Spatial Frailty Survival Model for Infection of Tuberculosis among HIV Infected Individuals
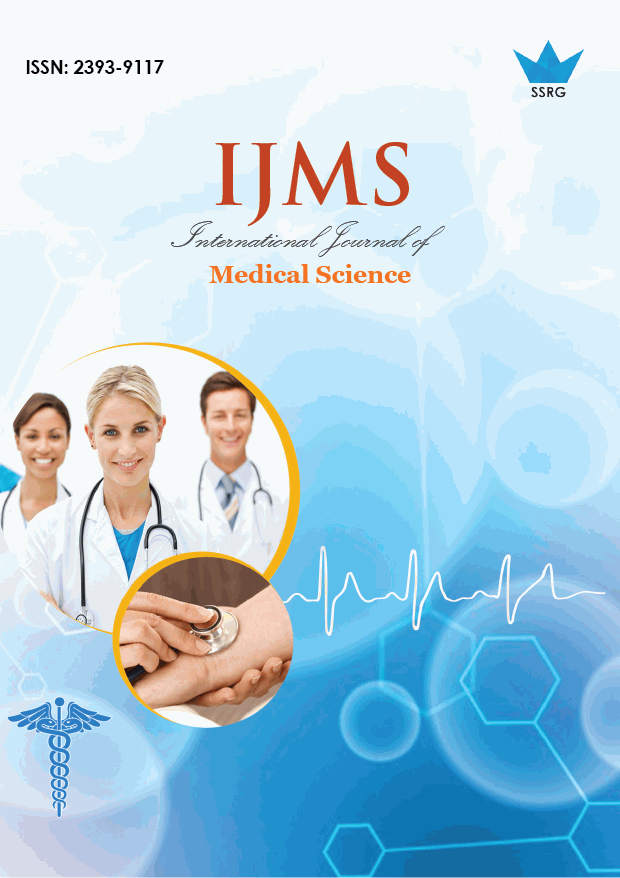
International Journal of Medical Science |
© 2017 by SSRG - IJMS Journal |
Volume 4 Issue 2 |
Year of Publication : 2017 |
Authors : Srinivasan R, Ponnuraja C, Rajendran S and Venkatesan P |
How to Cite?
Srinivasan R, Ponnuraja C, Rajendran S and Venkatesan P, "Spatial Frailty Survival Model for Infection of Tuberculosis among HIV Infected Individuals," SSRG International Journal of Medical Science, vol. 4, no. 2, pp. 5-10, 2017. Crossref, https://doi.org/10.14445/23939117/IJMS-V4I2P102
Abstract:
Capturing spatial variation is important while studying disease survival in different geographical regions where regions has its own risk factor associated with disease. To account this risk factor, frailty effect was introduced in this model that captures correlation and variation between neighboring locations using conditionally autoregressive (CAR) prior in Bayesian parametric survival model for studying dual infection of tuberculosis and HIV. National Institute for Research in Tuberculosis data on tuberculosis and HIV were used in this study. Monte Carlo Markov Chain (MCMC) technique was used to estimate the parameter. WinBUGS software was used for Bayesian Survival model estimation. The result of the study revealed that the spatial frailty model accounts higher heterogeneity along with weight at baseline was one of the significant factors associated with death and conclude that there were unmeasured covariates and risk factors influencing death in the Chennai regions.
Keywords:
Bayesian spatial, Survival, CAR, Weibull, random effects.
References:
[1] Banerjee S, Carlin B and Gelfand A E (2004): Hierarchical Modeling and Analysis for Spatial Data. Boca Raton: Chapman & Hall.
[2] Banerjee S, Wall M M and Carlin B P (2003): Frailty modeling for spatially-correlated survival data with application to infant mortality in Minnesota. Biostatistics, 4: 123-142.
[3] Besag J (1974): Spatial interaction and the statistical analysis of lattice systems. Journal of Royal Statistical Society, Series-B, 36: 192-236.
[4] Besag J and Kooperberg C (1995): On conditional and intrinsic autoregressions. Biometrika, 82: 733-746.
[5] Besag J, York J C and Mollie A (1991): Bayesian image restoration, with two applications in spatial statistics. Annals of the Institute of Statistical Mathematics, 43: 1-59.
[6] Carlin B P and Banerjee S (2003): Hierarchical multivariate CAR models for spatio-temporally correlated survival data, Bayesian Statistics 7. Oxford: Oxford University Press.
[7] Carlin B P and Hodges J S (1999): Hierarchical proportional hazards regression models for highly stratified data. Biometrics, 55: 1162-1170.
[8] Cox D and Oakes D (1984): Analysis of Survival Data. London: Chapman and Hall.
[9] Gelfand A Diggle P and Guttorp P (2010): Handbook of spatial statistics, Chapman & Hall / CRC.
[10] GeoBUGS User Manual (2004): GeoBUGS User Manual. Version 1.2.
[11] Lawson A, Browne W and Vidal Rodeiro C (2003): Disease mapping with WinBUGS and MLwiN. John Wiley & Sons Ltd.
[12] Li Y and Ryan L (2002): Modeling spatial survival data using semiparametric frailty models. Biometrics, 58, 287-297.
[13] Spiegelhalter D, Thomas A, Best N and Lunn D (2002b): WinBugs User Manual Version 1.4. Cambridge, England: MRC Biostatistics Unit.
[14] Venkatesan P and Srinivasan R (2008): Bayesian model of HIV/AIDS in India: A spatial Analysis. Applied Bayesian Statistical Analysis : 51-56.
[15] Venkatesan P and Srinivasan R (2011): Bayesian intrinsic conditional autoregressive random effect model of tuberculosis : An application, Recent trends in Statistics and computer application, Journal from Manonmanian Sundaranar University : 195-200.
[16] Venkatesan P, Srinivasan R and Dharuman C (2012): Bayesian conditional autoregressive model for mapping tuberculosis prevalence in India, International Journal of Pharmaceutical Studies and Research, Vol.III, online journal.
[17] Xia H and Carlin B (2005): Multivariate parametric spatiotemporal models for country level breast cancer survival data, Life time Data analysis, 11: 107–120.