol. 62, no. 4, pp. 521-532, 2013.
[CrossRef] [Google Scholar] [Publisher Link]
[24] S.D. Alaruri, and M.F. Amer, “Empirical Regression Models for Weather Data Measured in Kuwait during the Years 1985, 1986, and 1987,” Solar Energy, vol. 50, no. 3, pp. 229-233, 1993.
[CrossRef] [Google Scholar] [Publisher Link]
[25] Robert F. Chevalier, “Air Temperature Prediction Using Support Vector Regression and GENIE: The Georgia Extreme-Weather Neural-Network Informed Expert,” Thesis, University of Georgia, pp. 1-72, 2008.
[Google Scholar] [Publisher Link]
[26] Shanshan Duan et al., “Grain Pile Temperature Forecasting from Weather Factors: A Support Vector Regression Approach,” 2019 IEEE/CIC International Conference on Communications in China, Changchun, China, pp. 255-260, 2019.
[CrossRef] [Google Scholar] [Publisher Link]
[27] Luca Massaron, and Alberto Boschetti, Regression Analysis with Python, Packt Publishing, pp. 1-312, 2016.
[Google Scholar] [Publisher Link]
[28] Ashfaq Ali Shafin et al., “Machine Learning Approach to Forecast Average Weather Temperature of Bangladesh,” Global Journal of Computer Science and Technology, vol. 19, no. 3, pp. 39-48, 2019.
[Google Scholar]
[29] Antanas Verikas et al., “Electromyographic Patterns during Golf Swing: Activation Sequence Profiling and Prediction of Shot Effectiveness,” Sensors, vol. 16, no. 4, pp. 1-26, 2016.
[CrossRef] [Google Scholar] [Publisher Link]
[30] Woosik Lee et al., “Machine Learning Based Prediction of the Value of Buildings,” Korean Society for Internet Information, vol. 12, no. 8, pp. 3966-3991, 2018.
[CrossRef] [Google Scholar] [Publisher Link]
[31] Chirag Modi et al., “A Survey on Security Issues and Solutions at Different Layers of Cloud Computing,” The Journal of Supercomputing, vol. 63, pp. 561-592, 2013.
[CrossRef] [Google Scholar] [Publisher Link]
[32] A. Abu-Samah et al., “Failure Prediction Methodology for Improved Proactive Maintenance Using Bayesian Approach,” IFAC PapersOnLine, vol. 48, no. 21, pp. 844-851, 2015.
[CrossRef] [Google Scholar] [Publisher Link]
[33] Donghwoon Kwon et al., “A Survey of Deep Learning-Based Network Anomaly Detection,” Cluster Computing, vol. 22, pp. 949-961, 2019.
[CrossRef] [Google Scholar] [Publisher Link]
[34] Mohammed Abo-Zahhad et al., “Design and Implementation of Building Energy Monitoring and Management System Based on Wireless Sensor Networks,” 2015 Tenth International Conference on Computer Engineering & Systems, Cairo, Egypt, pp. 230-233, 2015.
[CrossRef] [Google Scholar] [Publisher Link]
[35] James Manyika et al., Unlocking the Potential of the Internet of Things, Mckinsey Digital. [Online]. Available: https://www.mckinsey.com/business-functions/digital-mckinsey/ourinsights/the-internet-of-things-the-value-of-digitizing-the physical-world