Application of Artificial Neural Network Model In Forecasting Water Demand: Case of Kimilili Water Supply Scheme, Kenya.
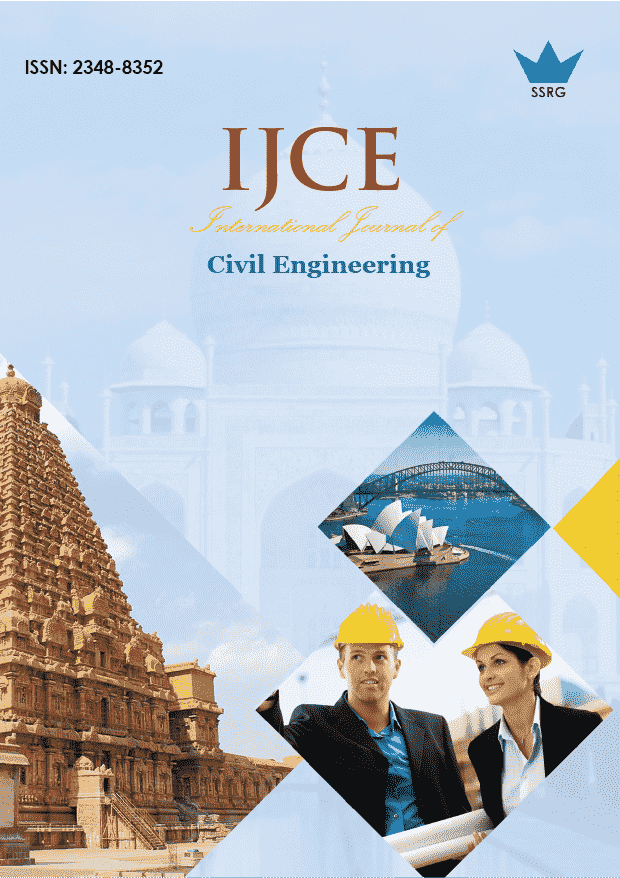
International Journal of Civil Engineering |
© 2019 by SSRG - IJCE Journal |
Volume 6 Issue 9 |
Year of Publication : 2019 |
Authors : Celsus Murenjekha Shilehwa, Sibilike Khamala Makhanu, Alex Wabwoba Khaemba |
How to Cite?
Celsus Murenjekha Shilehwa, Sibilike Khamala Makhanu, Alex Wabwoba Khaemba, "Application of Artificial Neural Network Model In Forecasting Water Demand: Case of Kimilili Water Supply Scheme, Kenya.," SSRG International Journal of Civil Engineering, vol. 6, no. 9, pp. 6-11, 2019. Crossref, https://doi.org/10.14445/23488352/IJCE-V6I9P102
Abstract:
Potable water treatment and supply systems are designed and constructed to deliver adequate water to meet consumer demand requirements. Consequently, water demand forecasting is essential for the design and operations management of treated water supply systems. Correct prediction of time-varying water demand trends and the critical water demand values determines the extent to which a network can satisfy critical demand and maintain economic efficiency. This study aimed to forecast Kimilili water supply scheme water demand up to 2030. Kimilili water supply scheme being operated by Nzoia Water Services Company Limited is characterized by rapidly increasing water demand leading to persistent water supply shortages hence unplanned fluctuations in the system water production hours. The artificial Neural Network (ANN) model was utilized to forecast Kimilili water supply scheme water demand. The trained model had good performance with a coefficient of determination (R2) of 0.999972988. The results indicated that Water demand for the Kimilili water supply increased with time, and the general relationship between time and water demand was defined by a sixth-order polynomial function given by y = 9e-0x6-1e-05x5+0.0005x4-0.0115x3+0.1178x2+0.1384x+100.48. The study confirmed that ANN could simulate the water demand characteristics of the water supply very well.
Keywords:
Water demand, Artificial neural network, Black box, Sociologic variables, Economic variables.
References:
[1] K. M. Anil, Plan for Augmentation of Capacities for Water Supply System in GIS. The thesis of Bachelor of Planning, Jawaharlal Nehru Technological University, Hyderabad, (2004).
[2] W. M. Patrick, Water Demand Determinants and Forecasting For Nzoia Cluster Services Area. (2016).
[3] T. T. Tanyimboh, M.Tabesh, & H. Surendran, Peaking demand factor-based reliability analysis of water distribution systems, Advances in Engineering Software,36,(2005),789-796,
[4] A. Kame'enui, Water Demand Forecasting in the Puget Sound Region: Short and Long term Models. The University of Washington,(2003).
[5] S. Rahman and R. Bhatnagar, Expert System Based Algorithm for Short-term Load Forecast, Journal of IEEE Trans Power Systems 3(2),392–399,(1988).
[6] J. A. Hartley and R. S Powell, The Development of a Combined Water Demand Prediction System, Civil Engin. Syst. 8,(1991),231–236.
[7] H. Caswell, The validation problem. Systems Analysis and Simulation in Ecology. Vol. IV. B. Patten, ed. New York: Academic Press, (1976).
[8] E. J. Rykiel, Testing Ecological Models. The Meaning of Validation, Ecological Modeling, 90:229-244, (1996).
[9] Nzoia Water Services Company Limited, NZOWASCO: 2014/2015 Annual Report, (2015).
[10] H. R. Douglas, Applied Statistics for Engineers. Academic Press, New York,(2003).
[11] Ankit Kumar Nigam, Prof. D.C Rahi, Analysis of Water Demand and Forecasting Water Demand for Year 2048 Jabalpur City, SSRG International Journal of Civil Engineering 3(7) (2016) 37-42.